Your Diffusion Model is Secretly a Certifiably Robust Classifier
CoRR(2024)
Abstract
Diffusion models are recently employed as generative classifiers for robust
classification. However, a comprehensive theoretical understanding of the
robustness of diffusion classifiers is still lacking, leading us to question
whether they will be vulnerable to future stronger attacks. In this study, we
propose a new family of diffusion classifiers, named Noised Diffusion
Classifiers (NDCs), that possess state-of-the-art certified robustness.
Specifically, we generalize the diffusion classifiers to classify
Gaussian-corrupted data by deriving the evidence lower bounds (ELBOs) for these
distributions, approximating the likelihood using the ELBO, and calculating
classification probabilities via Bayes' theorem. We integrate these generalized
diffusion classifiers with randomized smoothing to construct smoothed
classifiers possessing non-constant Lipschitzness. Experimental results
demonstrate the superior certified robustness of our proposed NDCs. Notably, we
are the first to achieve 80%+ and 70%+ certified robustness on CIFAR-10 under
adversarial perturbations with ℓ_2 norm less than 0.25 and 0.5,
respectively, using a single off-the-shelf diffusion model without any
additional data.
MoreTranslated text
AI Read Science
Must-Reading Tree
Example
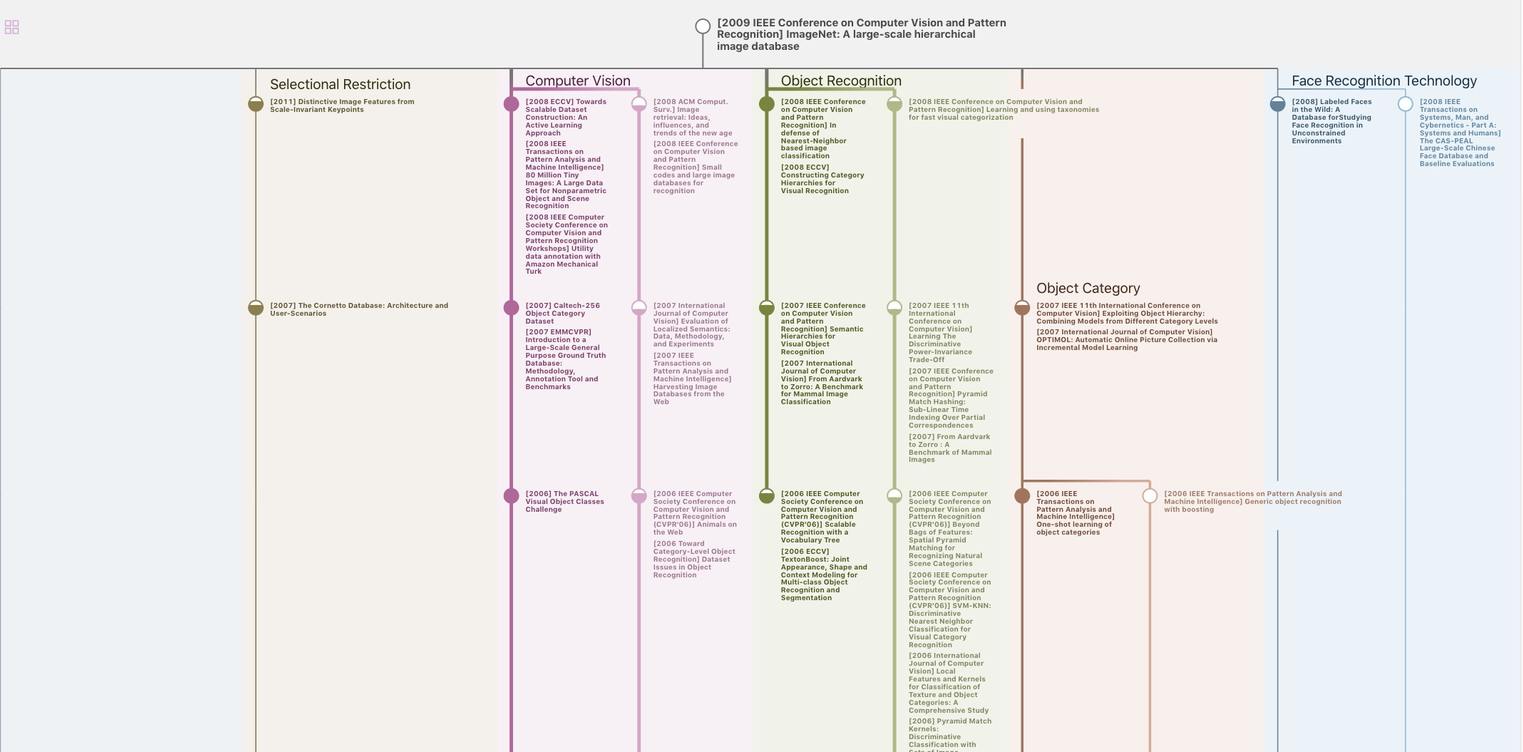
Generate MRT to find the research sequence of this paper
Chat Paper
Summary is being generated by the instructions you defined