Differentiable Cosmological Simulation with the Adjoint Method
ASTROPHYSICAL JOURNAL SUPPLEMENT SERIES(2024)
摘要
Rapid advances in deep learning have brought not only a myriad of powerful neural networks, but also breakthroughs that benefit established scientific research. In particular, automatic differentiation (AD) tools and computational accelerators like GPUs have facilitated forward modeling of the Universe with differentiable simulations. Based on analytic or automatic backpropagation, current differentiable cosmological simulations are limited by memory, and thus are subject to a trade-off between time and space/mass resolution, usually sacrificing both. We present a new approach free of such constraints, using the adjoint method and reverse time integration. It enables larger and more accurate forward modeling at the field level, and will improve gradient-based optimization and inference. We implement it in an open-source particle-mesh (PM) N-body library pmwd (PM with derivatives). Based on the powerful AD system JAX, pmwd is fully differentiable, and is highly performant on GPUs.
更多查看译文
关键词
Cosmology,Large-scale structure of the universe,N-body simulations,Astronomy software,Computational methods,Algorithms
AI 理解论文
溯源树
样例
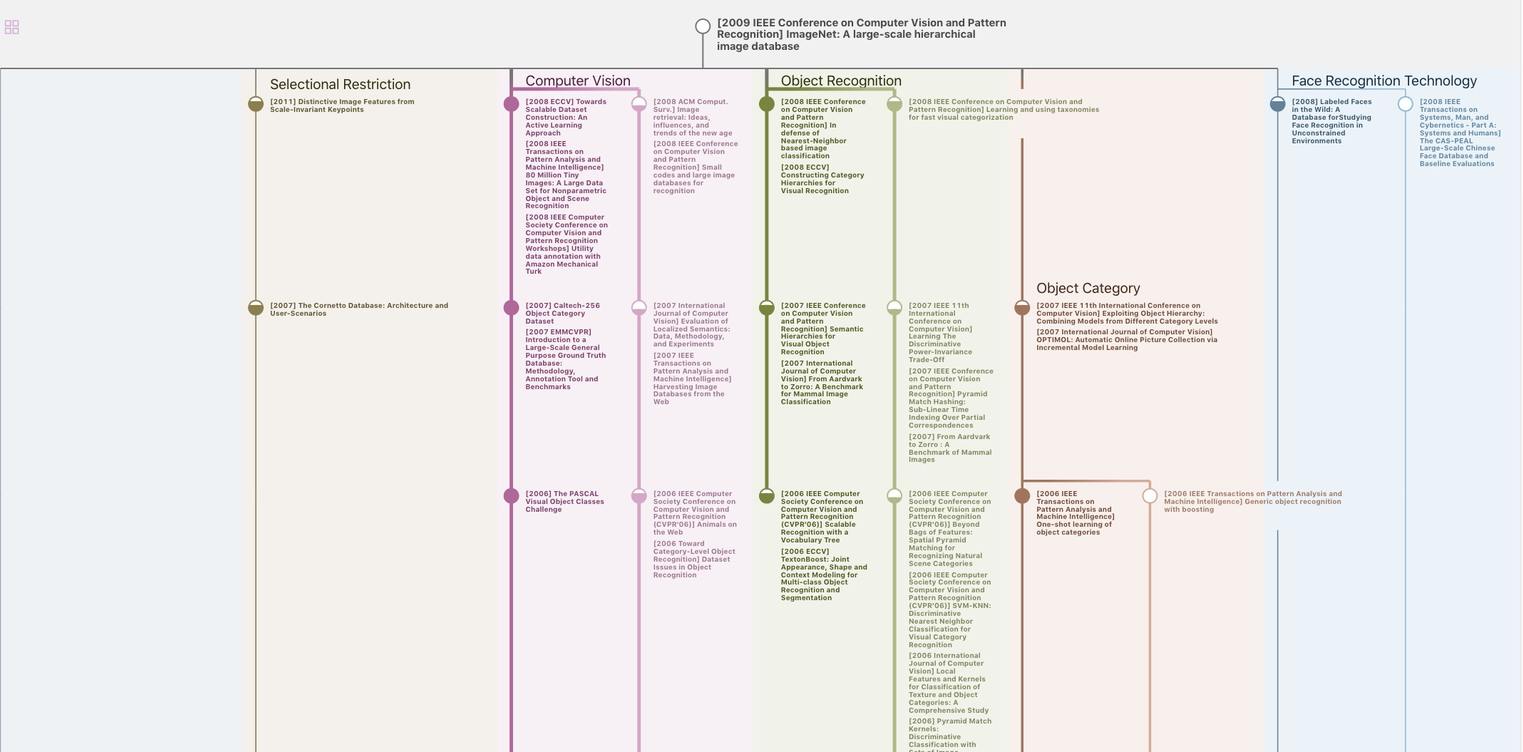
生成溯源树,研究论文发展脉络
Chat Paper
正在生成论文摘要