Multi-Robot Adaptive Sampling based on Mixture of Experts Approach to Modeling Non-Stationary Spatial Fields.
2023 International Symposium on Multi-Robot and Multi-Agent Systems (MRS)(2023)
摘要
This paper presents an adaptive sampling strategy for a team of robots to model non-stationary spatial fields – i.e., fields with uneven variations – in large environments, with a desired predictive accuracy. Modeling non-stationary heterogeneous fields is essential for many applications, like monitoring air quality or contamination level in lakes. Mainstream adaptive sampling strategies assume stationarity of the environmental phenomenon and use a single model to explain such fields, resulting in inaccurate characterization of unique localized variations. In this paper, we model a non-stationary field as a collection of (infinite, in theory) non-overlapping layers of stationary homogeneous subfields. This approach allows for modeling non-stationary fields using a mixture of experts, where each expert is assigned a particular homogeneous subregion to map. This approach decomposes the environment into smaller homogeneous regions, which allows real-time modeling of large environments. We design a data-driven approach to adaptively identify each stationary layer and define its relationship to other layers. We model the relationship between various subregions as a network of experts – the rapidly-sampling adaptive graph. Each robot incrementally builds its own sub-network of experts, which is used to determine where to sample. Several experiments in realistic simulation demonstrate competitive accuracy and sampling efficiency compared to other state-of-the-art methods.
更多查看译文
关键词
Adaptive Sampling,Mixture Of Experts,Non-stationary Field,Prediction Accuracy,Network Of Experts,Swarm Robotics,Root Mean Square Error,Mean Square Error,Accurate Estimation,Variance In The Data,Active Learning,Data In Order,Mutual Information,Gaussian Process,Kriging,Regional Environment,Acquisition Function,Voronoi Diagram,Robot Operating System,Minimum Accuracy,Autonomous Surface Vehicles,Gaussian Process Regression Model,Upper Confidence Bound,Path Information,Closest Measurements,Neighboring Data
AI 理解论文
溯源树
样例
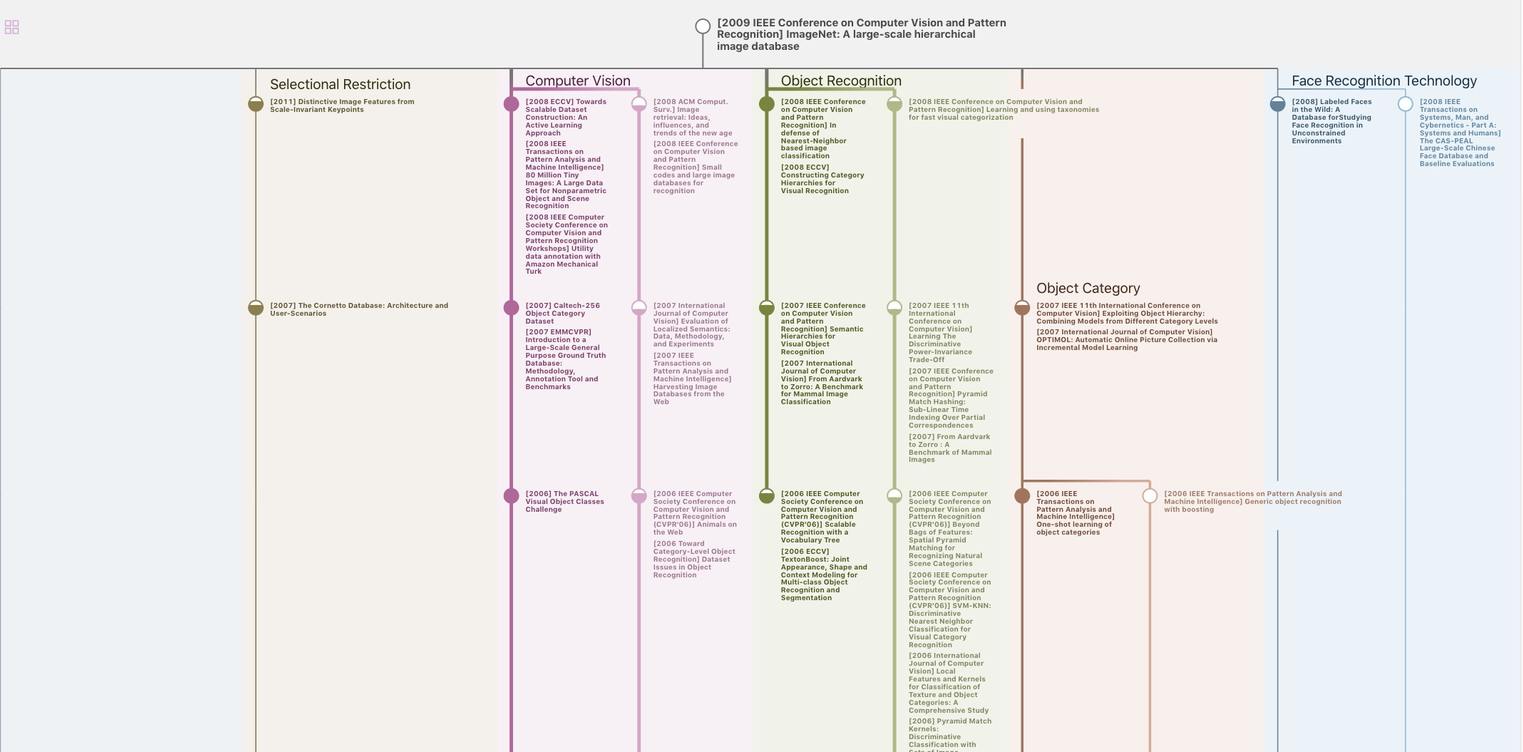
生成溯源树,研究论文发展脉络
Chat Paper
正在生成论文摘要