Improving Diffusion Models for Inverse Problems Using Optimal Posterior Covariance
CoRR(2024)
摘要
Recent diffusion models provide a promising zero-shot solution to noisy
linear inverse problems without retraining for specific inverse problems. In
this paper, we propose the first unified interpretation for existing zero-shot
methods from the perspective of approximating the conditional posterior mean
for the reverse diffusion process of conditional sampling. We reveal that
recent methods are equivalent to making isotropic Gaussian approximations to
intractable posterior distributions over clean images given diffused noisy
images, with the only difference in the handcrafted design of isotropic
posterior covariances. Inspired by this finding, we propose a general
plug-and-play posterior covariance optimization based on maximum likelihood
estimation to improve recent methods. To achieve optimal posterior covariance
without retraining, we provide general solutions based on two approaches
specifically designed to leverage pre-trained models with and without reverse
covariances. Experimental results demonstrate that the proposed methods
significantly enhance the overall performance or robustness to hyperparameters
of recent methods. Code is available at
https://github.com/xypeng9903/k-diffusion-inverse-problems
更多查看译文
AI 理解论文
溯源树
样例
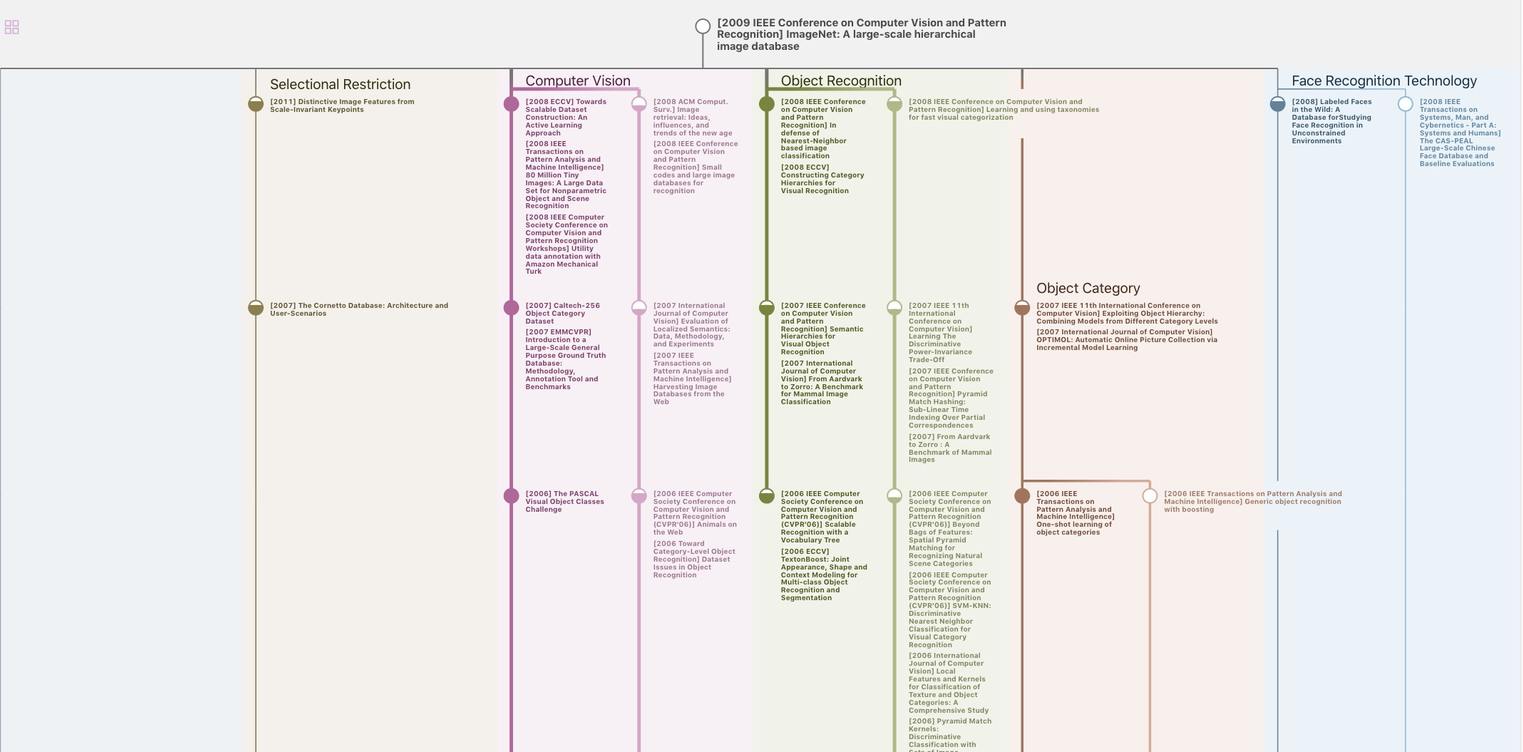
生成溯源树,研究论文发展脉络
Chat Paper
正在生成论文摘要