Outlier Accommodation for GNSS Precise Point Positioning using Risk-Averse State Estimation
CoRR(2024)
摘要
In the realm of intelligent transportation systems, reliable and precise
absolute Connected Automated Vehicles (CAV) positioning is necessary. Global
Navigation Satellite Systems (GNSS) provides the foundation for absolute
positioning. Recently enhanced Precise Point Positioning (PPP) GNSS, now
offering corrections for multi-GNSS on a global scale, offers the potential for
PPP GNSS to achieve the accuracy suitable for real-time CAV applications.
However, in obstructed sky conditions GNSS signals are often affected by
outliers; therefore, addressing outliers is crucial. In multi-GNSS
applications, there are many more measurements available than are required to
meet the specification, if outlier measurements can be avoided; therefore,
measurement selection is important. The recently developed Risk-Averse
Performance-Specified (RAPS) state estimation optimally selects measurements to
minimize outlier risk while meeting a positive semi-definite constraint on
performance; at present, the existing solution methods are not suitable for
real-time computation and have not been demonstrated using challenging
real-world data or in Real-time PPP (RT-PPP) applications. This article makes
contributions in a few directions. First, it uses a diagonal performance
specification, which reduces computational costs relative to the positive
semidefinite constraint. Second, this article considers multi-GNSS RT-PPP
applications. Third, the experiments use real-world GNSS data collected in
challenging environments. The RT-PPP experimental results show that among the
compared methods: all achieve comparable performance in open-sky conditions,
and all exceed the Society of Automotive Engineers (SAE) specification;
however, in challenging environments, the diagonal RAPS approach shows
improvement of 6-19
lowest outlier risk.
更多查看译文
AI 理解论文
溯源树
样例
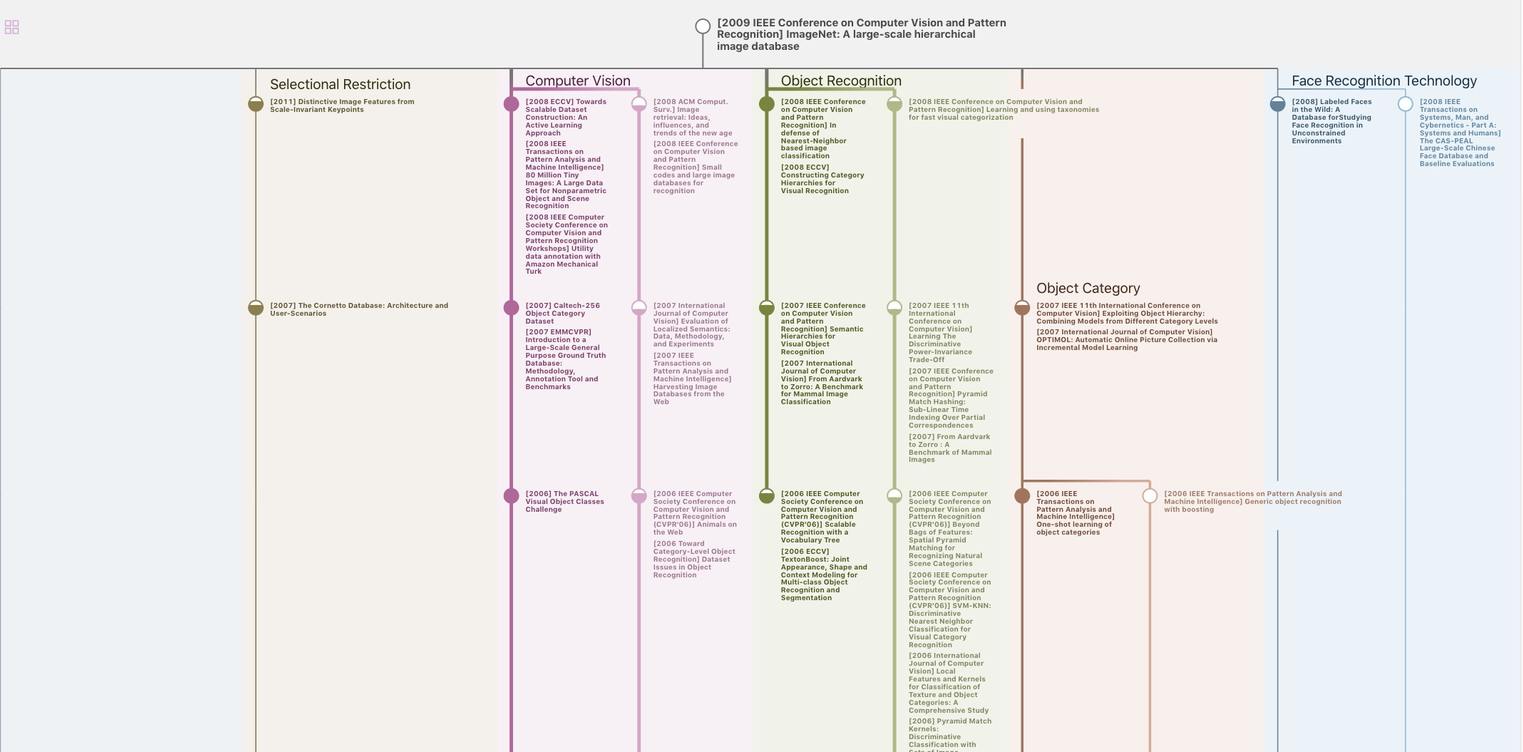
生成溯源树,研究论文发展脉络
Chat Paper
正在生成论文摘要