Value-Aided Conditional Supervised Learning for Offline RL
CoRR(2024)
摘要
Offline reinforcement learning (RL) has seen notable advancements through
return-conditioned supervised learning (RCSL) and value-based methods, yet each
approach comes with its own set of practical challenges. Addressing these, we
propose Value-Aided Conditional Supervised Learning (VCS), a method that
effectively synergizes the stability of RCSL with the stitching ability of
value-based methods. Based on the Neural Tangent Kernel analysis to discern
instances where value function may not lead to stable stitching, VCS injects
the value aid into the RCSL's loss function dynamically according to the
trajectory return. Our empirical studies reveal that VCS not only significantly
outperforms both RCSL and value-based methods but also consistently achieves,
or often surpasses, the highest trajectory returns across diverse offline RL
benchmarks. This breakthrough in VCS paves new paths in offline RL, pushing the
limits of what can be achieved and fostering further innovations.
更多查看译文
AI 理解论文
溯源树
样例
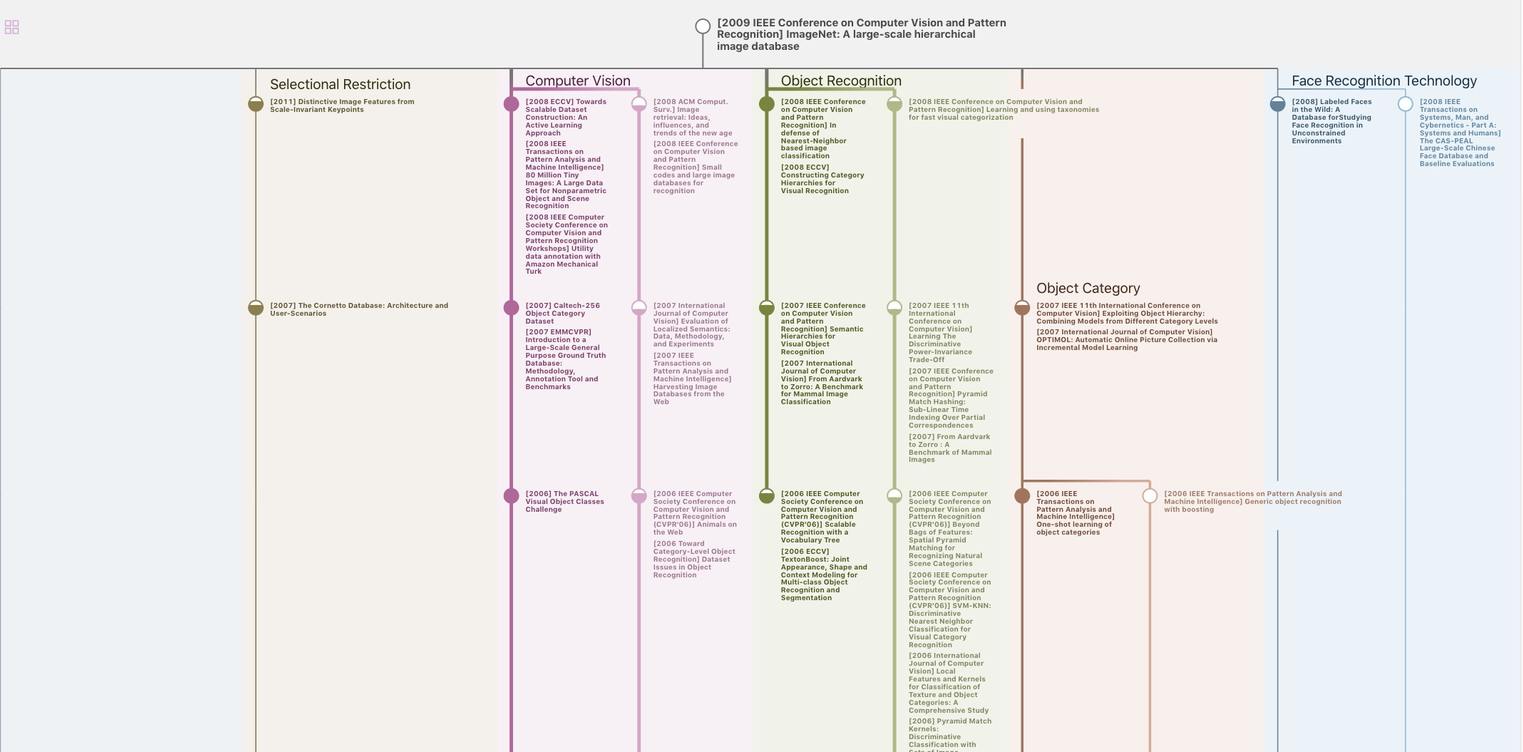
生成溯源树,研究论文发展脉络
Chat Paper
正在生成论文摘要