Online Ensemble of Ensemble OVA Framework for Class Evolution with Dominant Emerging Classes
23RD IEEE INTERNATIONAL CONFERENCE ON DATA MINING, ICDM 2023(2023)
摘要
In real-world data stream mining, the composition of classes undergoes unpredictable changes, giving rise to the challenge of class evolution, encompassing class emergence, disappearance, and reoccurrence. However, most existing approaches require the storage of past data to adapt their model. While some studies have focused on online learning approaches, they are built on an underlying assumption that the number of instances in any single class is consistently less than the sum of other classes. This assumption becomes invalid when a class emerges with a dominant amount, e.g., news about a pandemic outbreak, harming the performance of existing methods. In this paper, we thoroughly investigate this scenario and propose a novel online ensemble of ensemble one-versus-all framework (EWE) to handle class evolution adaptively. The novel ensemble of ensemble architecture boosts diversity in each one-versus-all classifier. A novel adaptive model adaptation method is also designed to balance the error feedback between the emerging class and the other classes. A confidence-triggered fallback mode is integrated to prevent performance drop due to a wrong decision regarding class disappearance. Experimental studies are conducted on both synthetic and real-world data streams to show that our method achieves higher accuracy in diverse class evolution scenarios compared with the state-of-the-art method, particularly when classes emerge with dominant amounts.
更多查看译文
关键词
data stream mining,online learning,class evolution,ensemble model
AI 理解论文
溯源树
样例
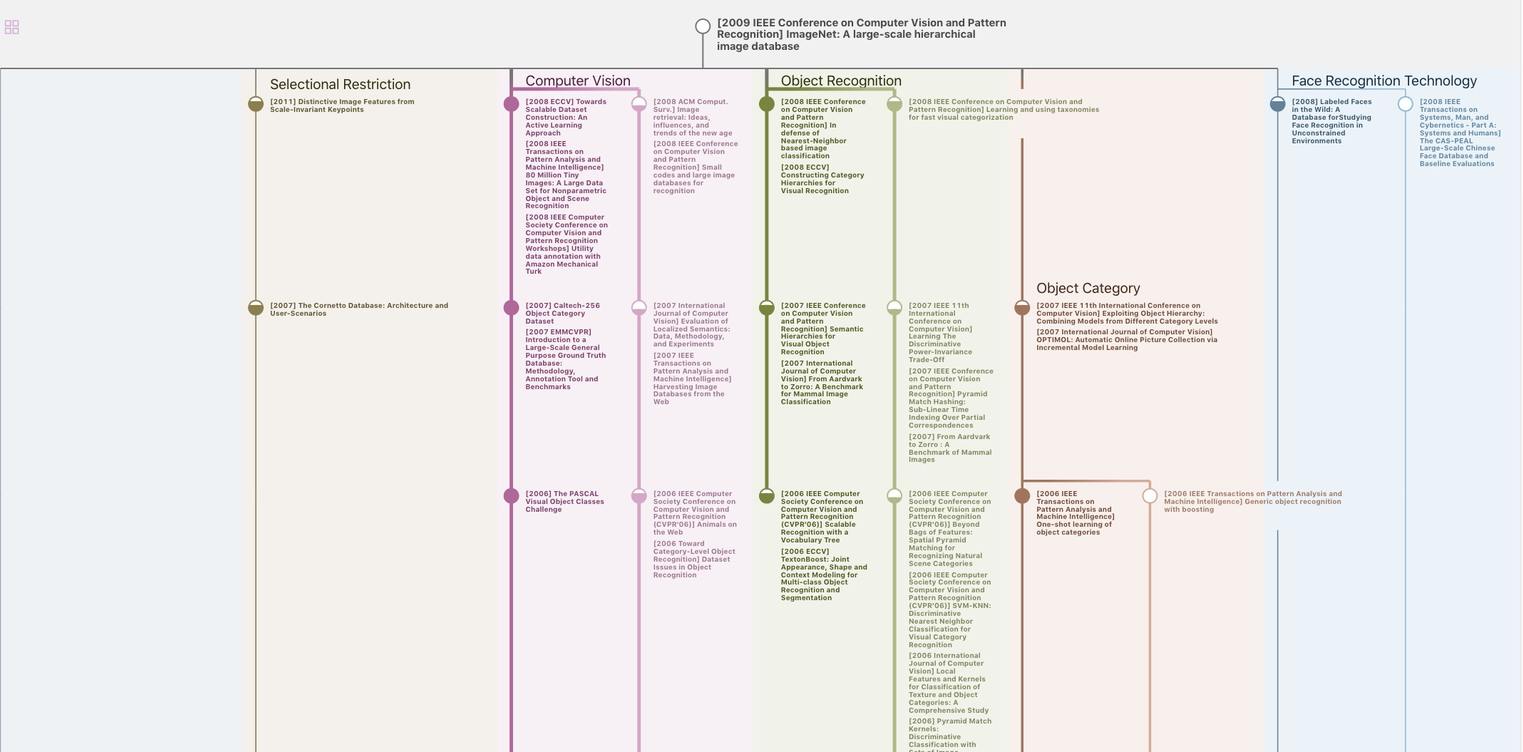
生成溯源树,研究论文发展脉络
Chat Paper
正在生成论文摘要