Reducing the Cost of Quantum Chemical Data By Backpropagating Through Density Functional Theory
CoRR(2024)
摘要
Density Functional Theory (DFT) accurately predicts the quantum chemical
properties of molecules, but scales as O(N_electrons^3). Schütt et
al. (2019) successfully approximate DFT 1000x faster with Neural Networks (NN).
Arguably, the biggest problem one faces when scaling to larger molecules is the
cost of DFT labels. For example, it took years to create the PCQ dataset
(Nakata Shimazaki, 2017) on which subsequent NNs are trained within a week.
DFT labels molecules by minimizing energy E(· ) as a "loss function." We
bypass dataset creation by directly training NNs with E(· ) as a loss
function. For comparison, Schütt et al. (2019) spent 626 hours creating a
dataset on which they trained their NN for 160h, for a total of 786h; our
method achieves comparable performance within 31h.
更多查看译文
AI 理解论文
溯源树
样例
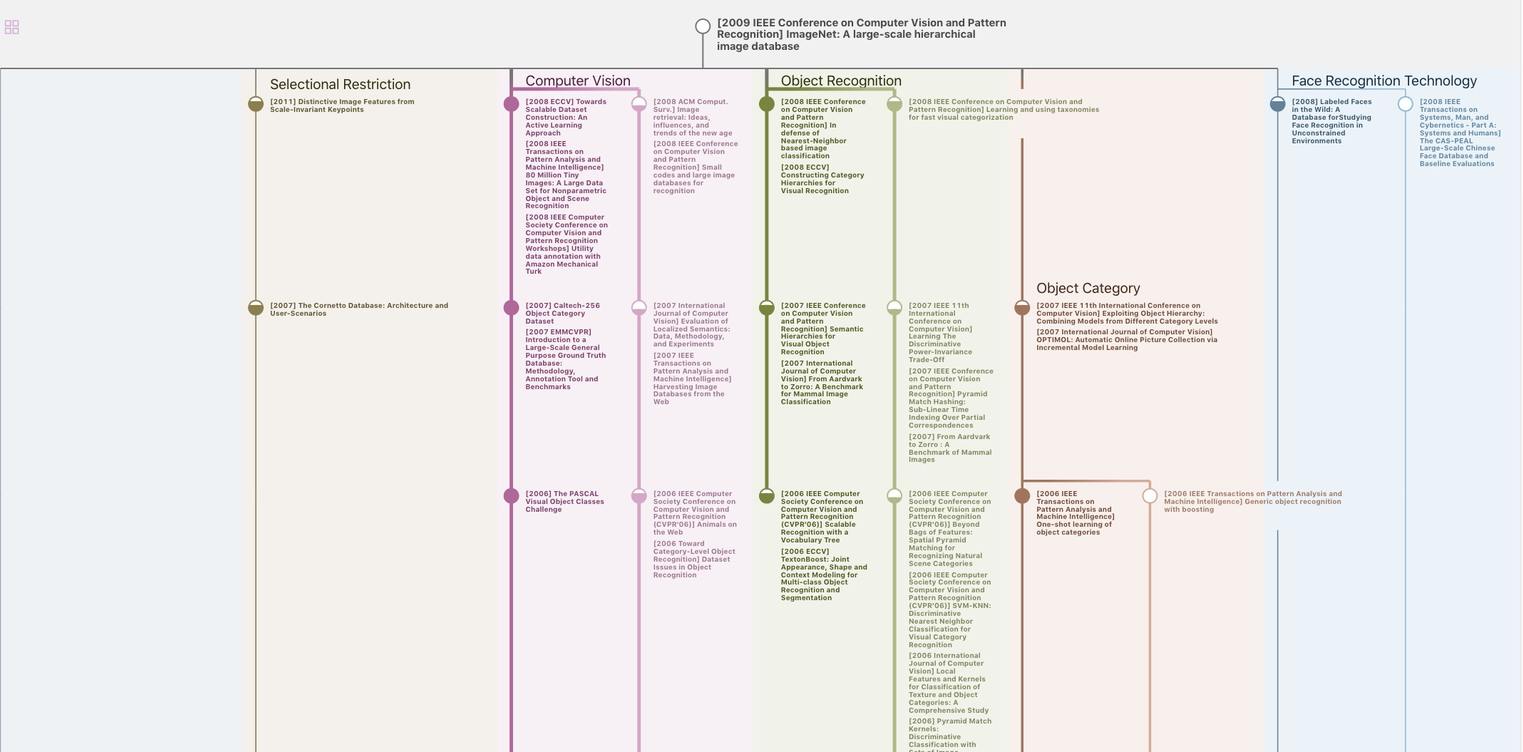
生成溯源树,研究论文发展脉络
Chat Paper
正在生成论文摘要