Simulator-Free Visual Domain Randomization via Video Games
CoRR(2024)
Abstract
Domain randomization is an effective computer vision technique for improving
transferability of vision models across visually distinct domains exhibiting
similar content. Existing approaches, however, rely extensively on tweaking
complex and specialized simulation engines that are difficult to construct,
subsequently affecting their feasibility and scalability. This paper introduces
BehAVE, a video understanding framework that uniquely leverages the plethora of
existing commercial video games for domain randomization, without requiring
access to their simulation engines. Under BehAVE (1) the inherent rich visual
diversity of video games acts as the source of randomization and (2) player
behavior – represented semantically via textual descriptions of actions –
guides the *alignment* of videos with similar content. We test BehAVE on 25
games of the first-person shooter (FPS) genre across various video and text
foundation models and we report its robustness for domain randomization. BehAVE
successfully aligns player behavioral patterns and is able to zero-shot
transfer them to multiple unseen FPS games when trained on just one FPS game.
In a more challenging setting, BehAVE manages to improve the zero-shot
transferability of foundation models to unseen FPS games (up to 22
trained on a game of a different genre (Minecraft). Code and dataset can be
found at https://github.com/nrasajski/BehAVE.
MoreTranslated text
AI Read Science
Must-Reading Tree
Example
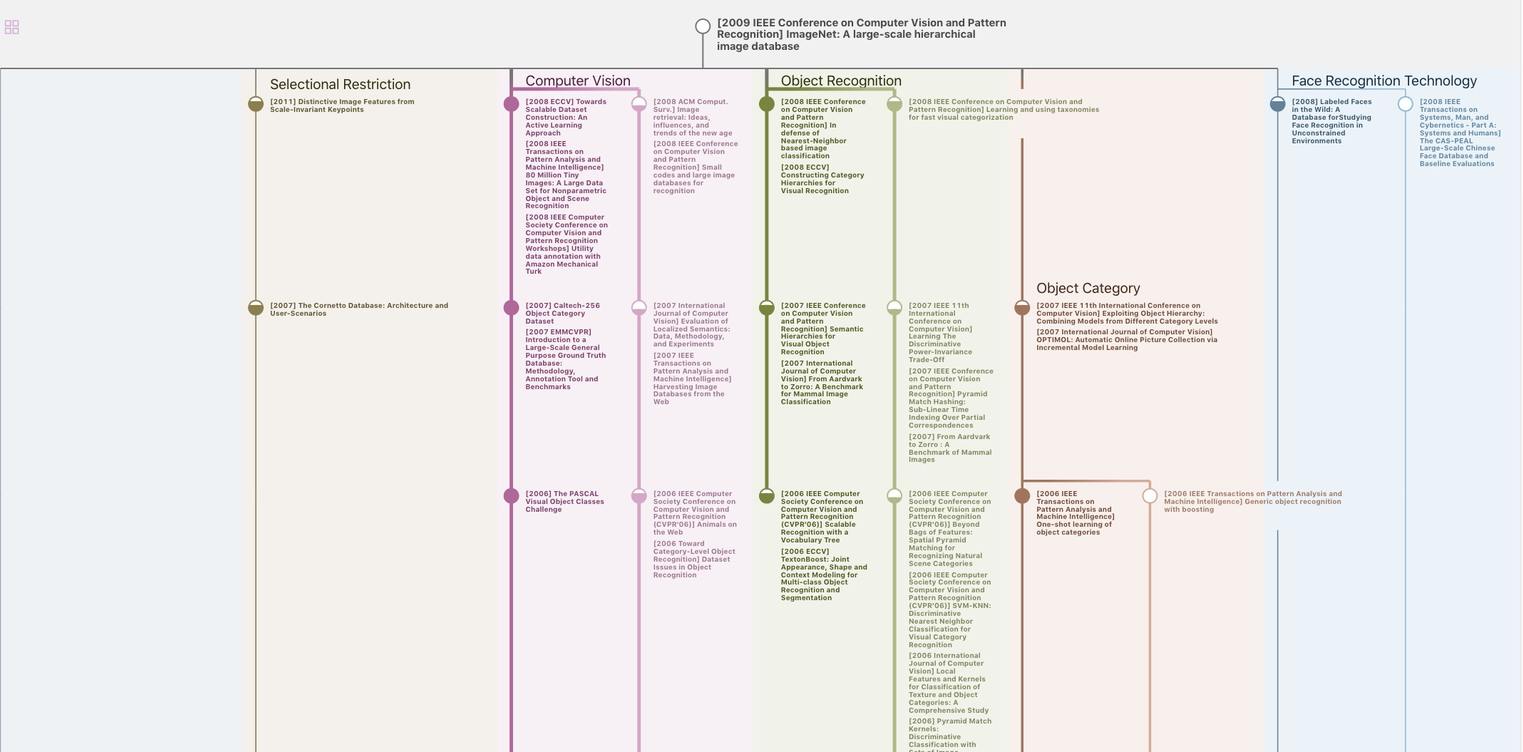
Generate MRT to find the research sequence of this paper
Chat Paper
Summary is being generated by the instructions you defined