Aligner: Achieving Efficient Alignment through Weak-to-Strong Correction
CoRR(2024)
摘要
Efforts to align Large Language Models (LLMs) are mainly conducted via
Reinforcement Learning from Human Feedback (RLHF) methods. However, RLHF
encounters major challenges including training reward models, actor-critic
engineering, and importantly, it requires access to LLM parameters. Here we
introduce Aligner, a new efficient alignment paradigm that bypasses the whole
RLHF process by learning the correctional residuals between the aligned and the
unaligned answers. Our Aligner offers several key advantages. Firstly, it is an
autoregressive seq2seq model that is trained on the query-answer-correction
dataset via supervised learning; this offers a parameter-efficient alignment
solution with minimal resources. Secondly, the Aligner facilitates
weak-to-strong generalization; finetuning large pretrained models by Aligner's
supervisory signals demonstrates strong performance boost. Thirdly, Aligner
functions as a model-agnostic plug-and-play module, allowing for its direct
application on different open-source and API-based models. Remarkably,
Aligner-7B improves 11 different LLMs by 18
harmlessness on average (GPT-4 by 26.9
Llama2-70B with (weak) Aligner-7B's supervision, we can improve Llama2 by 8.2
in helpfulness and 61.6
.
更多查看译文
AI 理解论文
溯源树
样例
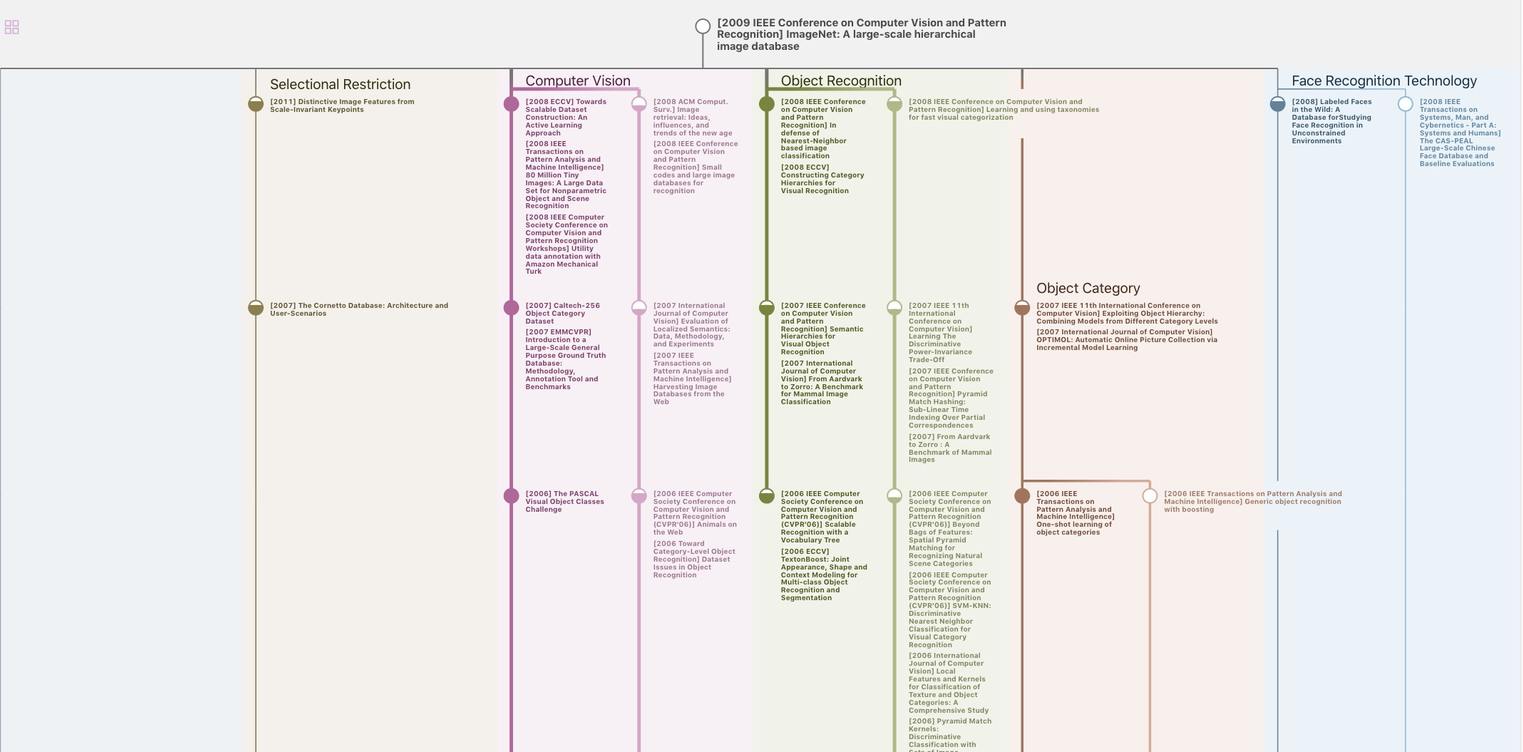
生成溯源树,研究论文发展脉络
Chat Paper
正在生成论文摘要