Enriched Representation Learning for Longitudinal Chest X-ray Analysis: A Novel Approach for Improved Disease Detection and Localization
23RD IEEE INTERNATIONAL CONFERENCE ON DATA MINING, ICDM 2023(2023)
摘要
Chest X-rays are commonly used for diagnosing and characterizing lung diseases, but the complex morphological patterns in radiographic appearances can challenge clinicians in making accurate diagnoses. To address this challenge, various learning methods have been developed for algorithm-aided disease detection and automated diagnosis. However, most existing methods fail to account for the heterogeneous variability in longitudinal imaging records and the presence of missing or inconsistent temporal data. In this paper, we propose a novel longitudinal learning framework that enriches inconsistent imaging data over sequential time points by leveraging two-dimensional principal component analysis and a robust adaptive loss function. We also derive an efficient solution algorithm that ensures both objective and sequence convergence for the non-convex optimization problem. Our experiments on the CheXpert dataset demonstrate improved performance in capturing indicative abnormalities in medical images and achieving satisfactory diagnoses. We believe that our method will be of significant interest to the research community working on medical image analysis.
更多查看译文
关键词
Longitudinal Learning,Representation Enrichment,Robust Learning
AI 理解论文
溯源树
样例
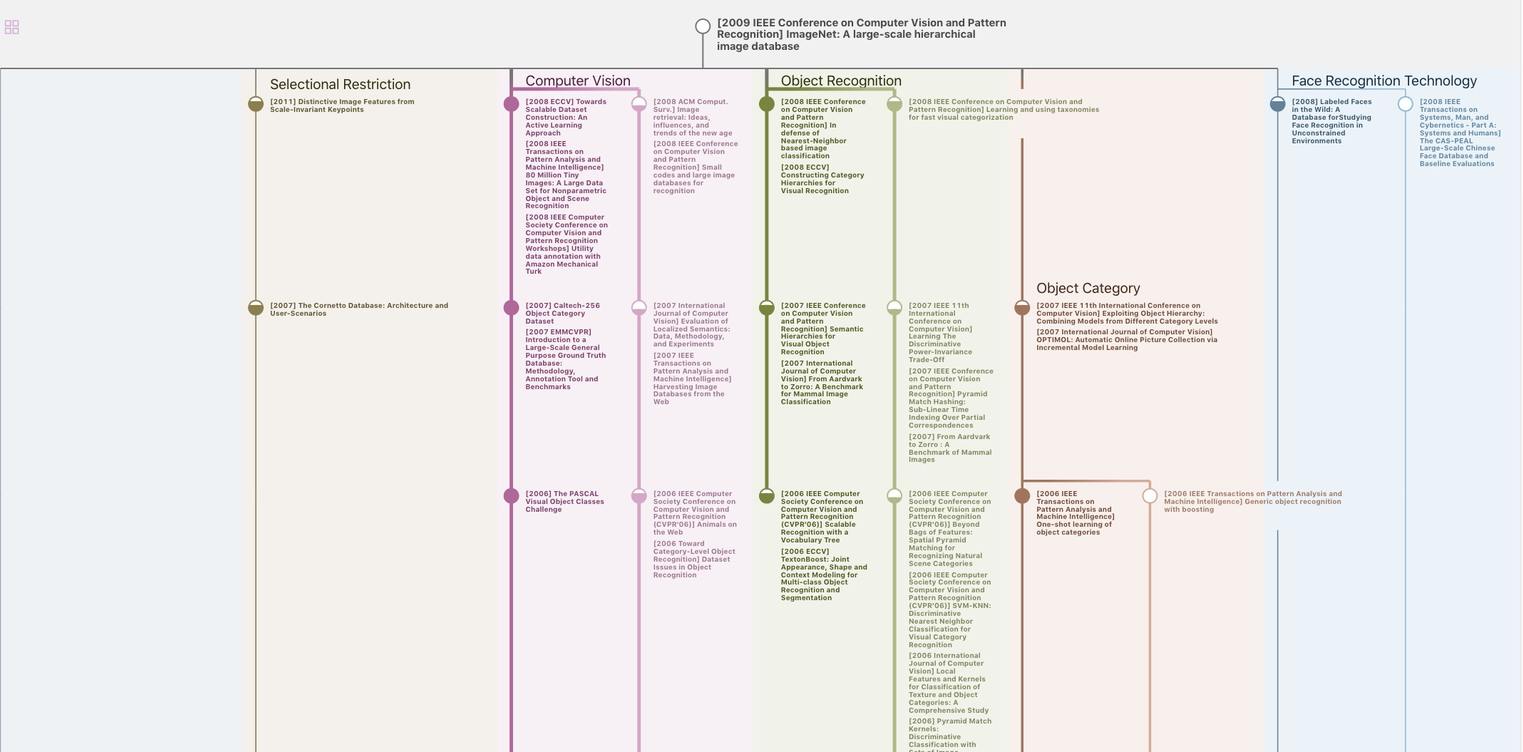
生成溯源树,研究论文发展脉络
Chat Paper
正在生成论文摘要