Privacy Leakage on DNNs: A Survey of Model Inversion Attacks and Defenses
CoRR(2024)
摘要
Model Inversion (MI) attacks aim to disclose private information about the
training data by abusing access to the pre-trained models. These attacks enable
adversaries to reconstruct high-fidelity data that closely aligns with the
private training data, which has raised significant privacy concerns. Despite
the rapid advances in the field, we lack a comprehensive overview of existing
MI attacks and defenses. To fill this gap, this paper thoroughly investigates
this field and presents a holistic survey. Firstly, our work briefly reviews
the traditional MI on machine learning scenarios. We then elaborately analyze
and compare numerous recent attacks and defenses on Deep
Neural Networks (DNNs) across multiple modalities and
learning tasks.
更多查看译文
AI 理解论文
溯源树
样例
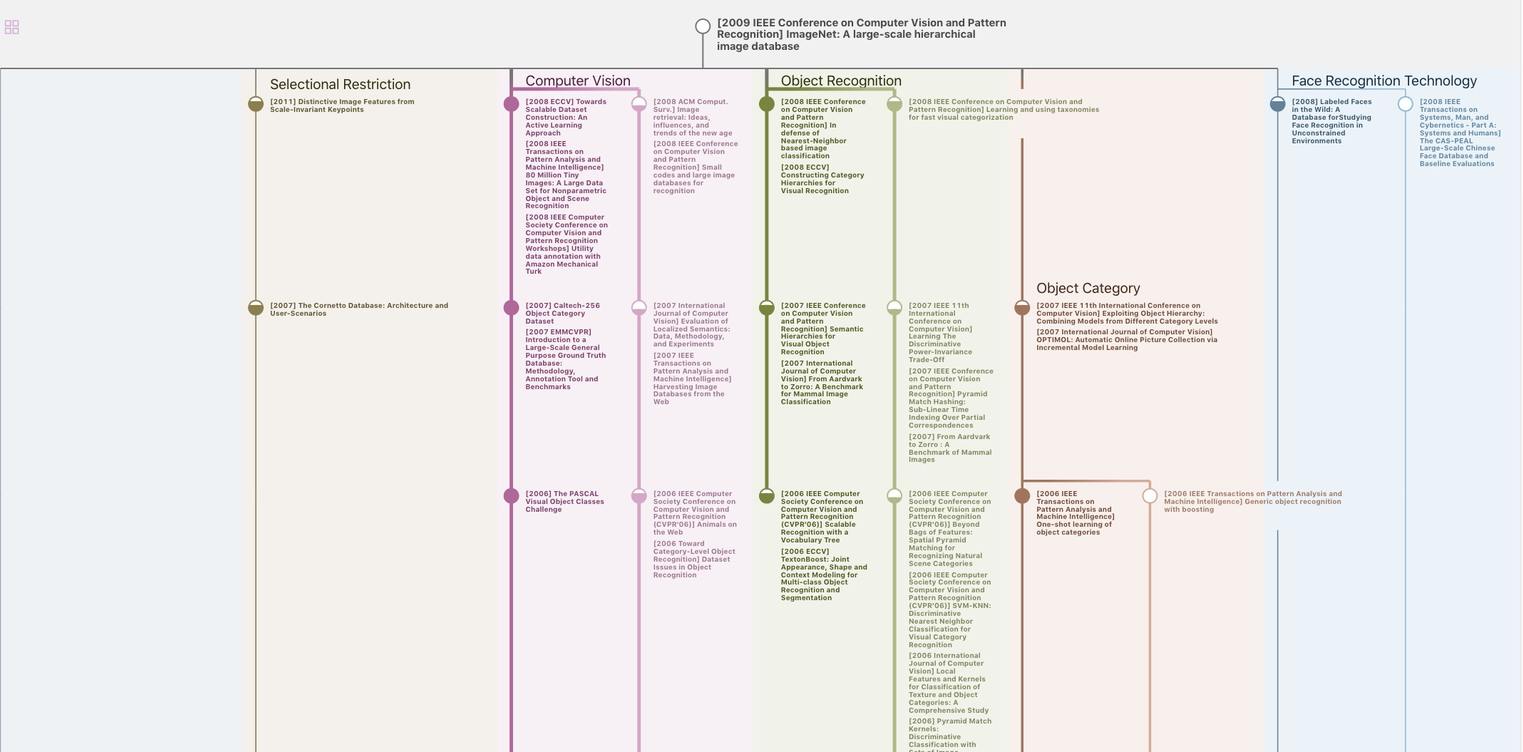
生成溯源树,研究论文发展脉络
Chat Paper
正在生成论文摘要