Unfairness in Distributed Graph Frameworks
23RD IEEE INTERNATIONAL CONFERENCE ON DATA MINING, ICDM 2023(2023)
Abstract
In the era of big data, distributed graph processing frameworks have become important in processing large-scale graph datasets. Such distributed frameworks exhibit major advantages with respect to scalability, and provide various ways to speed up sequential graph algorithms. However, the literature lacks an analysis on the fairness properties of such distributed algorithms. In this work, we analyze several important distributed frameworks and graph analysis algorithms with respect to their fairness properties. Across numerous real-world network datasets, we demonstrate that distributed algorithms often exhibit worse fairness performance as compared to their sequential counterparts. Moreover, we observe that this phenomenon is often strongly connected to the homophily of the graph dataset the tendency of nodes to connect to other nodes of the same class.
MoreTranslated text
Key words
n/a
AI Read Science
Must-Reading Tree
Example
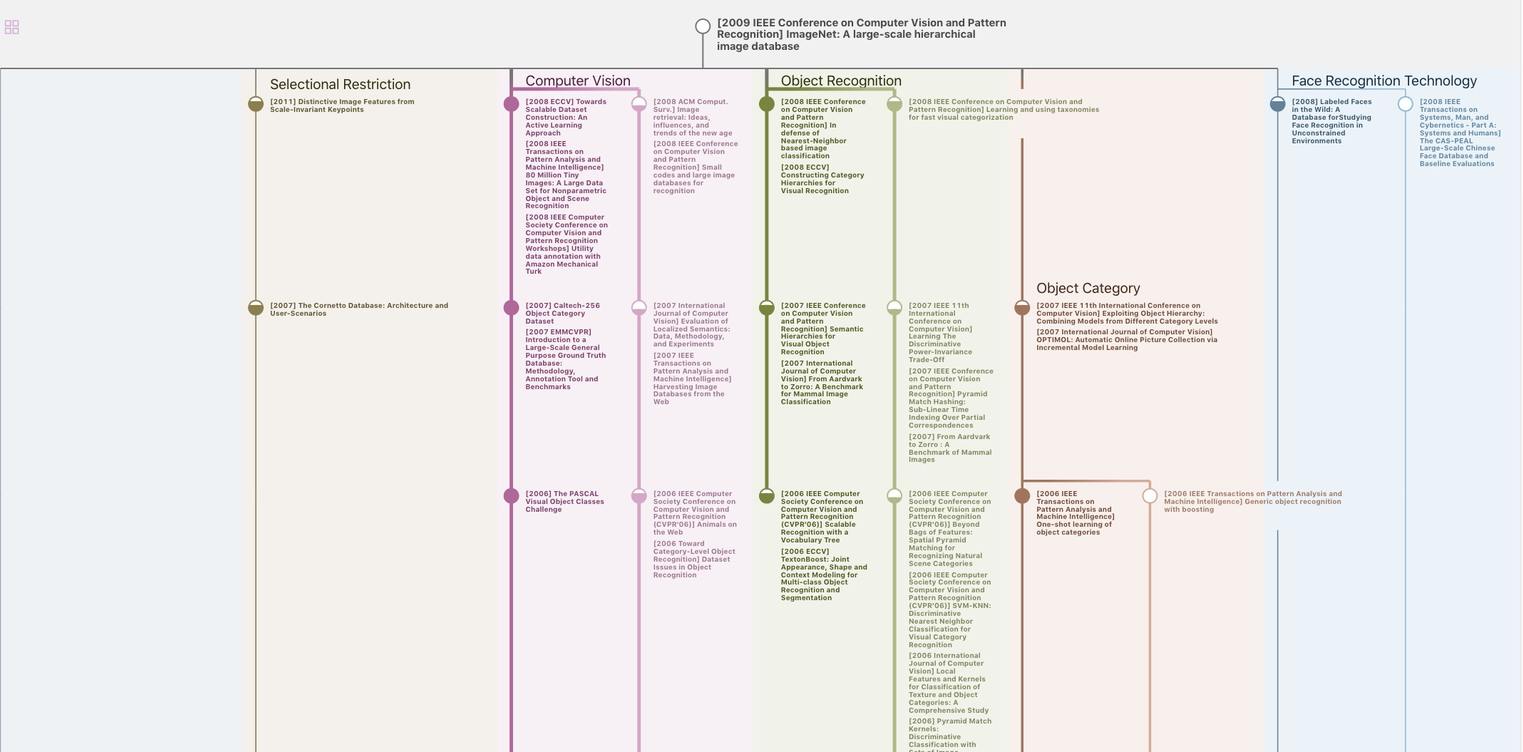
Generate MRT to find the research sequence of this paper
Chat Paper
Summary is being generated by the instructions you defined