Déjà Vu Memorization in Vision-Language Models
CoRR(2024)
摘要
Vision-Language Models (VLMs) have emerged as the state-of-the-art
representation learning solution, with myriads of downstream applications such
as image classification, retrieval and generation. A natural question is
whether these models memorize their training data, which also has implications
for generalization. We propose a new method for measuring memorization in VLMs,
which we call déjà vu memorization. For VLMs trained on image-caption
pairs, we show that the model indeed retains information about individual
objects in the training images beyond what can be inferred from correlations or
the image caption. We evaluate déjà vu memorization at both sample and
population level, and show that it is significant for OpenCLIP trained on as
many as 50M image-caption pairs. Finally, we show that text randomization
considerably mitigates memorization while only moderately impacting the model's
downstream task performance.
更多查看译文
AI 理解论文
溯源树
样例
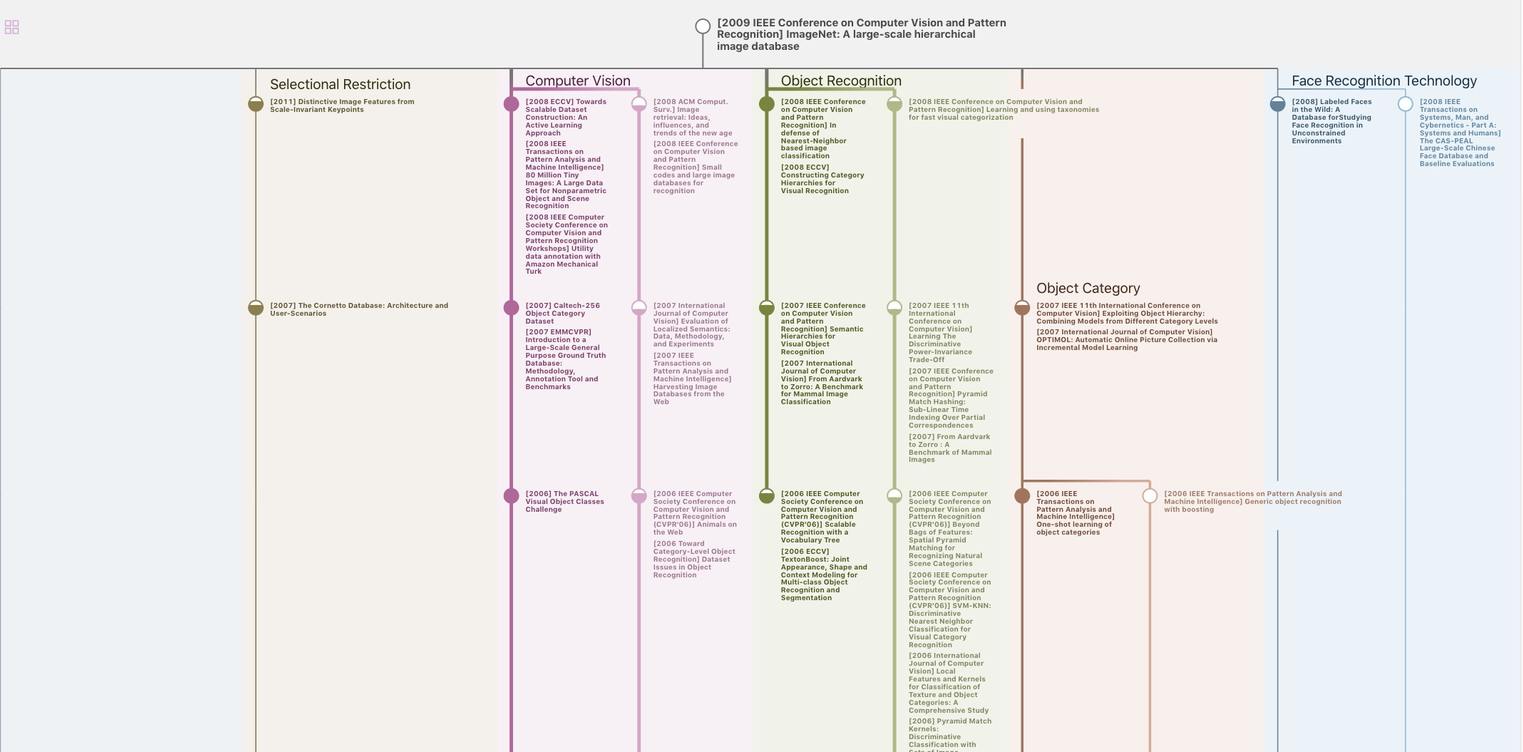
生成溯源树,研究论文发展脉络
Chat Paper
正在生成论文摘要