Assessing the Performance of a Dynamical Downscaling Simulation Driven by a Bias-Corrected CMIP6 Dataset for Asian Climate
Advances in Atmospheric Sciences(2024)
摘要
In this study, we aim to assess dynamical downscaling simulations by utilizing a novel bias-corrected global climate model (GCM) data to drive a regional climate model (RCM) over the Asia-western North Pacific region. Three simulations were conducted with a 25-km grid spacing for the period 1980–2014. The first simulation (WRF_ERA5) was driven by the European Centre for Medium-Range Weather Forecasts Reanalysis 5 (ERA5) dataset and served as the validation dataset. The original GCM dataset (MPI-ESM1-2-HR model) was used to drive the second simulation (WRF_GCM), while the third simulation (WRF_GCMbc) was driven by the bias-corrected GCM dataset. The bias-corrected GCM data has an ERA5-based mean and interannual variance and long-term trends derived from the ensemble mean of 18 CMIP6 models. Results demonstrate that the WRFGCMbc significantly reduced the root-mean-square errors (RMSEs) of the climatological mean of downscaled variables, including temperature, precipitation, snow, wind, relative humidity, and planetary boundary layer height by 50%–90% compared to the WRF_GCM. Similarly, the RMSEs of interannual-to-interdecadal variances of downscaled variables were reduced by 30%–60%. Furthermore, the WRFGCMbc better captured the annual cycle of the monsoon circulation and intraseasonal and day-to-day variabilities. The leading empirical orthogonal function (EOF) shows a monopole precipitation mode in the WRFGCM. In contrast, the WRF_GCMbc successfully reproduced the observed tri-pole mode of summer precipitation over eastern China. This improvement could be attributed to a better-simulated location of the western North Pacific subtropical high in the WRF_GCMbc after GCM bias correction.
更多查看译文
关键词
bias correction,multi-model ensemble mean,dynamical downscaling,interannual variability,day-to-day variability,validation
AI 理解论文
溯源树
样例
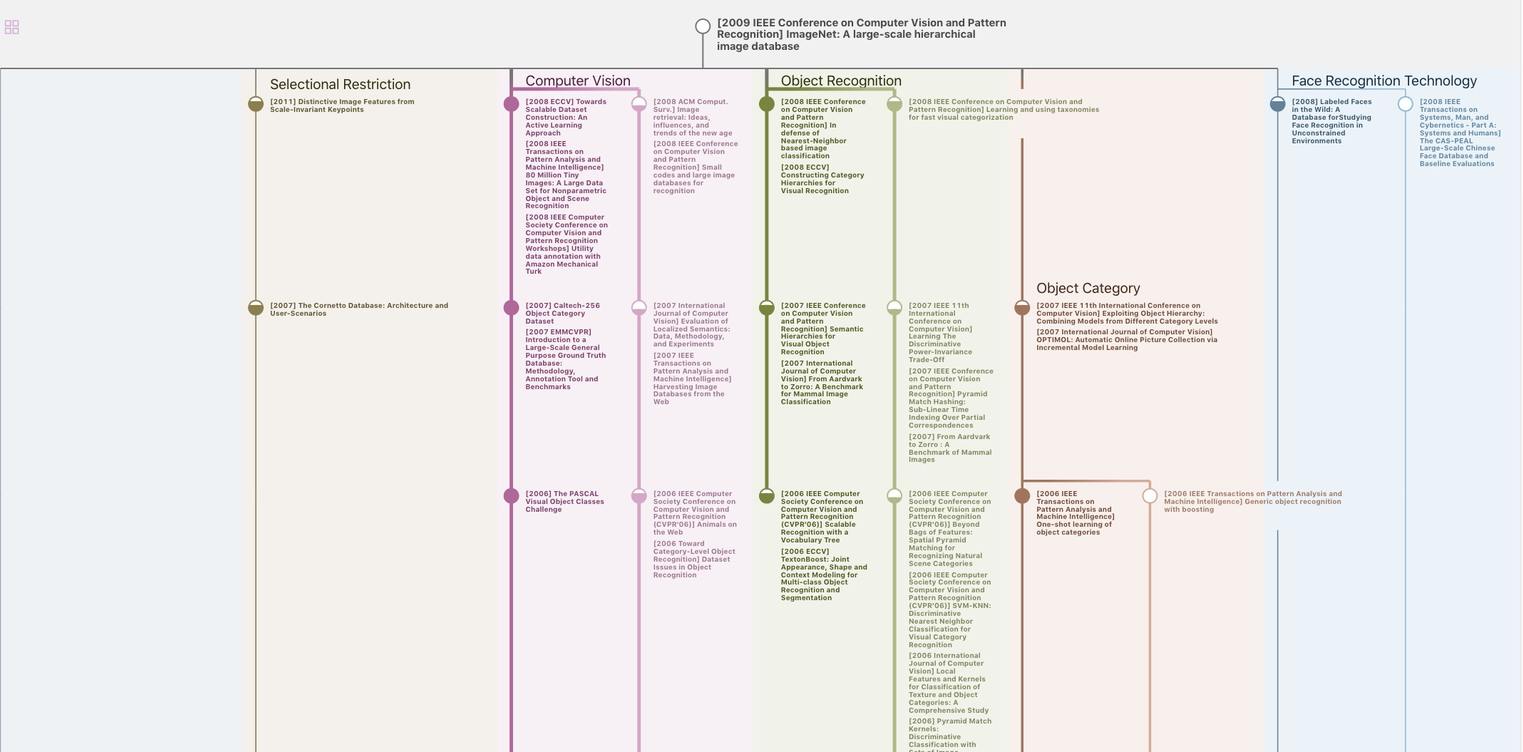
生成溯源树,研究论文发展脉络
Chat Paper
正在生成论文摘要