Investigating Fitness Functions Using Bonobo Optimizer for Optimal Control of PV-HESS Systems: A Comparative Analysis
2023 Second International Conference on Energy Transition and Security (ICETS)(2023)
摘要
This paper enhances PV-HESS performance and control under uncertain PV generation and load demand. It compares single (SOO) and multi-objective optimization (MOO) approaches, identifies winning fitness functions for each variable, and evaluates the Bnobo optimizer's (BO) performance. System performance is assessed using KPIs for voltage, Battery/Supercapacitor currents, and load demand. The results demonstrate that the MOO algorithm achieves, on average, a 15% reduction in DC Bus Voltage Error compared to the SOO algorithm when using RMSE as the fitness function. The SOO algorithm outperforms in terms of current errors, achieving a reduction of 20% with MAE as the winning fitness function. The MOO algorithm achieves, on average, a 10% reduction in Supercapacitor Current Error using MSE as the winning fitness function. However, the SOO algorithm generally outperforms in terms of Load Supply Mismatch, reducing the error by 12% with RMSE as the winning fitness function. These findings provide insights into the selection of the most effective fitness functions for optimizing specific variables under different objective functions and highlight the effectiveness of the BO. The study contributes to the optimization of PV-HESS control systems, enhancing stability, reliability, and performance in the presence of renewable energy sources and variable loads.
更多查看译文
关键词
PV-HESS,single-objective optimization,multi-objective optimization,fitness functions,Bonobo optimizer
AI 理解论文
溯源树
样例
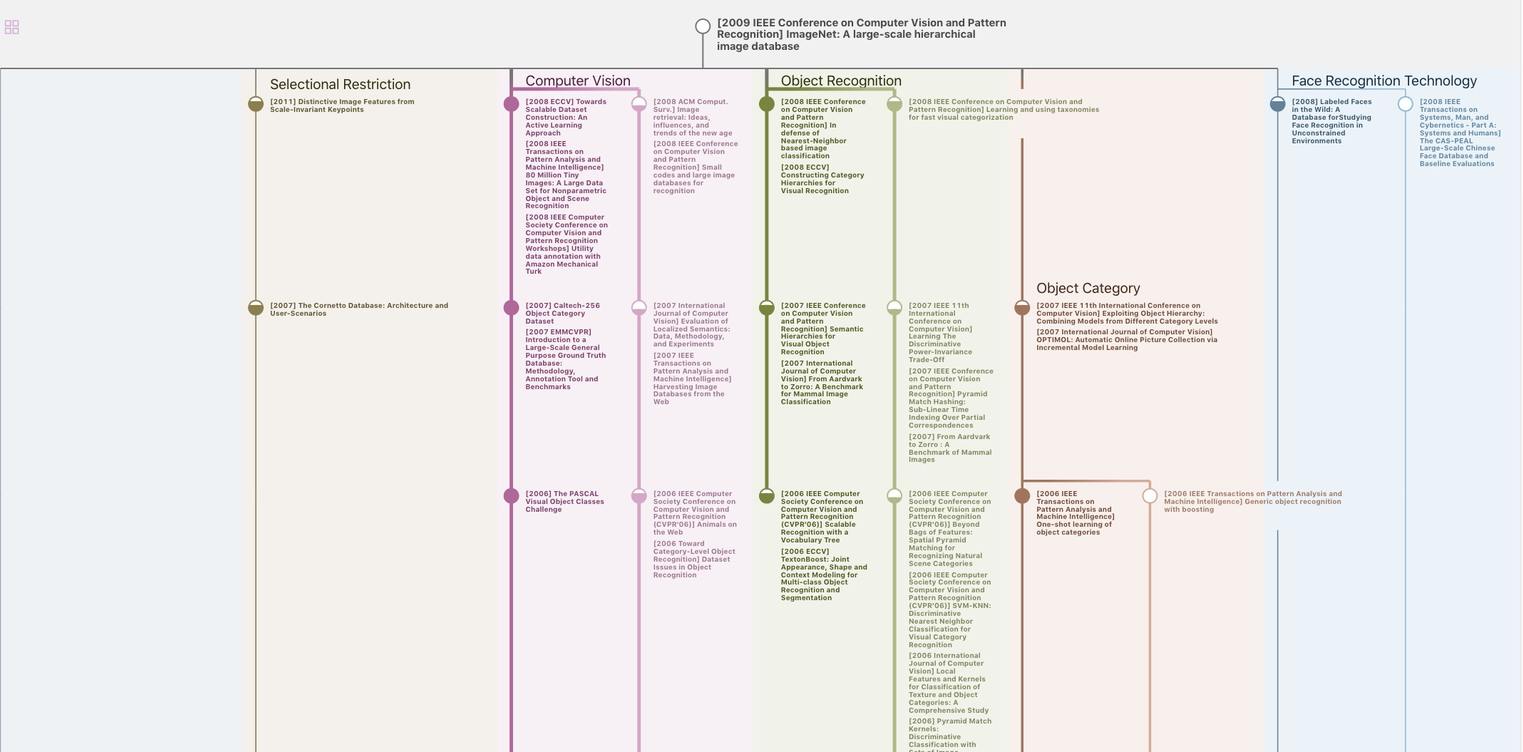
生成溯源树,研究论文发展脉络
Chat Paper
正在生成论文摘要