Hard Anomaly Detection: An Adversarial Data Augmentation Solution
2023 23RD IEEE INTERNATIONAL CONFERENCE ON DATA MINING WORKSHOPS, ICDMW 2023(2023)
摘要
We propose a measure to divide anomaly detection into four categories. At the same time, we focus on a special and representative type of anomaly detection problem, called hard anomaly detection. Compared with ordinary anomaly detection where anomalies usually appear as outliers, the so-called hard anomaly has two characteristics in terms of the differences between abnormal and normal samples: 1) the boundary is exceedingly unobvious, and 2) the distribution is extremely imbalanced, both of which result in the difficulty of anomaly detection. It is really hard for regular classifiers to find the boundary between abnormal and normal samples here. To address the issue of hard anomaly detection, in this work, we first propose a quantitative definition of the hard anomaly according to detection difficulty and then design a dedicated solution framework, named Hard Anomaly Detection (HAD). Under the framework HAD, we devise a GAN-based (Generative Adversarial Network) method, called HadGAN, which produces both abnormal and normal samples with a similar distribution. We then pre-train a base anomaly detection model using the data generated by HadGAN. Moreover, we apply transfer learning to fine-tune the base model on real datasets. The superiority of our solution is demonstrated both by theoretical analysis as well as in extensive experiments.
更多查看译文
关键词
hard anomaly detection,GAN,data imbalance,data generation,model enhancement
AI 理解论文
溯源树
样例
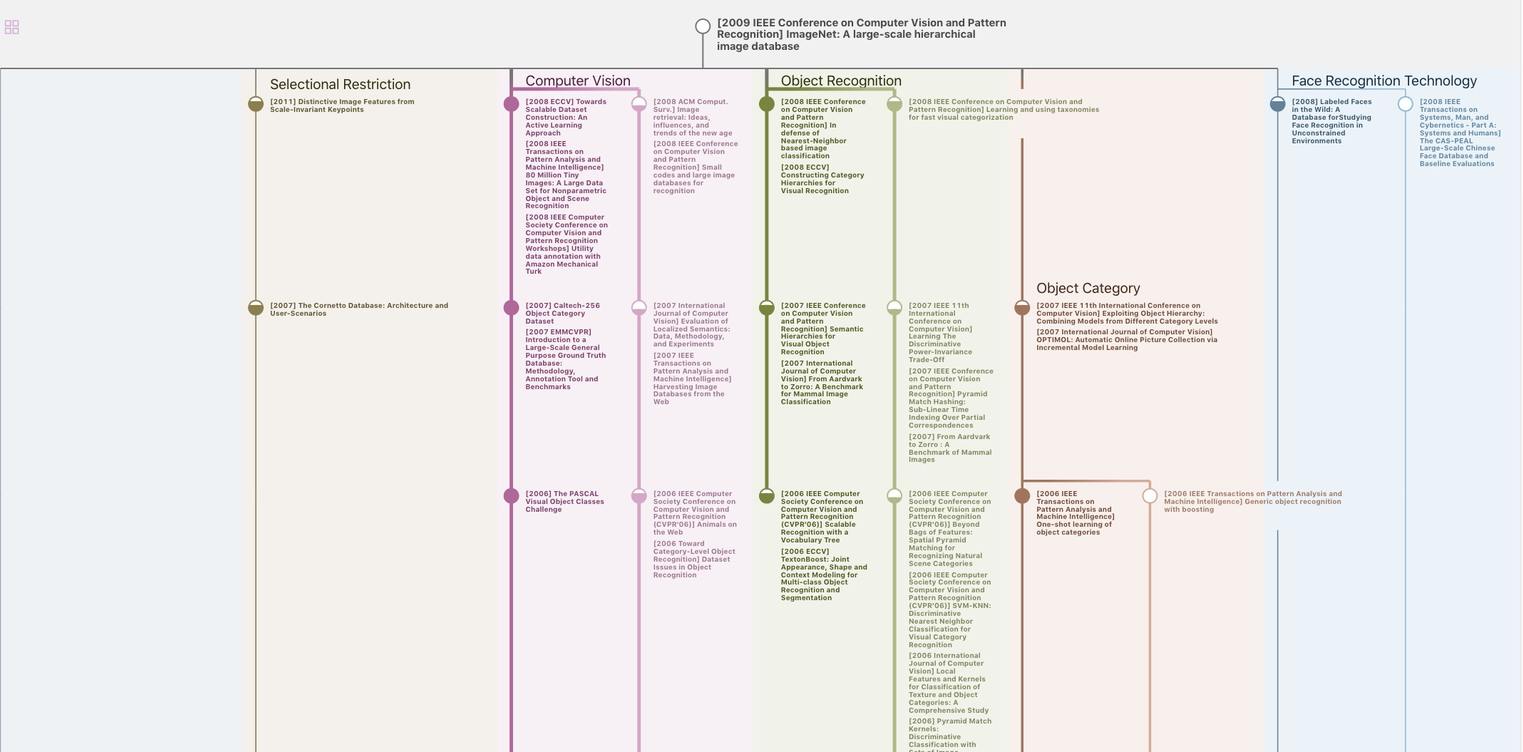
生成溯源树,研究论文发展脉络
Chat Paper
正在生成论文摘要