Neural Contextual Combinatorial Bandit under Non -stationary Environment
23RD IEEE INTERNATIONAL CONFERENCE ON DATA MINING, ICDM 2023(2023)
摘要
Classic contextual combinatorial multi -armed bandit problems aim to maximize the expected cumulative joint reward in the long run, where a learner plays a set of arms (i.e., a super arm) with time-invariant linear rewards of context features in each round. However, in many real-world applications, linearreward assumptions often fail to be satisfied and the environment is in general non -stationary, leading to low performance with the bandit models above. Existing works fail to deal with non-linear rewards in the non -stationary environment and the algorithmic challenge remains. In this paper, we initiate the study of a non -stationary neural contextual combinatorial bandit problem, where the reward function of each individual arm can be estimated by a deep neural network based on boundedness assumption and a time -variant reward mapping function. Furthermore, we design an algorithm NNCMAB, which dynamically partitions the context subspace into multiple subspaces and fits reward mapping functions for each subspace by neural networks such that only the models of related subspaces are re-trained when local environment changes happen. NNCMAB can provably achieve (O) over tilde (T-3/4 + root T N-c) regret, where T is the number of rounds, and N-c is a parameter associated with the distribution change. Evaluation results under synthetic and real -world LastFM datasets show that NNCMAB significantly outperforms other state-of-the-art with both linear and non-linear individual rewards under non -stationary environments.
更多查看译文
关键词
Non-stationary Environments,Combinatorial Bandit,Neural Network,Environmental Changes,Deep Neural Network,Changes In Distribution,Local Changes,Real-world Applications,Real-world Datasets,Combinatorial Problem,Reward Function,Problem Context,Changes In The Local Environment,Arm Function,Multi-armed Bandit,Individual Reward,Bandit Problem,Upper Bound,Linear Function,Nonlinear Function,Reward Model,Reward Distribution,Beginning Of Each Round,Change Point,Static Environment,Occurrence Of Changes,Sliding Window Technique,End Of Round,Neural Algorithm,Time Slot
AI 理解论文
溯源树
样例
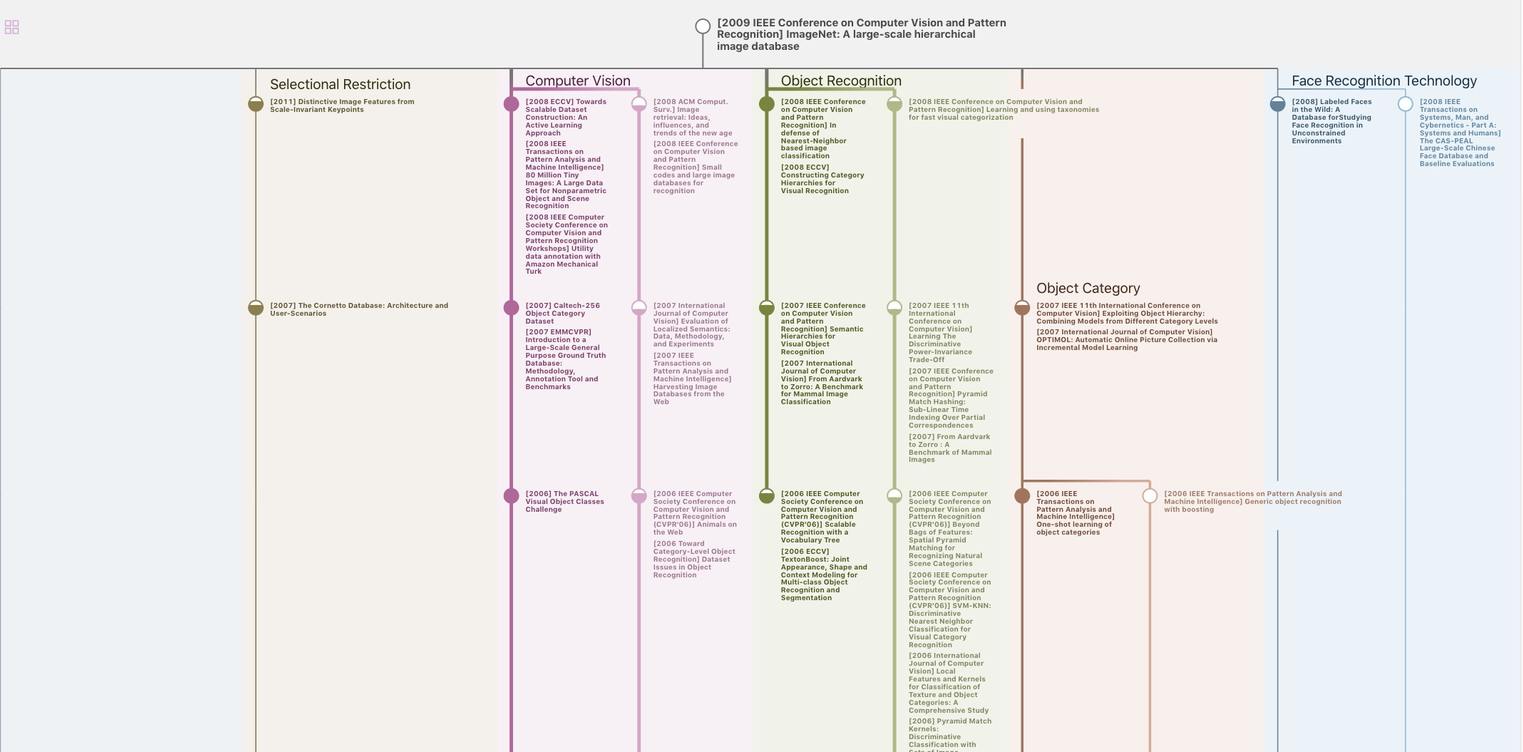
生成溯源树,研究论文发展脉络
Chat Paper
正在生成论文摘要