A Plug-in Tiny AI Module for Intelligent and Selective Sensor Data Transmission
CoRR(2024)
Abstract
Applications in the Internet of Things (IoT) utilize machine learning to
analyze sensor-generated data. However, a major challenge lies in the lack of
targeted intelligence in current sensing systems, leading to vast data
generation and increased computational and communication costs. To address this
challenge, we propose a novel sensing module to equip sensing frameworks with
intelligent data transmission capabilities by integrating a highly efficient
machine learning model placed near the sensor. This model provides prompt
feedback for the sensing system to transmit only valuable data while discarding
irrelevant information by regulating the frequency of data transmission. The
near-sensor model is quantized and optimized for real-time sensor control. To
enhance the framework's performance, the training process is customized and a
"lazy" sensor deactivation strategy utilizing temporal information is
introduced. The suggested method is orthogonal to other IoT frameworks and can
be considered as a plugin for selective data transmission. The framework is
implemented, encompassing both software and hardware components. The
experiments demonstrate that the framework utilizing the suggested module
achieves over 85
with negligible impact on performance. This methodology has the potential to
significantly reduce data output from sensors, benefiting a wide range of IoT
applications.
MoreTranslated text
AI Read Science
Must-Reading Tree
Example
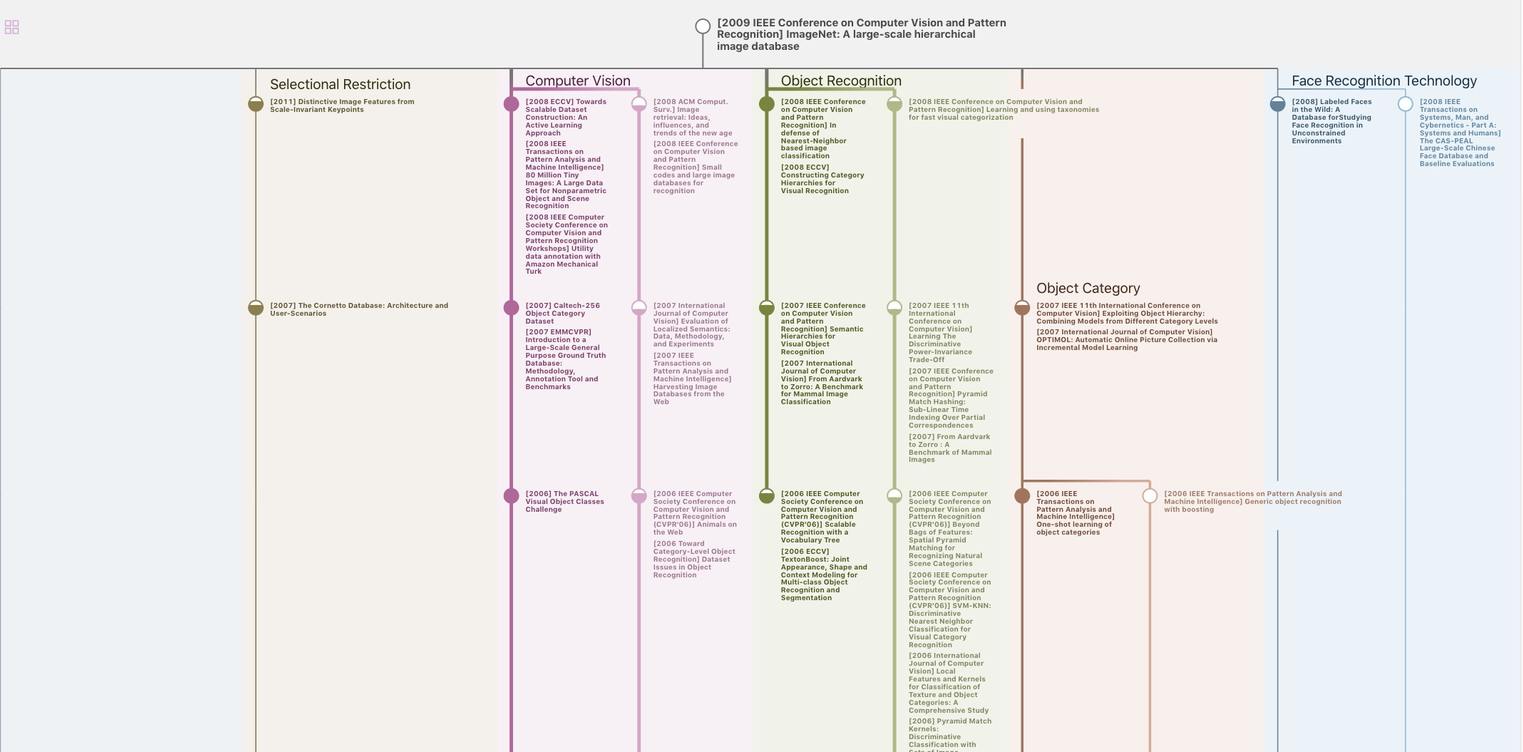
Generate MRT to find the research sequence of this paper
Chat Paper
Summary is being generated by the instructions you defined