Causal Discovery by Continuous Optimization with Conditional Independence Constraint: Methodology and Performance
23RD IEEE INTERNATIONAL CONFERENCE ON DATA MINING, ICDM 2023(2023)
摘要
Discovering causal relationships from observational data is a challenging topic in artificial intelligence. Recent works formulate causal discovery as a continuous optimization problem with a differentiable acyclic constraint. Although these methods have achieved considerable performance improvement, they have two drawbacks: 1) they require a relatively large number of training samples; and 2) their performance will substantially deteriorate when facing heterogeneous noise. To address these problems, we first propose a low-order conditional independence (CI) constraint for the continuous optimization problem, and then design a soft version of the constraint by transforming it to a regularization term in the loss function of the continuous optimization problem. We show the convergence of continuous optimization with our constraint under some mild conditions, and the consistency of causal structure learning with the CI regularization. Extensive experiments on both synthetic and realworld datasets show that with our CI constraint or regularization, existing continuous optimization methods can achieve considerable performance improvement of causal discovery, especially when sample size is small.
更多查看译文
关键词
Causal discovery,continuous optimization,conditional,independence.
AI 理解论文
溯源树
样例
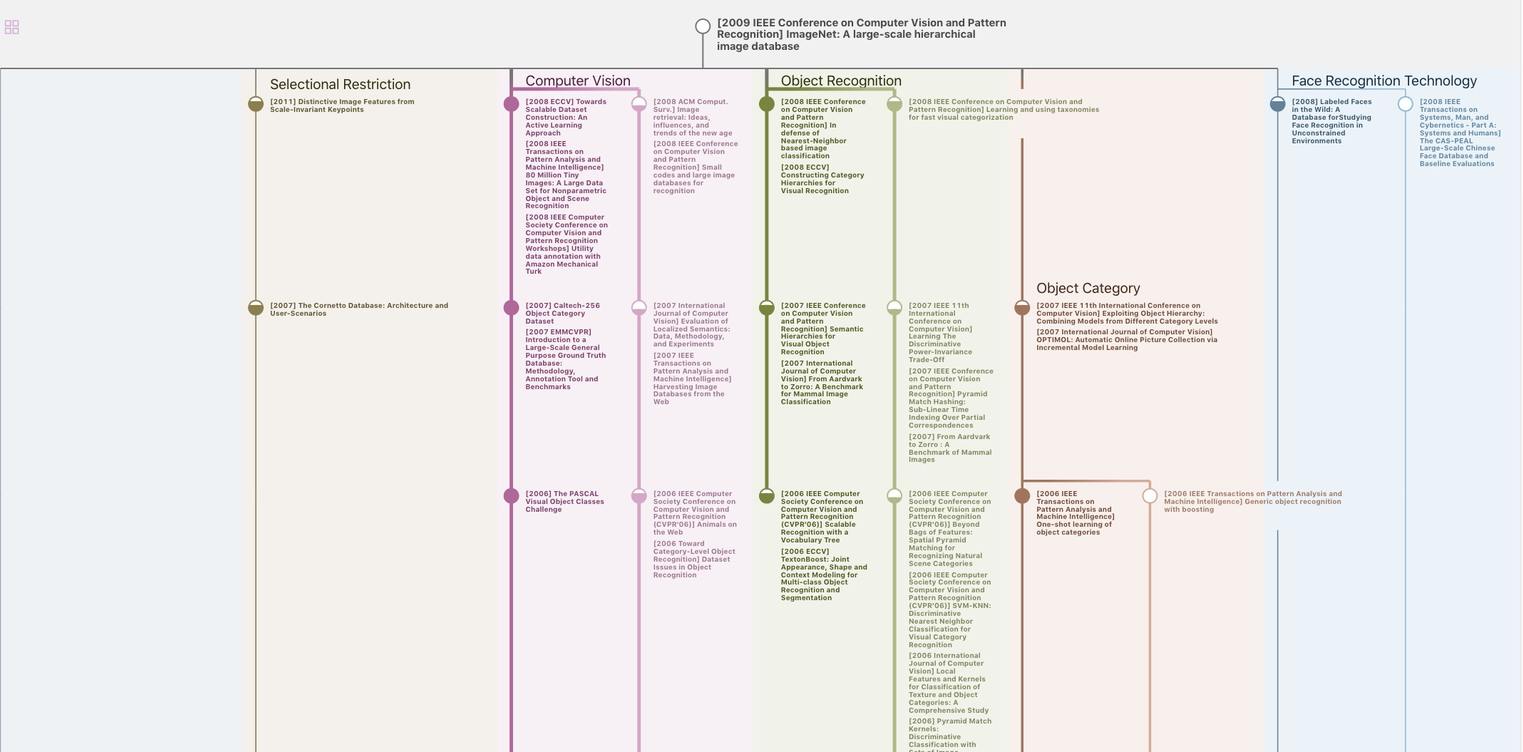
生成溯源树,研究论文发展脉络
Chat Paper
正在生成论文摘要