Machine learning-based risk prediction of hypoxemia for outpatients undergoing sedation colonoscopy: a practical clinical tool
POSTGRADUATE MEDICINE(2024)
摘要
ObjectivesHypoxemia as a common complication in colonoscopy under sedation and may result in serious consequences. Unfortunately, a hypoxemia prediction model for outpatient colonoscopy has not been developed. Consequently, the objective of our study was to develop a practical and accurate model to predict the risk of hypoxemia in outpatient colonoscopy under sedation.MethodsIn this study, we included patients who received colonoscopy with anesthesia in Nanjing First Hospital from July to September 2021. Risk factors were selected through the least absolute shrinkage and selection operator (LASSO). Prediction models based on logistic regression (LR), random forest classifier (RFC), extreme gradient boosting (XGBoost), support vector machine (SVM), and stacking classifier (SCLF) model were implemented and assessed by standard metrics such as the area under the receiver operating characteristic curve (AUROC), sensitivity and specificity. Then choose the best model to develop an online tool for clinical use.ResultsWe ultimately included 839 patients. After LASSO, body mass index (BMI) (coefficient = 0.36), obstructive sleep apnea-hypopnea syndrome (OSAHS) (coefficient = 1.32), basal oxygen saturation (coefficient = -0.14), and remifentanil dosage (coefficient = 0.04) were independent risk factors for hypoxemia. The XGBoost model with an AUROC of 0.913 showed the best performance among the five models.ConclusionOur study selected the XGBoost as the first model especially for colonoscopy, with over 95% accuracy and excellent specificity. The XGBoost includes four variables that can be quickly obtained. Moreover, an online prediction practical tool has been provided, which helps screen high-risk outpatients with hypoxemia swiftly and conveniently. Colonoscopy under sedation is an effective technique for the inspection and treatment of alimentary canal diseases, but hypoxemia associated with this process cannot be ignored, since prolonged or severe hypoxemia may result in several serious consequences.We wanted to develop a practical and accurate model to predict the risk of hypoxemia for outpatient colonoscopy under sedation, which could help clinicians make more accurate and objective judgments to prevent patients from being harmed.A total of 839 patients were included in our study and we constructed five machine learning models and selected the best one, which demonstrated satisfactory performance. On this basis, a user-friendly data interface has been developed for convenient application. Clinicians can log in to this interface at any time and it will automatically calculate the patient's risk of hypoxemia when entering patient information.This study offers evidence that machine learning algorithms can accurately predict the risk of hypoxemia for outpatient colonoscopy under sedation and the model we developed is a practical and interpretable tool that could be used as a clinical decision-making aid.
更多查看译文
关键词
Hypoxemia,colonoscopy,anesthesia,machine learning,prediction,outpatient
AI 理解论文
溯源树
样例
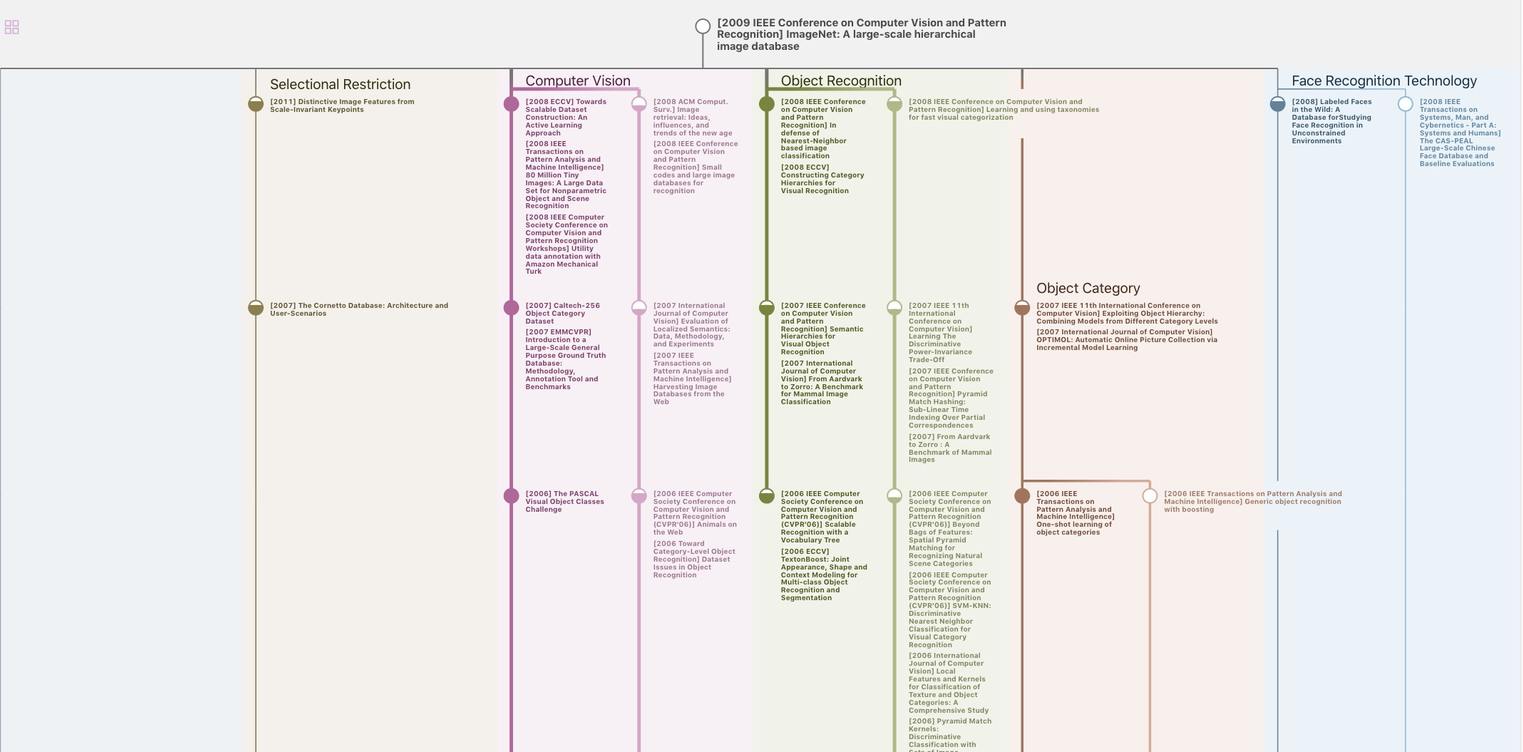
生成溯源树,研究论文发展脉络
Chat Paper
正在生成论文摘要