A divergence-based condition to ensure quantile improvement in black-box global optimization
arxiv(2024)
摘要
Black-box global optimization aims at seeking for the minimizers of an
objective function whose analytical form is not known. To do so, many
state-of-the-art methods rely on sampling-based strategies, where sampling
distributions are built in an iterative fashion, so that their mass concentrate
where the objective function is low. Despite empirical success, the convergence
of these methods remains difficult to show theoretically. In this work, we
introduce a new framework, based on divergence-decrease conditions, to study
and design black-box global optimization algorithms. We show that the
information-geometric optimization approach fits within our framework, which
yields a new proof for its convergence analysis. We also establish a quantile
improvement result for two novel algorithms, one related with the cross-entropy
approach with mixture models, and another using heavy-tailed sampling
distributions.
更多查看译文
AI 理解论文
溯源树
样例
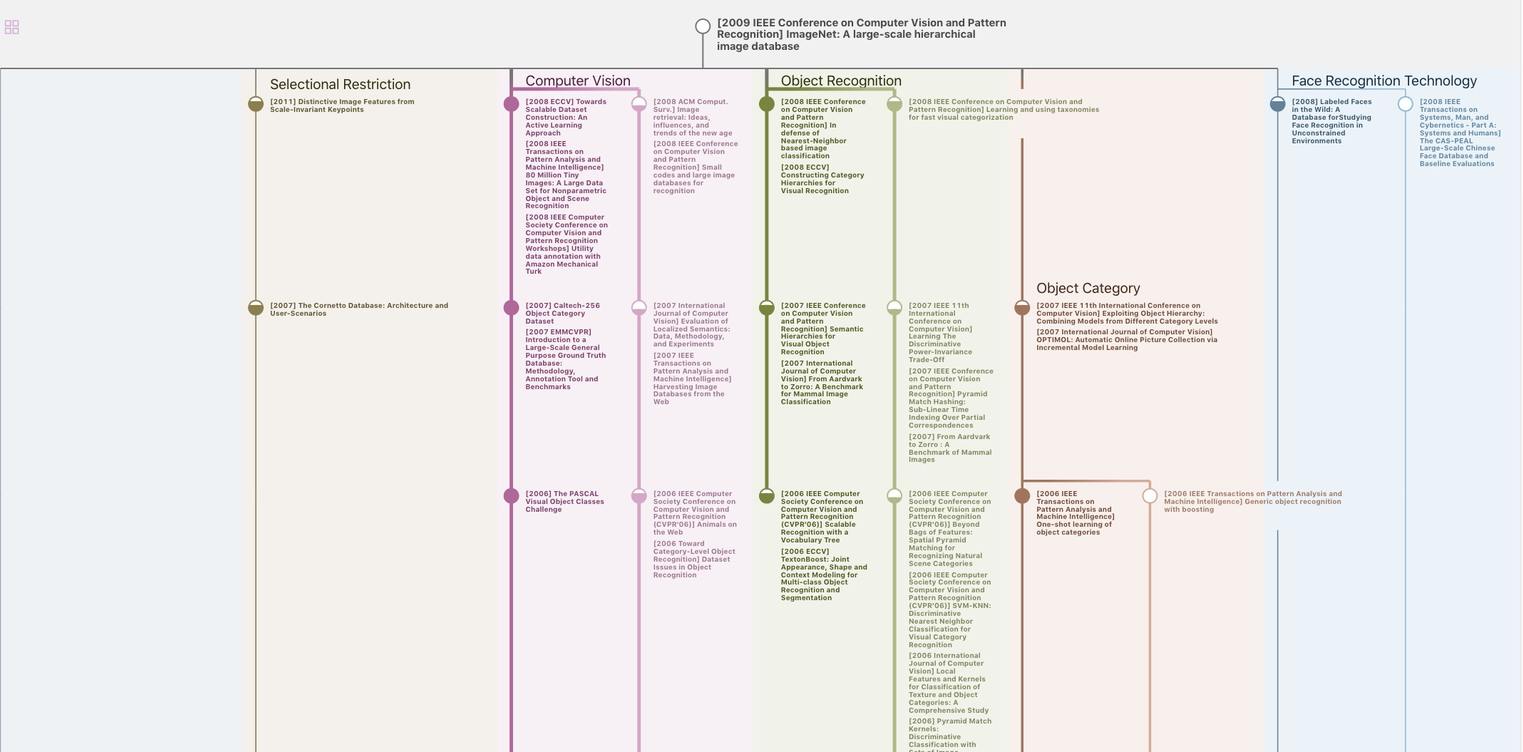
生成溯源树,研究论文发展脉络
Chat Paper
正在生成论文摘要