Bayes-Optimal Fair Classification with Linear Disparity Constraints via Pre-, In-, and Post-processing
CoRR(2024)
摘要
Machine learning algorithms may have disparate impacts on protected groups.
To address this, we develop methods for Bayes-optimal fair classification,
aiming to minimize classification error subject to given group fairness
constraints. We introduce the notion of linear disparity measures, which
are linear functions of a probabilistic classifier; and bilinear
disparity measures, which are also linear in the group-wise regression
functions. We show that several popular disparity measures – the deviations
from demographic parity, equality of opportunity, and predictive equality –
are bilinear.
We find the form of Bayes-optimal fair classifiers under a single linear
disparity measure, by uncovering a connection with the Neyman-Pearson lemma.
For bilinear disparity measures, Bayes-optimal fair classifiers become
group-wise thresholding rules. Our approach can also handle multiple fairness
constraints (such as equalized odds), and the common scenario when the
protected attribute cannot be used at the prediction phase.
Leveraging our theoretical results, we design methods that learn fair
Bayes-optimal classifiers under bilinear disparity constraints. Our methods
cover three popular approaches to fairness-aware classification, via
pre-processing (Fair Up- and Down-Sampling), in-processing (Fair Cost-Sensitive
Classification) and post-processing (a Fair Plug-In Rule). Our methods control
disparity directly while achieving near-optimal fairness-accuracy tradeoffs. We
show empirically that our methods compare favorably to existing algorithms.
更多查看译文
AI 理解论文
溯源树
样例
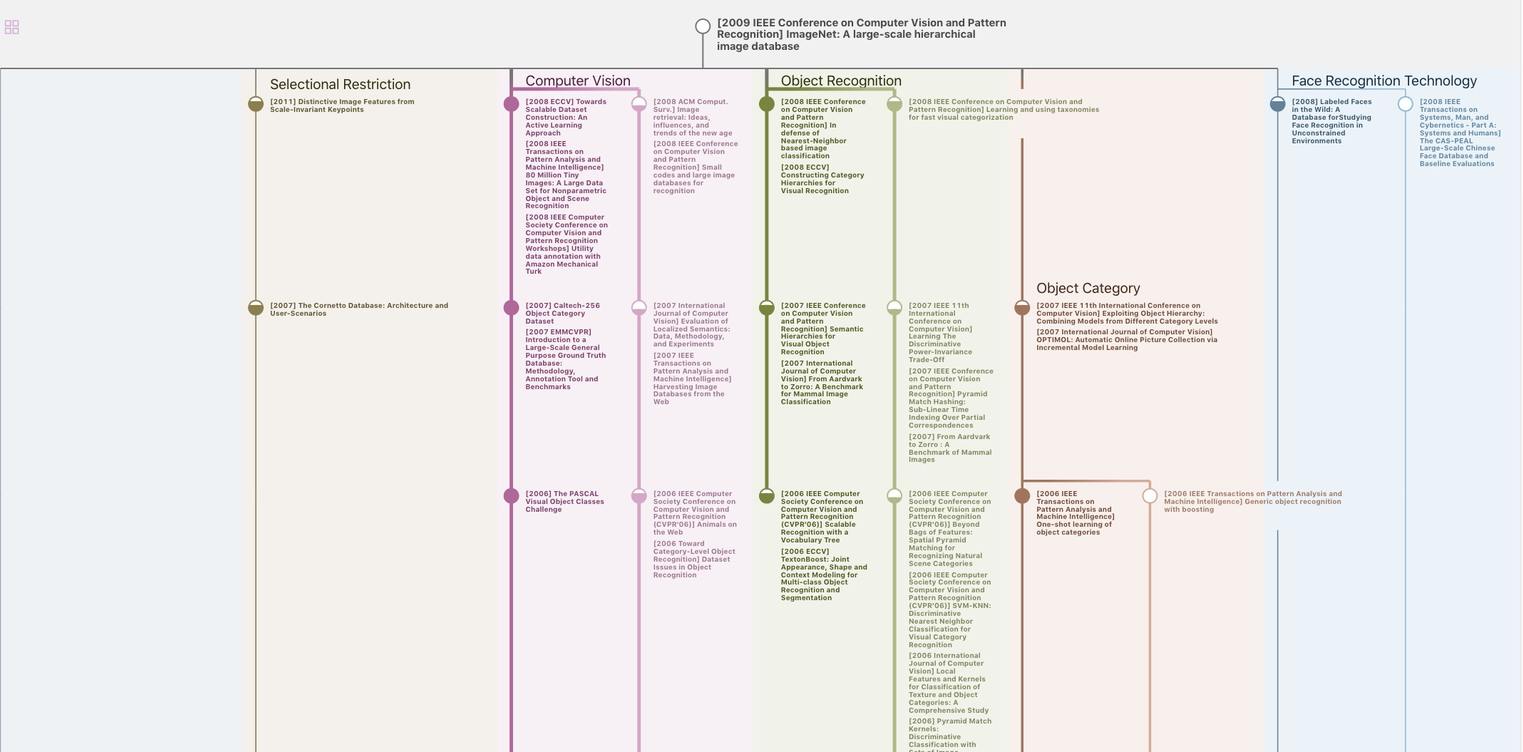
生成溯源树,研究论文发展脉络
Chat Paper
正在生成论文摘要