Machine Learning-Based Cardiovascular Disease Detection Using Optimal Feature Selection.
IEEE Access(2024)
摘要
Cardiovascular disease (CVD) is a prevalent and serious condition causing a significant global mortality rate. According to the World Health Organization (WHO), in 2022, CVD claimed the lives of approximately 19.1 million people, accounting for 33% of global fatalities. ECG is widely used for automatic detection of CVD using traditional machine learning; however, it is usually difficult to select optimal features. Addressing this issue, a scalable machine learning-based architecture is proposed for early CVD detection based optimal feature selection. This architecture aims to revolutionize healthcare by enabling timely diagnosis and treatment, reducing CVD-related fatalities. Comprising data collection, storage, and processing components, the system employs machine learning classifiers to predict patients’ heart conditions. Initially features are extracted from ECG signals then feature selection techniques like FCBF, MrMr, and relief, along with PSO-optimization are used to select optimal features. Extra Tree and Random Forest classifiers trained on the selected features have achieved notable performance rates with accuracy of 100% respectively. Furthermore, a comparison of the proposed method with state of the art on both small and large dataset is provided. The proposed system holds potential to revolutionize patient care and substantially lower CVD-related mortality, enhancing the quality of life for affected individuals. In summary, this architecture offers a promising solution to the pressing issue of CVD and paves the way for advanced healthcare systems.
更多查看译文
关键词
Cardiovascular disease,feature selection,optimization,machine learning
AI 理解论文
溯源树
样例
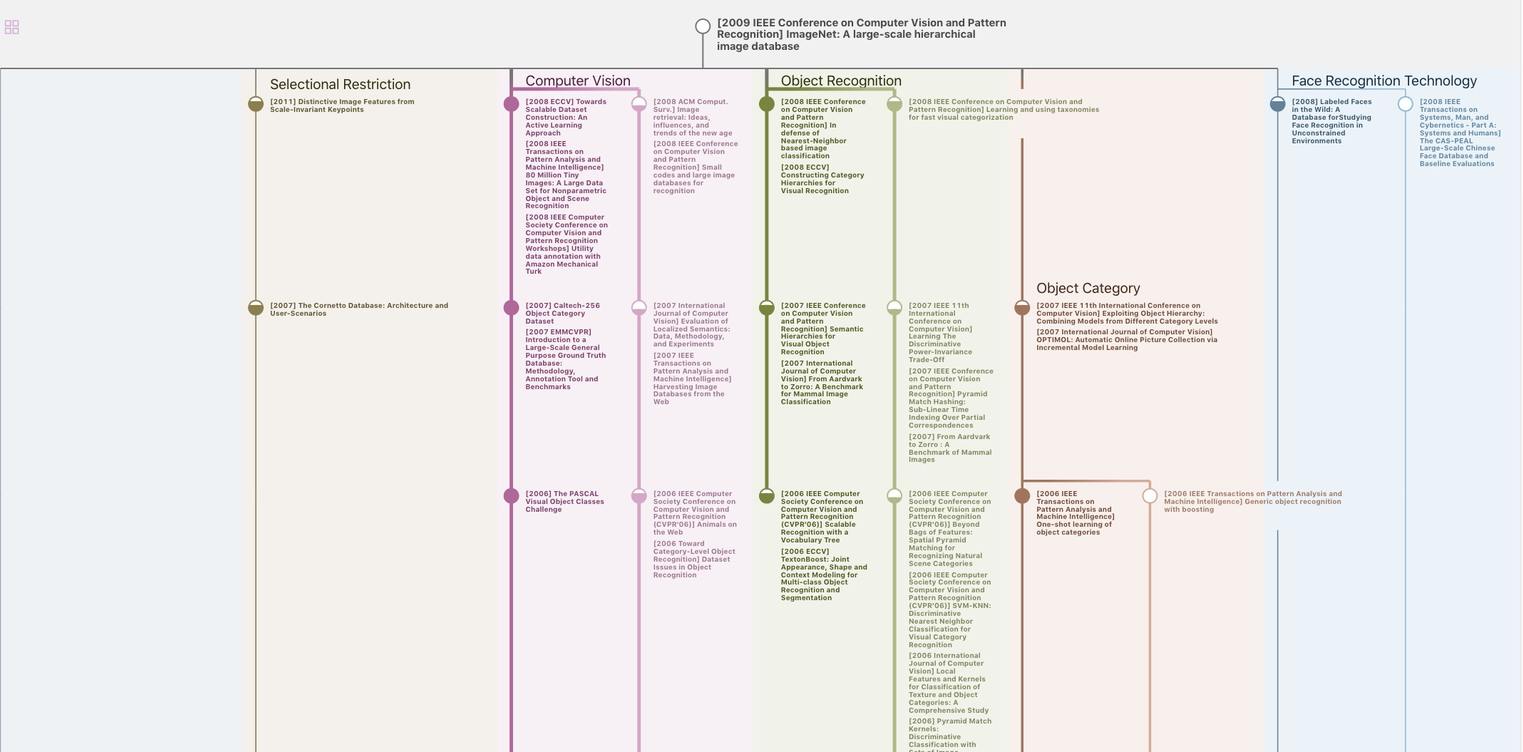
生成溯源树,研究论文发展脉络
Chat Paper
正在生成论文摘要