Match and Retrieval: Legal Similar Case Retrieval via Graph Matching Network
2023 23RD IEEE INTERNATIONAL CONFERENCE ON DATA MINING WORKSHOPS, ICDMW 2023(2023)
摘要
Legal document retrieval is a crucial application in the field of legal Artificial Intelligence (AI). It involves retrieving the most relevant legal cases from a legal document database when inputting a query. Mainstream solutions typically employ pretrained language models or large language models for retrieval. However, both of these methods rely on black-box models trained on unlabeled data, resulting in a lack of interpretability in the model's retrieval outcomes. Graph Neural Networks (GNNs) possess strong representational and interpretable capabilities. Therefore, representing legal cases using graph-structured data and performing the Legal Similar Case Retrieval (LSCR) task through GNNs is a promising approach. Nevertheless, typical GNNs primarily focus on node information within graph-structured data and overlook edge and inter-graph interaction information, leading to lower retrieval accuracy. To address these issues, in this paper, we propose a Legal case retrieval model utilizing a Graph Matching Network, called Legal-GMN. We evaluate the model on a dataset composed of real legal judgment cases. Experimental results demonstrate that Legal-GMN effectively enhances the retrieval accuracy for the LSCR task. Compared with baseline methods, Legal-GMN improves retrieval precision by approximately 15% and enhances retrieval efficiency by approximately 90%. Remarkably, while maintaining State-Of-The-Art (SOTA) performance, Legal-GMN can also generate visualized graphs of the retrieval process, significantly enhancing its interpretability.
更多查看译文
关键词
legal case retrieval,legal intelligence,graph neural network,graph matching network,text similarity
AI 理解论文
溯源树
样例
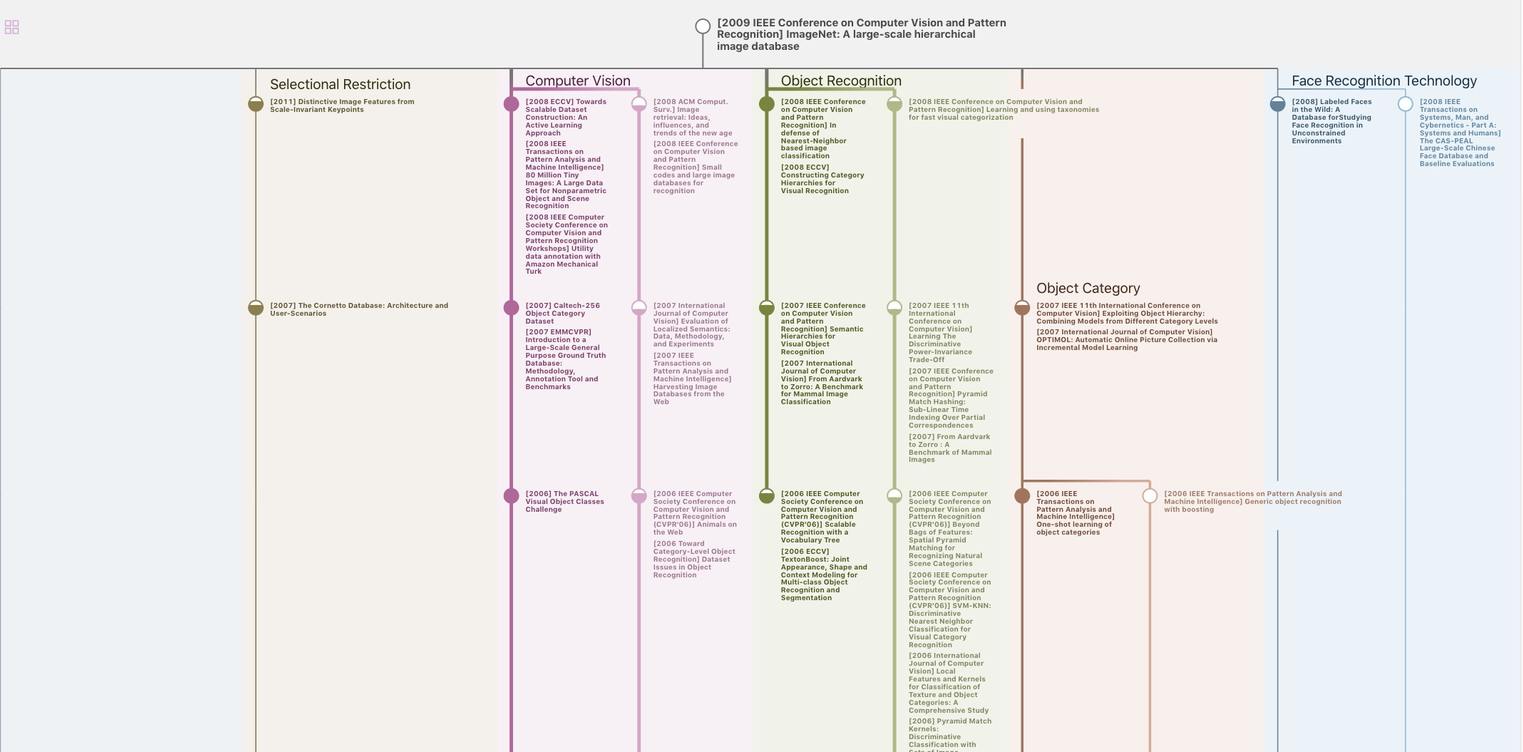
生成溯源树,研究论文发展脉络
Chat Paper
正在生成论文摘要