Models for predicting coffee yield from chemical characteristics of soil and leaves using machine learning
JOURNAL OF THE SCIENCE OF FOOD AND AGRICULTURE(2024)
摘要
BACKGROUNDCoffee farming constitutes a substantial economic resource, representing a source of income for several countries due to the high consumption of coffee worldwide. Precise management of coffee crops involves collecting crop attributes (characteristics of the soil and the plant), mapping, and applying inputs according to the plants' needs. This differentiated management is precision coffee growing and it stands out for its increased yield and sustainability.RESULTSThis research aimed to predict yield in coffee plantations by applying machine learning methodologies to soil and plant attributes. The data were obtained in a field of 54.6 ha during two consecutive seasons, applying varied fertilization rates in accordance with the recommendations of soil attribute maps. Leaf analysis maps also were monitored with the aim of establishing a correlation between input parameters and yield prediction. The machine-learning models obtained from these data predicted coffee yield efficiently. The best model demonstrated predictive fit results with a Pearson correlation of 0.86. Soil chemical attributes did not interfere with the prediction models, indicating that this analysis can be dispensed with when applying these models.CONCLUSIONThese findings have important implications for optimizing coffee management and cultivation, providing valuable insights for producers and researchers interested in maximizing yield using precision agriculture. (c) 2024 Society of Chemical Industry.
更多查看译文
关键词
biennial yield,coffee growing,digital farming,precision agriculture
AI 理解论文
溯源树
样例
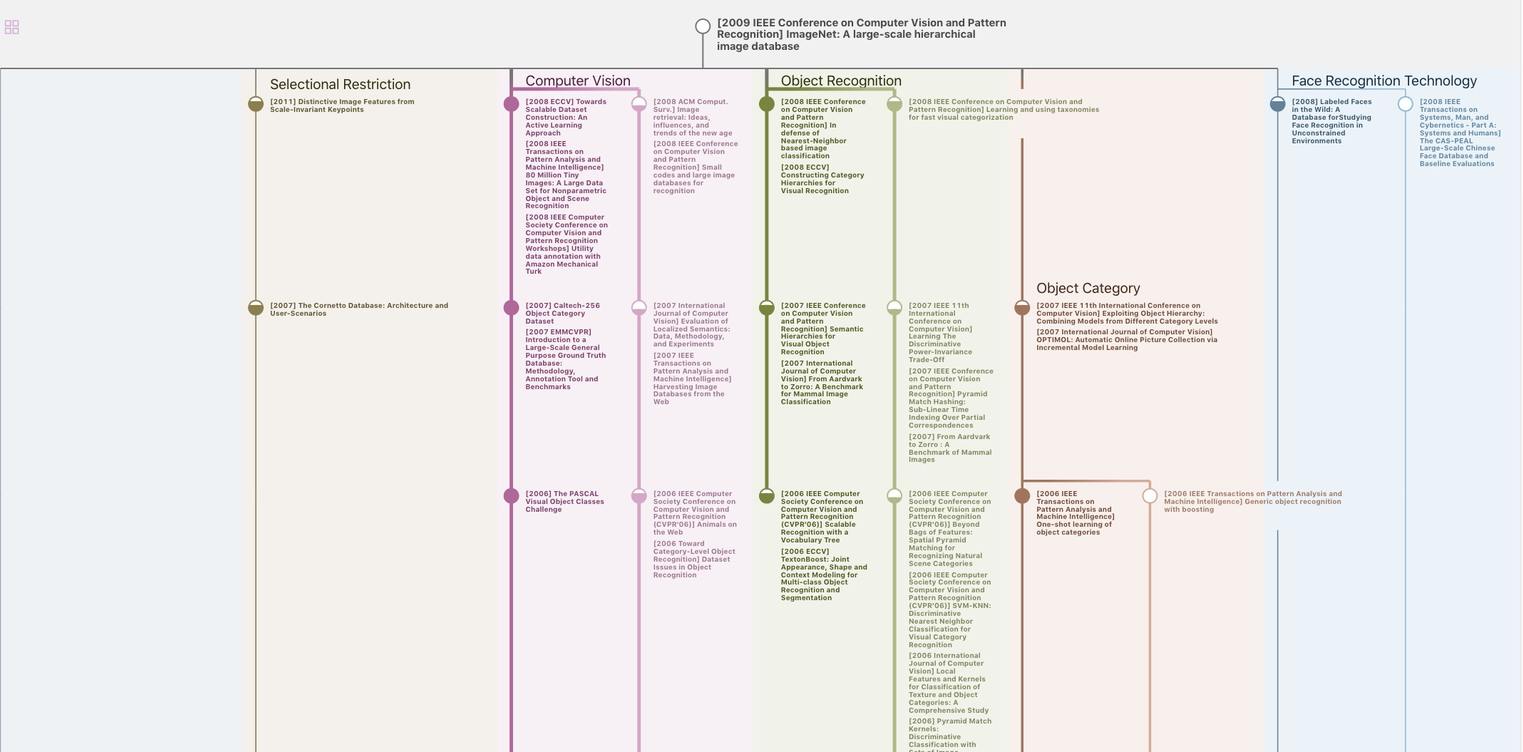
生成溯源树,研究论文发展脉络
Chat Paper
正在生成论文摘要