GliDe with a CaPE: A Low-Hassle Method to Accelerate Speculative Decoding
CoRR(2024)
摘要
Speculative decoding is a relatively new decoding framework that leverages
small and efficient draft models to reduce the latency of LLMs. In this study,
we introduce GliDe and CaPE, two low-hassle modifications to vanilla
speculative decoding to further improve the decoding speed of a frozen LLM.
Specifically, GliDe is a modified draft model architecture that reuses the
cached keys and values from the target LLM, while CaPE is a proposal expansion
method that uses the draft model's confidence scores to help select additional
candidate tokens for verification. Extensive experiments on different
benchmarks demonstrate that our proposed GliDe draft model significantly
reduces the expected decoding latency. Additional evaluation using walltime
reveals that GliDe can accelerate Vicuna models up to 2.17x and further extend
the improvement to 2.61x with CaPE. We will release our code, data, and the
trained draft models.
更多查看译文
AI 理解论文
溯源树
样例
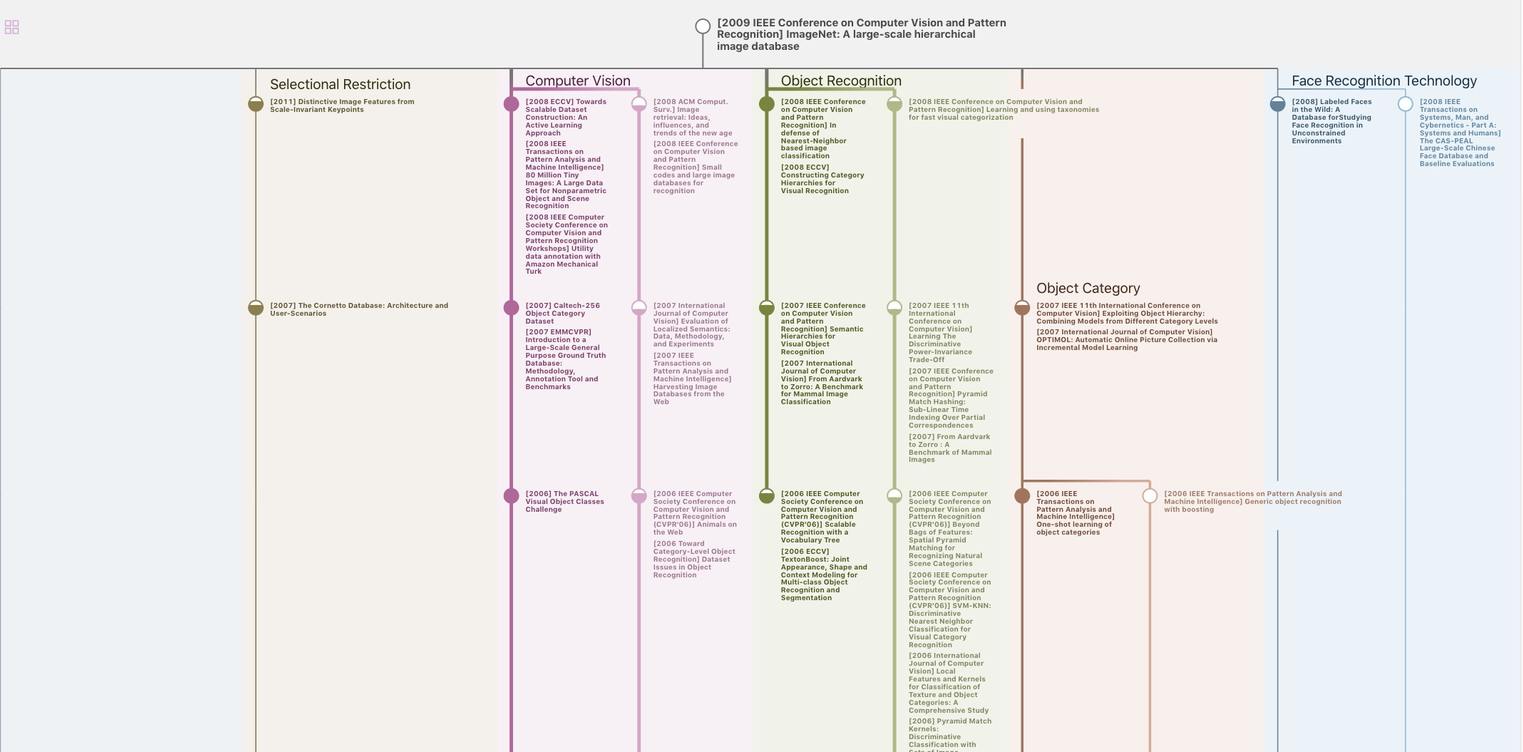
生成溯源树,研究论文发展脉络
Chat Paper
正在生成论文摘要