Deep Supervision by Gaussian Pseudo-label-based Morphological Attention for Abdominal Aorta Segmentation in Non-Contrast CTs
CoRR(2024)
摘要
The segmentation of the abdominal aorta in non-contrast CT images is a
non-trivial task for computer-assisted endovascular navigation, particularly in
scenarios where contrast agents are unsuitable. While state-of-the-art deep
learning segmentation models have been proposed recently for this task, they
are trained on manually annotated strong labels. However, the inherent
ambiguity in the boundary of the aorta in non-contrast CT may undermine the
reliability of strong labels, leading to potential overfitting risks. This
paper introduces a Gaussian-based pseudo label, integrated into conventional
deep learning models through deep supervision, to achieve Morphological
Attention (MA) enhancement. As the Gaussian pseudo label retains the
morphological features of the aorta without explicitly representing its
boundary distribution, we suggest that it preserves aortic morphology during
training while mitigating the negative impact of ambiguous boundaries, reducing
the risk of overfitting. It is introduced in various 2D/3D deep learning models
and validated on our local data set of 30 non-contrast CT volumes comprising
5749 CT slices. The results underscore the effectiveness of MA in preserving
the morphological characteristics of the aorta and addressing overfitting
concerns, thereby enhancing the performance of the models.
更多查看译文
AI 理解论文
溯源树
样例
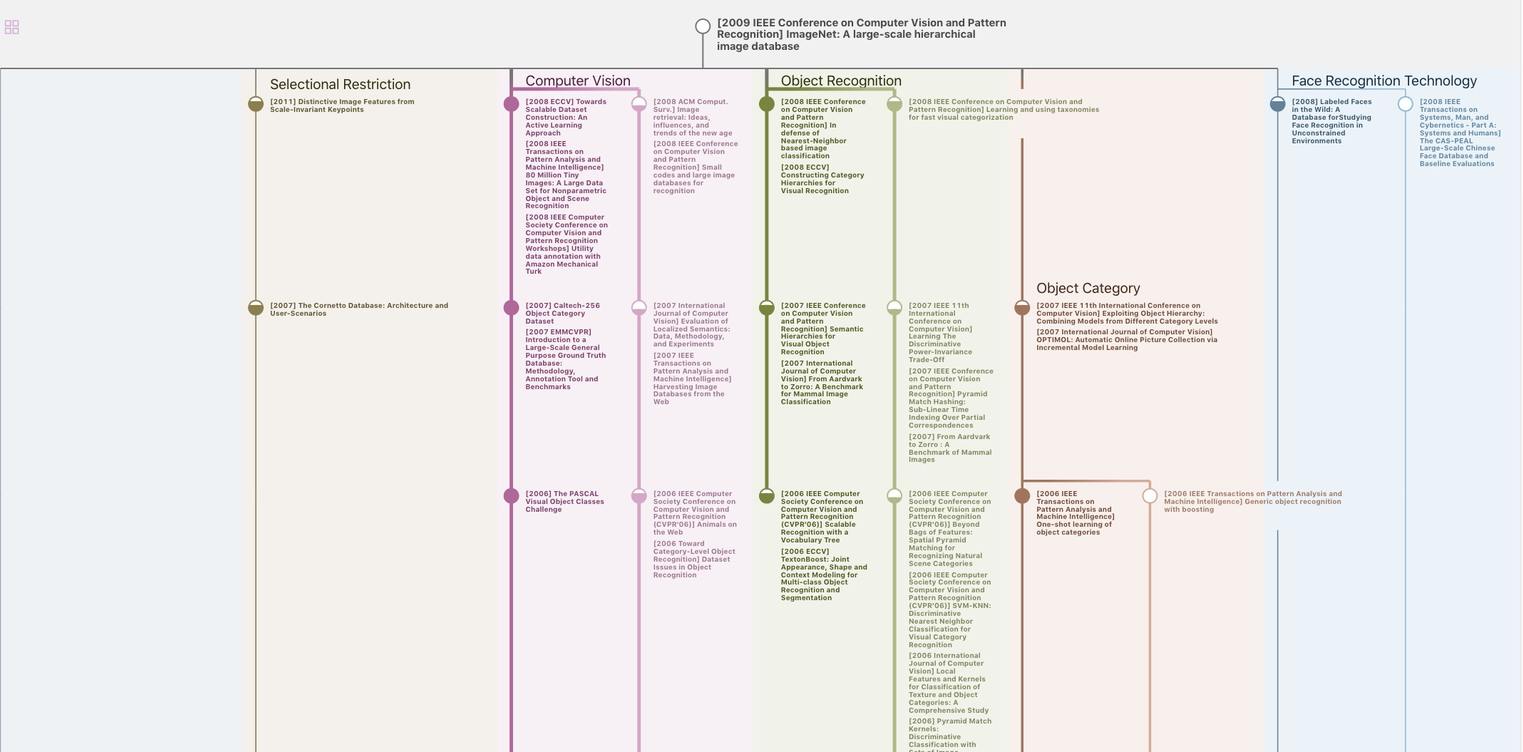
生成溯源树,研究论文发展脉络
Chat Paper
正在生成论文摘要