Influence Propagation for Linear Threshold Model with Graph Neural Networks
2023 23RD IEEE INTERNATIONAL CONFERENCE ON DATA MINING WORKSHOPS, ICDMW 2023(2023)
摘要
Influence propagation is a network phenomenon governing how information is diffused in a network. With the advent of deep learning, there has been growing interest in applying graph neural networks to extract salient feature representation of the nodes for a variety of network mining tasks, such as forecasting the virality of information cascade. Given the importance of social influence, this paper presents a novel deep learning framework called IP-GNN for simulating the information propagation process in a complex network and learning a node representation that embeds information about the diffusion process under the linear threshold model. Our framework employs a modified graph convolutional network architecture with adaptive diffusion kernel to capture long-range propagation of information along with an entropy-regularized mixture of loss functions to ensure accurate prediction and faster convergence of the learning algorithm. Experimental results on 4 real-world datasets show that the model accurately mimics the output of the linear threshold model, achieving an average accuracy that exceeds 90% on all datasets.
更多查看译文
关键词
Influence propagation,Deep Learning,Graph Neural Network
AI 理解论文
溯源树
样例
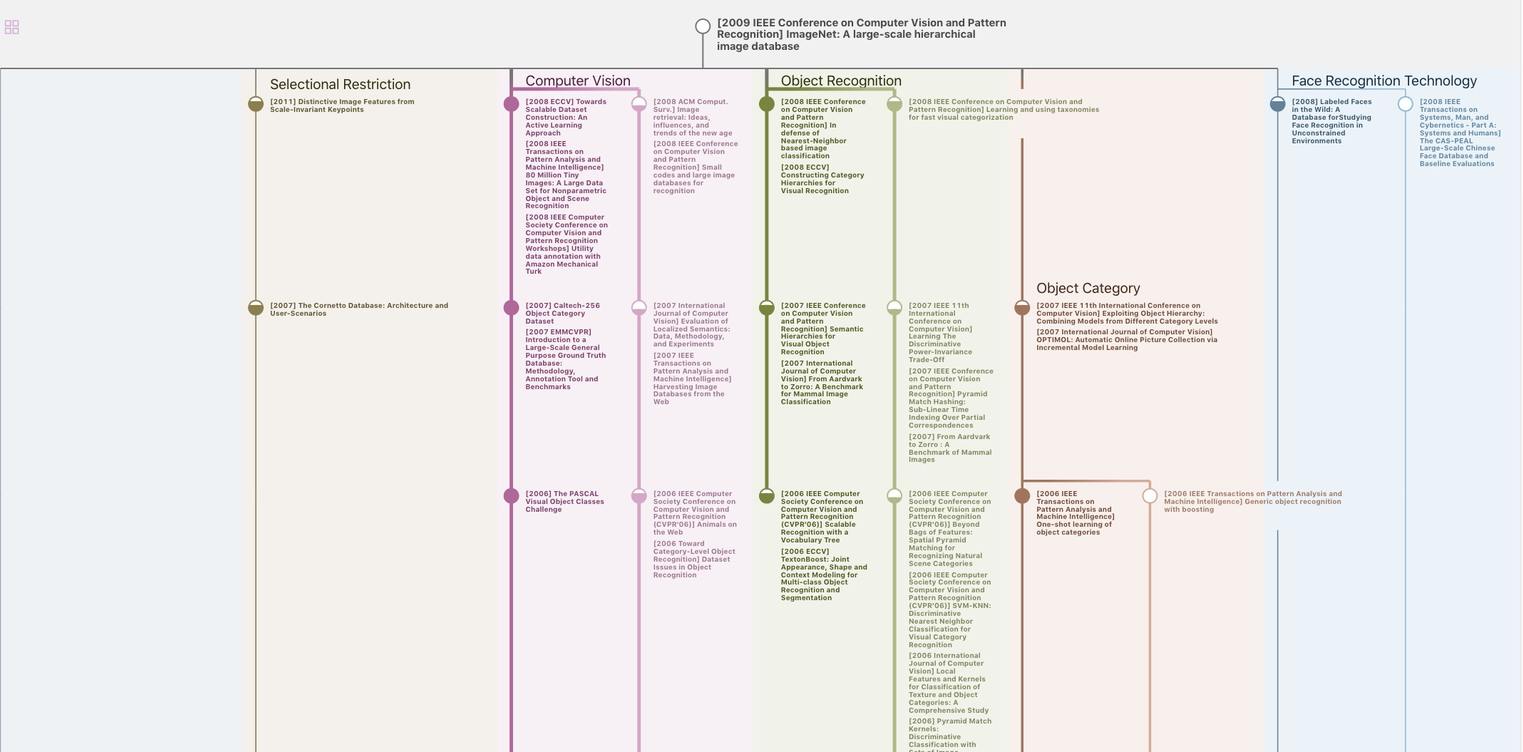
生成溯源树,研究论文发展脉络
Chat Paper
正在生成论文摘要