Review-Enhanced Sequential Recommendation with Self-Attention and Graph Collaborative Features
2023 23RD IEEE INTERNATIONAL CONFERENCE ON DATA MINING WORKSHOPS, ICDMW 2023(2023)
摘要
Recommender systems have gained significant popularity in recent years, with research focusing on three main branches: sequential recommendation, graph-based recommendation, and review-based recommendation. These branches leverage different data modalities to provide personalized recommendations. However, these individual modalities have their own biases and limitations, making it challenging to fully capture user preferences and item relations. Naive modality combination methods often face compatibility issues, leading to suboptimal performance. In this work, we propose a comprehensive model called RSSG (Review-enhanced Sequential recommendation with Self-attention and Graph collaborative features) to address this challenge. Our model finely integrates all three data modalities to provide more accurate and personalized recommendations. We utilize a language model to capture textual representations of users and items, as well as user-item attention weights. With shared user and item representations, we employ a time-interval aware self-attention mechanism to capture long-term user behavior patterns, and leverage graph attention networks to capture collaborative information between users and items. Finally, we fuse the sequential and collaborative features to make predictions. We conduct extensive experiments on various datasets to evaluate the performance of our proposed method. The experimental results demonstrate significant performance gains compared to state-of-the-art methods. Our approach effectively leverages the strengths of different data modalities, enabling more accurate and personalized recommendations for users.
更多查看译文
关键词
Sequential Recommendation,Graph Collaborative,Features for Recommendation
AI 理解论文
溯源树
样例
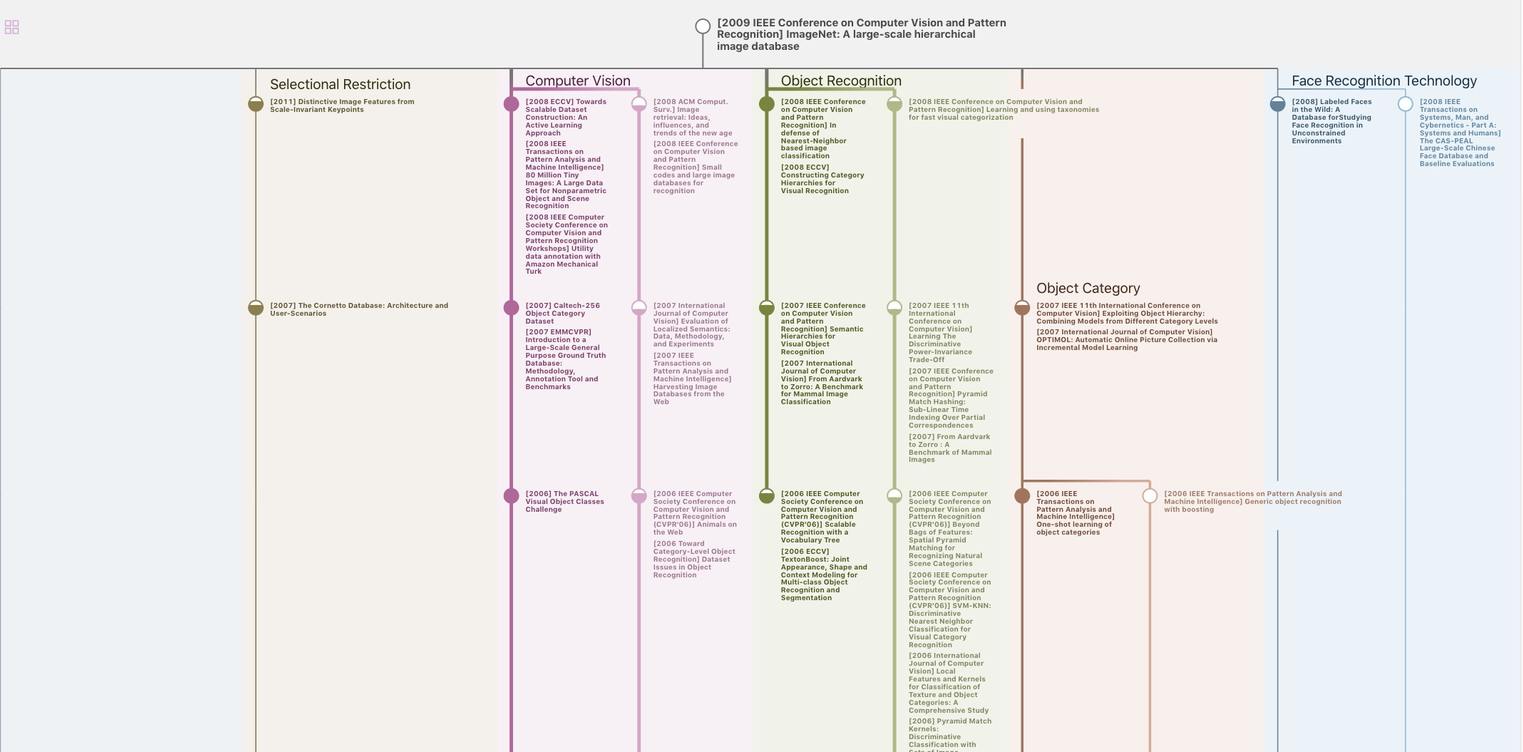
生成溯源树,研究论文发展脉络
Chat Paper
正在生成论文摘要