A Progressive Sampling Method for Dual -Node Imbalanced Learning with Restricted Data Access
23RD IEEE INTERNATIONAL CONFERENCE ON DATA MINING, ICDM 2023(2023)
摘要
Imbalanced learning, characterised by disproportionate class distributions, impedes the effectiveness of learning algorithms, particularly when available data is scarce. Although the utilisation of external data sources can alleviate these challenges, complete access to such resources is often hampered by privacy regulations or lack of annotations, further complicating the imbalanced learning problem. Additionally, exploiting all data from an external node may not be efficient due to data redundancy and computational constraints. To navigate these issues, this paper introduces an innovative solution for unbalanced learning with restricted data access. We propose a data selection method focused on selecting balanced data from the data -rich but restricted node, prioritising diversity, informativeness and balance. Our strategy mitigates the need Inr exhaustive data exploration and promotes efficient use of the available data. To further enhance the robustness of data selection, we present an iterative method that progressively selects balanced data. The iterative process, involving training a fully supervised model on the data -shortage node and a contrastive model on the data -rich node, incrementally refines the balance of selected data. Additionally, our method employs prediction entropy to automatically generate weights for training the contrastive models, a distinct improvement over manual weight specification. We validate the effectiveness of our approach through extensive experimentation and demonstrate that our proposed methodology addresses the challenges of imbalanced learning under restricted data access, leading to improved data utilisation, enhanced balance, and better representation in imbalanced learning scenarios. The code is available on Gitllub at https://github.com/ugyqiu/CPSI-
更多查看译文
关键词
Imbalanced learning,privacy,data sampling
AI 理解论文
溯源树
样例
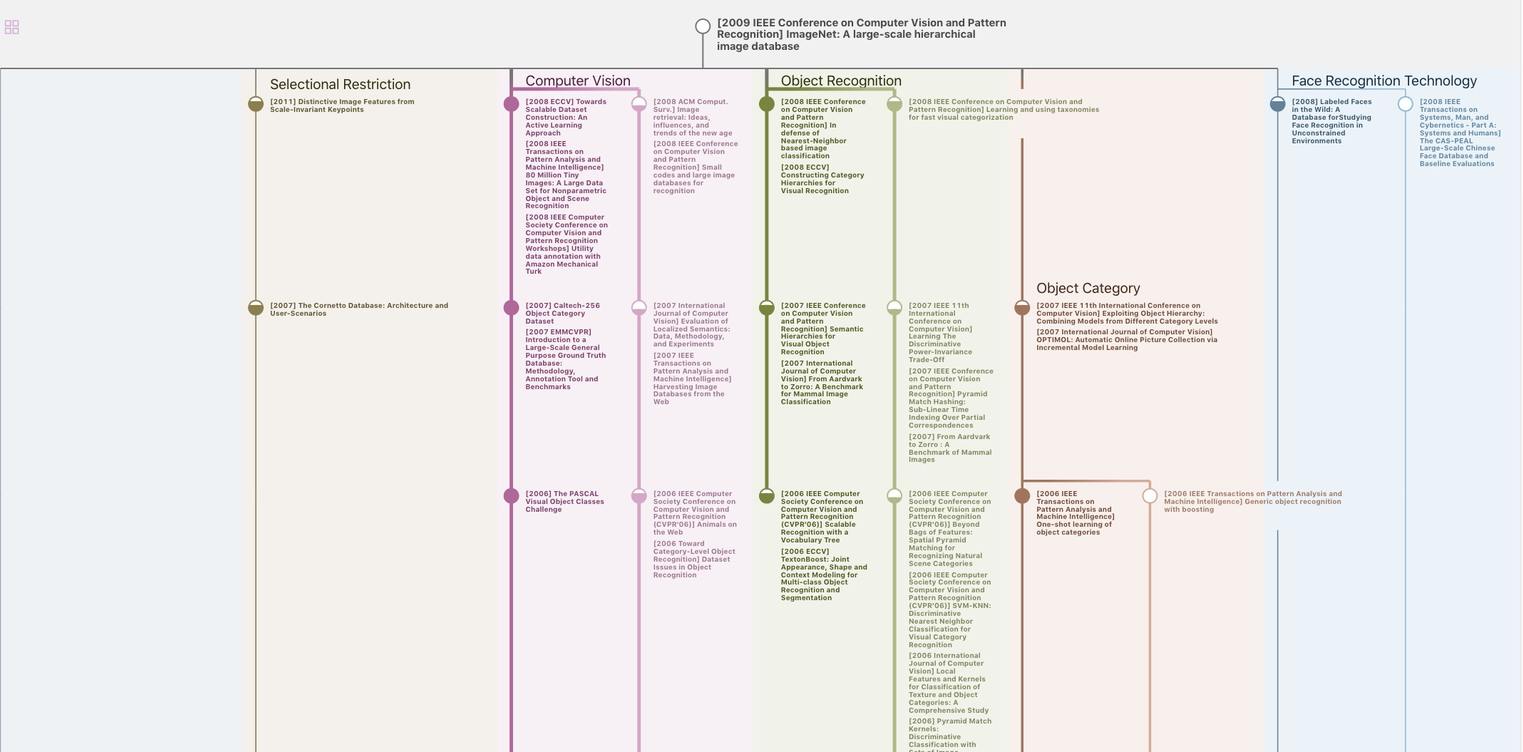
生成溯源树,研究论文发展脉络
Chat Paper
正在生成论文摘要