A Survey of Constraint Formulations in Safe Reinforcement Learning
CoRR(2024)
摘要
Ensuring safety is critical when applying reinforcement learning (RL) to
real-world problems. Consequently, safe RL emerges as a fundamental and
powerful paradigm for safely optimizing an agent's policy from experimental
data. A popular safe RL approach is based on a constrained criterion, which
solves the problem of maximizing expected cumulative reward under safety
constraints. Though there has been recently a surge of such attempts to achieve
safety in RL, a systematic understanding of the field is difficult due to 1)
the diversity of constraint representations and 2) little discussion of their
interrelations. To address this knowledge gap, we provide a comprehensive
review of representative constraint formulations, along with a curated
selection of algorithms specifically designed for each formulation.
Furthermore, we elucidate the theoretical underpinnings that reveal the
mathematical mutual relations among common problem formulations. We conclude
with a discussion of the current state and future directions of safe
reinforcement learning research.
更多查看译文
AI 理解论文
溯源树
样例
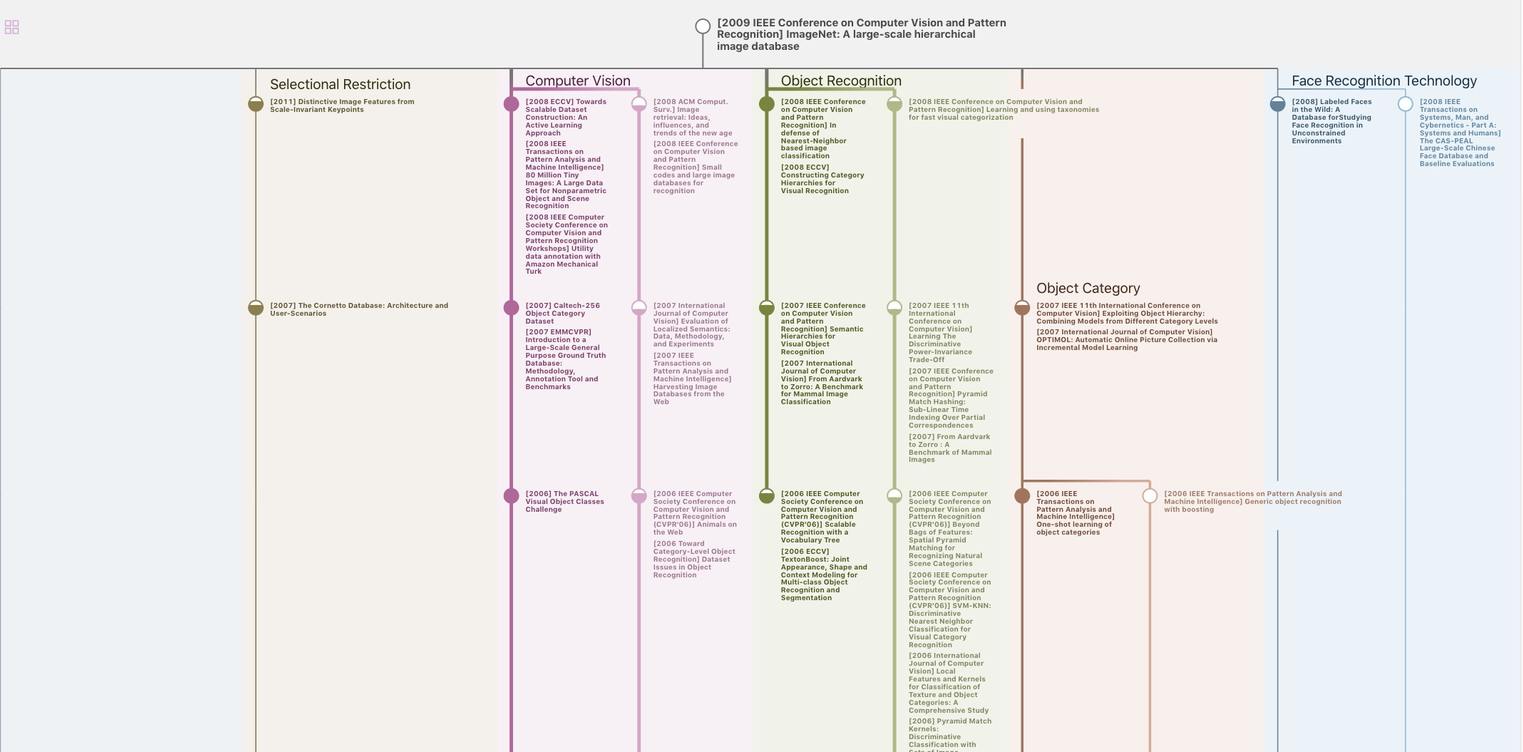
生成溯源树,研究论文发展脉络
Chat Paper
正在生成论文摘要