Dynamic Incremental Optimization for Best Subset Selection
CoRR(2024)
摘要
Best subset selection is considered the `gold standard' for many sparse
learning problems. A variety of optimization techniques have been proposed to
attack this non-smooth non-convex problem. In this paper, we investigate the
dual forms of a family of ℓ_0-regularized problems. An efficient
primal-dual algorithm is developed based on the primal and dual problem
structures. By leveraging the dual range estimation along with the incremental
strategy, our algorithm potentially reduces redundant computation and improves
the solutions of best subset selection. Theoretical analysis and experiments on
synthetic and real-world datasets validate the efficiency and statistical
properties of the proposed solutions.
更多查看译文
AI 理解论文
溯源树
样例
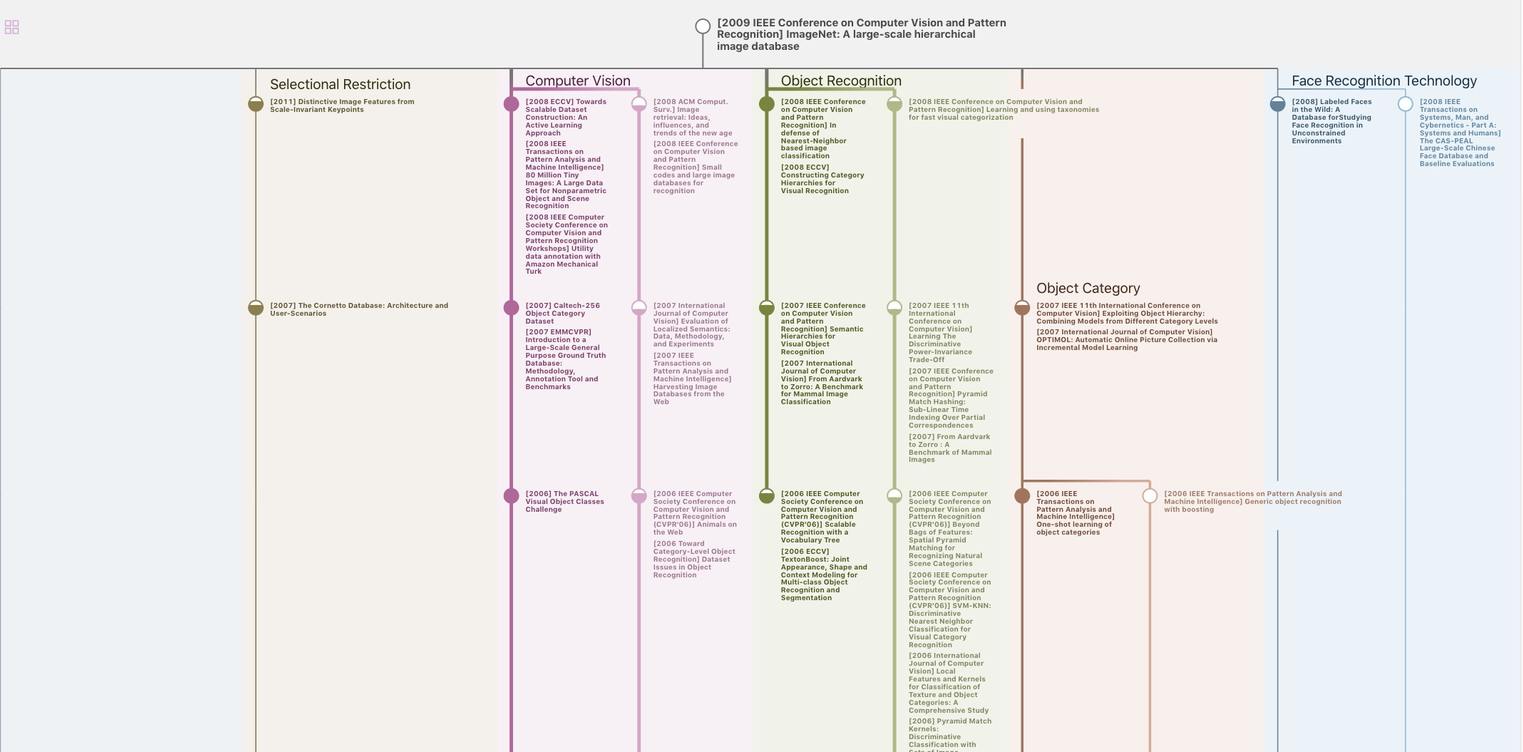
生成溯源树,研究论文发展脉络
Chat Paper
正在生成论文摘要