Data Quality Aware Hierarchical Federated Reinforcement Learning Framework for Dynamic Treatment Regimes
23RD IEEE INTERNATIONAL CONFERENCE ON DATA MINING, ICDM 2023(2023)
摘要
Due to the privacy concerns and rigorous data regulations, dynamic treatment regimes across hospitals have become increasingly difficult. Fortunately, federated learning provides a distributed learning framework to collaboratively train the model without sharing the highly sensitive electronic health record (UM) data with others. However, there exist two main challenges, namely data quality discrepancy, and heterogeneous data distribution, which seriously restrict the development of federated dynamic treatment regimes. To this end, we develop a global data quality aware dynamic treatment regime based on hierarchical federated reinforcement learning across different hospitals. In detail, we first quantify data quality in EHR using immediate health status changes, which are then utilized as rewards to encourage the high -quality treatment actions in the offline actor-critic reinforcement learning model. Within the parameter server, an online reinforcement learning based clustering scheme is proposed to capture the internal similarities to augment the positive knowledge transfer of high-quality hospitals while neglecting the heterogeneity. Extensive experiments are conducted on two diverse real-world datasets to show the advantages of DFR-DTR over state-of-the-art baselines.
更多查看译文
关键词
Dynamic treatment regimes,Federated learning,Data quality,Heterogeneity
AI 理解论文
溯源树
样例
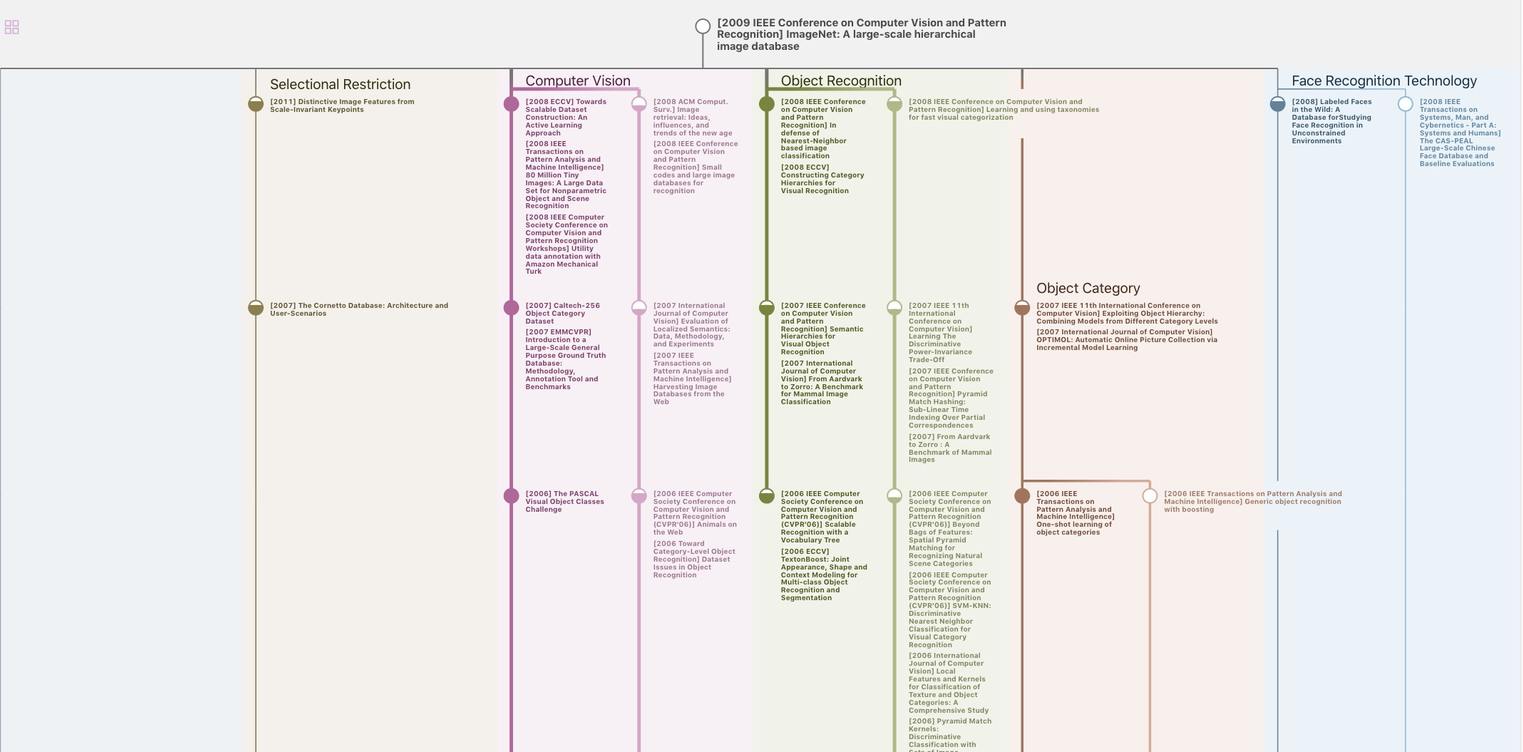
生成溯源树,研究论文发展脉络
Chat Paper
正在生成论文摘要