GAFNO: Gated Adaptive Fourier Neural Operator for Task-Agnostic Time Series Modeling
23RD IEEE INTERNATIONAL CONFERENCE ON DATA MINING, ICDM 2023(2023)
摘要
Time series data is ubiquitous in various domains, making it crucial for numerous research and practical applications. Previous methods have primarily focused on modeling local patterns or pairwise correlations, limiting their ability to capture global dependencies. This paper introduces a versatile time series backbone called Gated Adaptive Fourier Neural Operator (GAFNO), which efficiently performs global convolution in the Fourier domain, adapting FNO for time series analysis through a novel monotonic gating mechanism and adaptive weight sharing. With our proposed monotonic gate, GAFNO is allowed to adaptively filter high-frequency noise for different datasets, thereby improving flexibility and ensuring robustness. Based on the resolution-invariant nature inherited from the neural operator, we develop a learnable multi-scale architecture built upon GAFNO, enabling automatic integration of multi-scale results without the need for manually designed sampling. Additionally, we propose an iterative forecasting framework capable of predicting arbitrary horizons without requiring encoder modifications or extra decoders. Extensive experiments show that our proposed GAFNO consistently achieves overall state-of-the-art in five mainstream time series analysis tasks.
更多查看译文
关键词
time series analysis,operator learning,Fourier transform,multi-scale learning
AI 理解论文
溯源树
样例
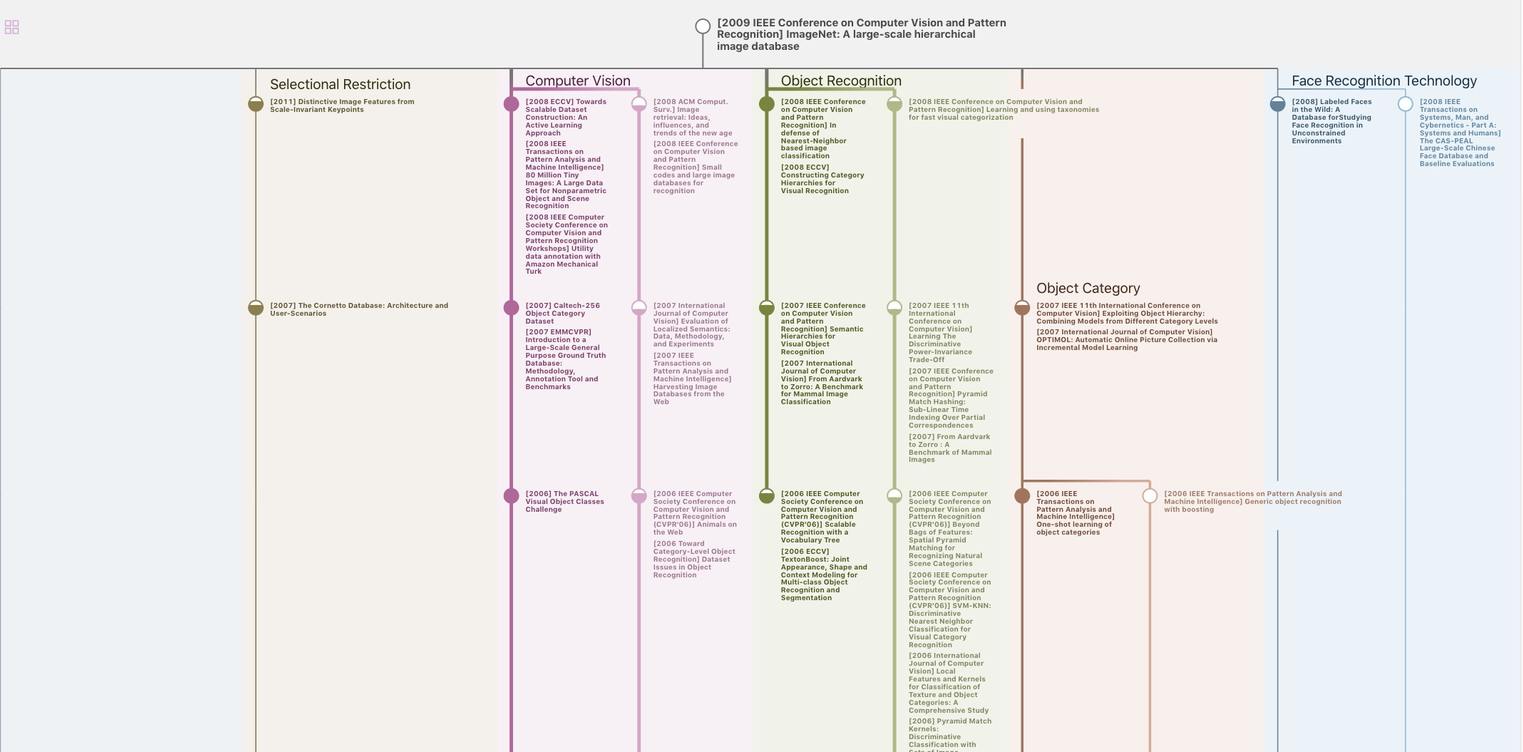
生成溯源树,研究论文发展脉络
Chat Paper
正在生成论文摘要