Variational Shapley Network: A Probabilistic Approach to Self-Explaining Shapley values with Uncertainty Quantification
CoRR(2024)
摘要
Shapley values have emerged as a foundational tool in machine learning (ML)
for elucidating model decision-making processes. Despite their widespread
adoption and unique ability to satisfy essential explainability axioms,
computational challenges persist in their estimation when (i) evaluating a
model over all possible subset of input feature combinations, (ii) estimating
model marginals, and (iii) addressing variability in explanations. We
introduce a novel, self-explaining method that simplifies the computation of
Shapley values significantly, requiring only a single forward pass. Recognizing
the deterministic treatment of Shapley values as a limitation, we explore
incorporating a probabilistic framework to capture the inherent uncertainty in
explanations. Unlike alternatives, our technique does not rely directly on the
observed data space to estimate marginals; instead, it uses adaptable baseline
values derived from a latent, feature-specific embedding space, generated by a
novel masked neural network architecture. Evaluations on simulated and real
datasets underscore our technique's robust predictive and explanatory
performance.
更多查看译文
AI 理解论文
溯源树
样例
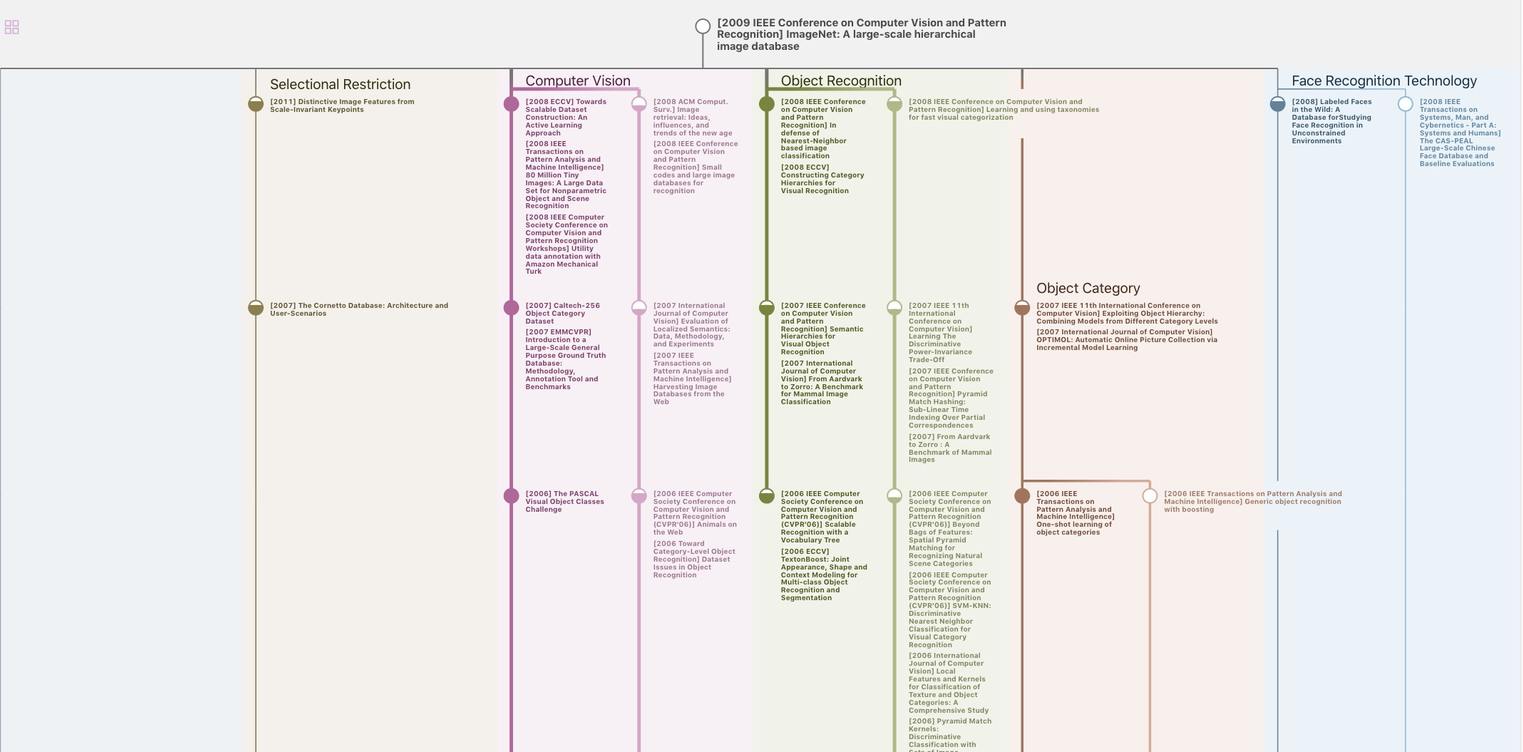
生成溯源树,研究论文发展脉络
Chat Paper
正在生成论文摘要