Unsupervised Low-light Image Enhancement with Plug-and-play Prior Modeling
2023 International Conference on the Cognitive Computing and Complex Data (ICCD)(2023)
摘要
Low-light image enhancement plays a very important role in computer vision. Due to the difficulty in acquiring paired samples, unsupervised learning methods have received increasing attention in recent years. The construction of the loss function plays a critical role in unsupervised methods, which often relies on the modeling of image priors. The RUAS method combines the modeling of physical imaging priors based on Retinex theory, the modeling of image geometric priors based on total variation, and the mining of priors based on network search, achieving good performance in low-light image enhancement. However, the single total variation geometric prior modeling is not suitable for preserving image color and details. In this paper, based on the RUAS algorithm, plug-and-play prior regularization terms are introduced into the loss function, and an ADMM-based unfolding optimization algorithm is designed. Numerical experiments show that the method proposed in this paper provides a flexible algorithmic framework for introducing plug-and-play priors, and the enhanced images also have better details.
更多查看译文
关键词
Low-light image enhancement,Retinex theory,Deep learning,Unfolding
AI 理解论文
溯源树
样例
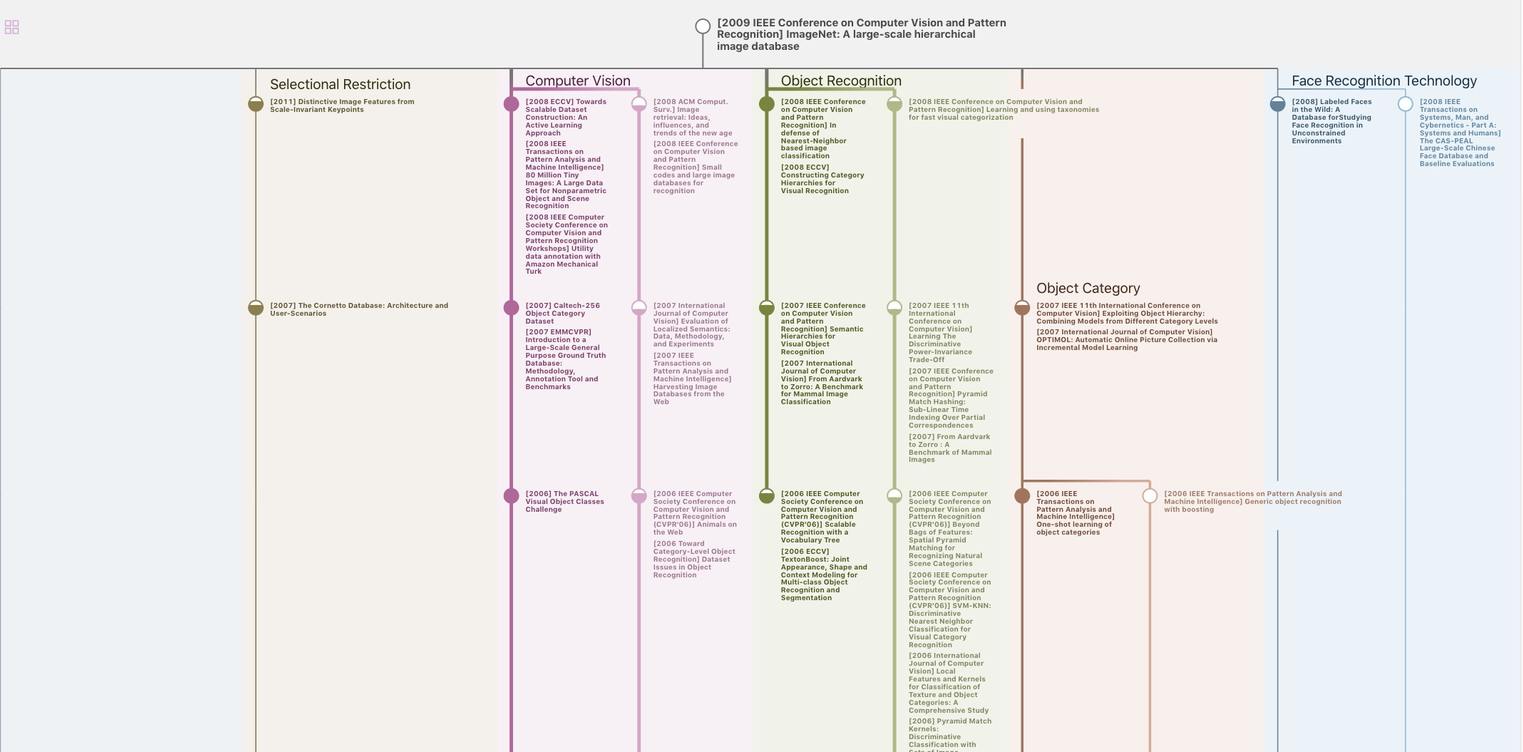
生成溯源树,研究论文发展脉络
Chat Paper
正在生成论文摘要