Towards graph-based forecasting with attention for real-world restaurant sales
2023 23RD IEEE INTERNATIONAL CONFERENCE ON DATA MINING WORKSHOPS, ICDMW 2023(2023)
摘要
Forecasting is the process of predicting future events or values based on past data to support, for instance, strategic business decisions. This historical data often takes the form of multivariate time series. Recently, graph networks emerged as a powerful forecasting framework by considering spatiotemporal relations in the data. In this paper, we describe the adoption of a forecasting model built in an encoder-decoder architecture. The encoder projects the spatiotemporal relations into a lower dimension subspace, whereas the decoder uses the generated embeddings to produce the predicted value for a given period. We test the model on three datasets with data from different areas. Two datasets are public benchmarks with data from disease spread and street traffic scenarios which are used to evaluate the performance of our architecture. Additionally, we also evaluate our model on a new private company-owned dataset with product sales on a chain of restaurants. This dataset is more diverse, especially in terms of variety across different restaurants. We provide a deep dive on the challenges posed by it. We experiment with various state-of-the-art models to compare the performances on all datasets. The results show that our model obtains overall good predictions, outperforming all SOTA models, in terms of accuracy, by 5.2% and 9.8%, on the benchmark datasets. On the real-world restaurant data, our model outperforms all benchmarks and surpasses the second best by 140%.
更多查看译文
关键词
Time series,Forecasting,Deep Learning,Graph Networks.
AI 理解论文
溯源树
样例
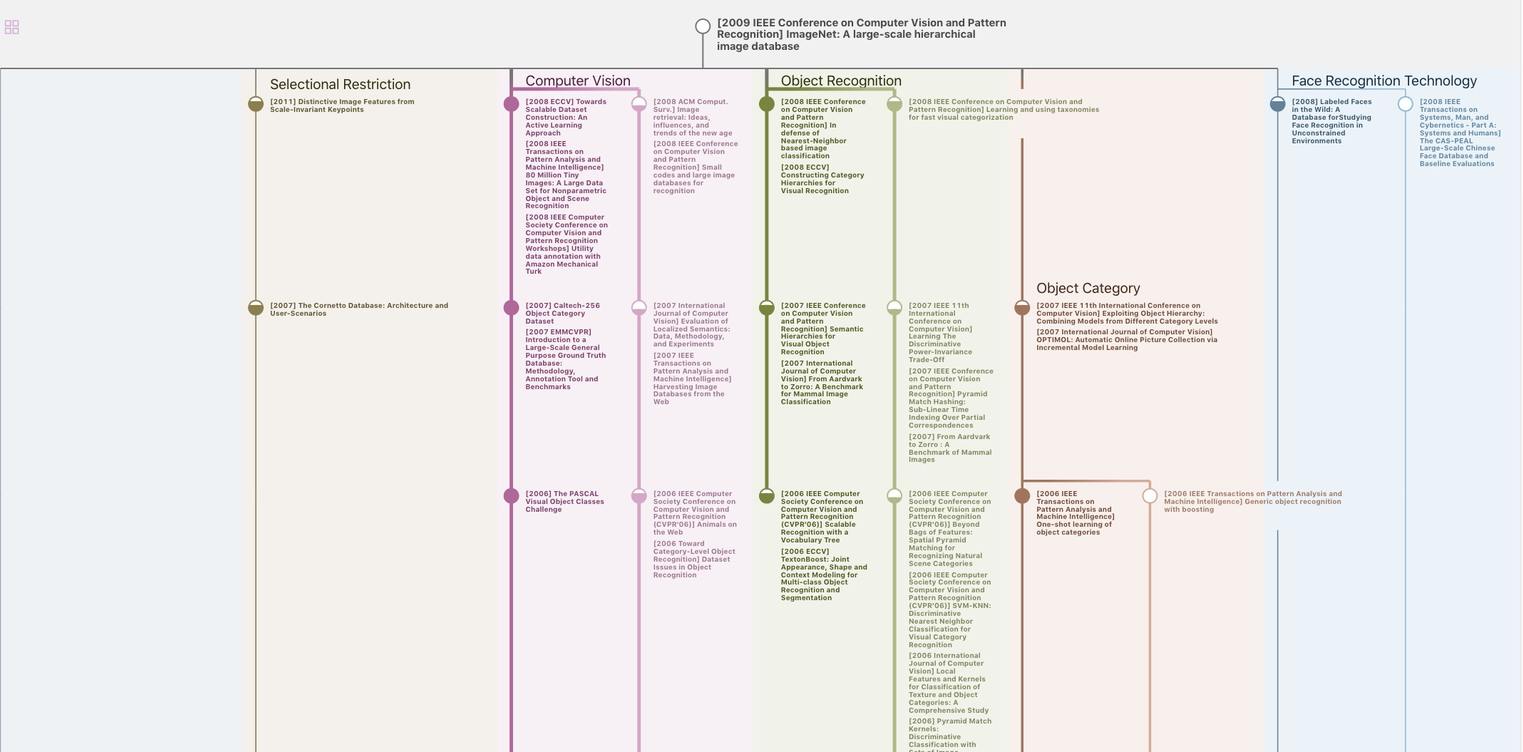
生成溯源树,研究论文发展脉络
Chat Paper
正在生成论文摘要