User Intent Recognition and Satisfaction with Large Language Models: A User Study with ChatGPT
CoRR(2024)
摘要
The rapid evolution of large language models such as GPT-4 Turbo represents
an impactful paradigm shift in digital interaction and content engagement.
While these models encode vast amounts of human-generated knowledge and excel
in processing diverse data types, recent research shows that they often face
the challenge of accurately responding to specific user intents, leading to
increased user dissatisfaction. Based on a fine-grained intent taxonomy and
intent-based prompt reformulations, we analyze (1) the quality of intent
recognition and (2) user satisfaction with answers from intent-based prompt
reformulations for two recent ChatGPT models, GPT-3.5 Turbo and GPT-4 Turbo.
The results reveal that GPT-4 outperforms GPT-3.5 on the recognition of common
intents, but is conversely often outperformed by GPT-3.5 on the recognition of
less frequent intents. Moreover, whenever the user intent is correctly
recognized, while users are more satisfied with the answers to intent-based
reformulations of GPT 4 compared to GPT-3.5, they tend to be more satisfied
with the answers of the models to their original prompts compared to the
reformulated ones. Finally, the study indicates that users can quickly learn to
formulate their prompts more effectively, once they are shown possible
reformulation templates.
更多查看译文
AI 理解论文
溯源树
样例
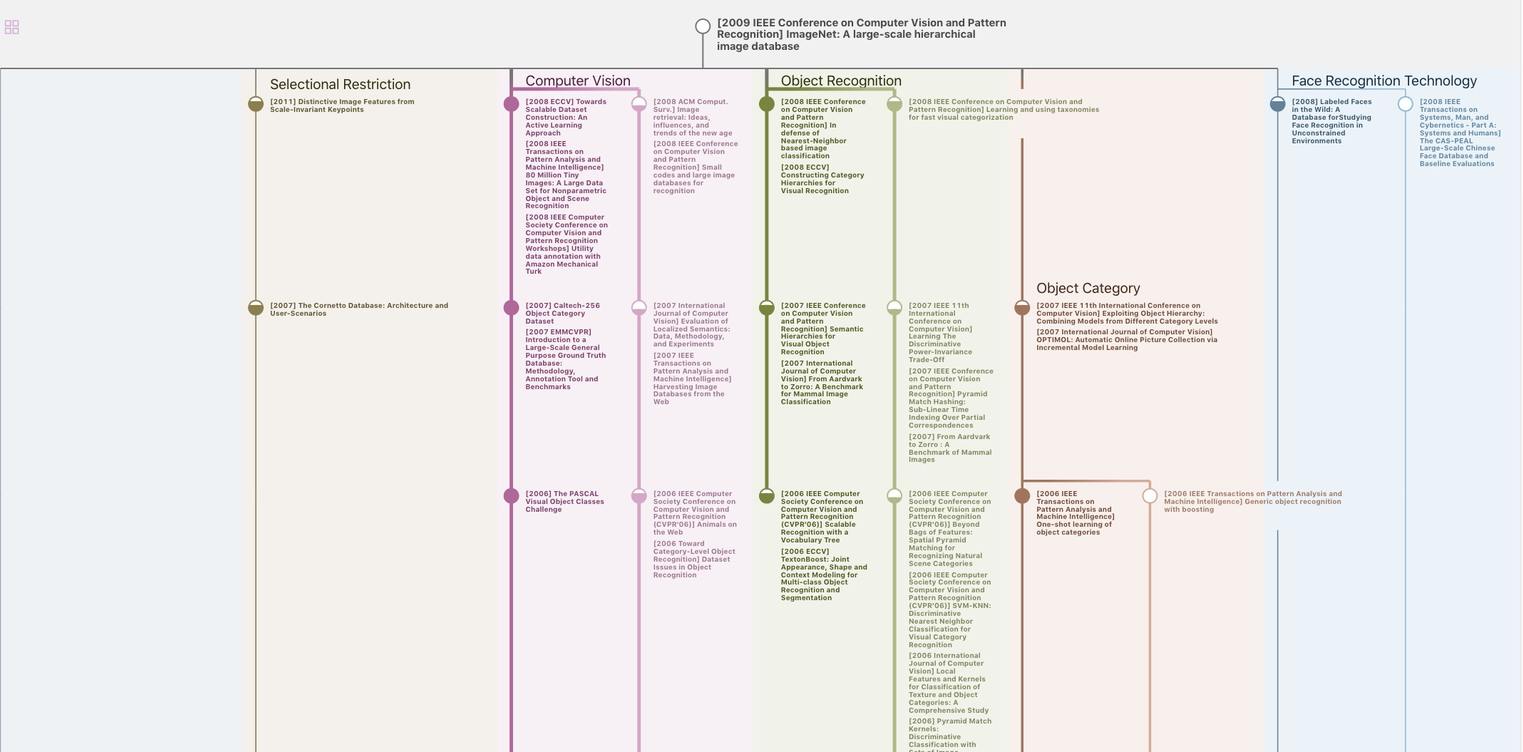
生成溯源树,研究论文发展脉络
Chat Paper
正在生成论文摘要