Advantages of transformer and its application for medical image segmentation: a survey
BioMedical Engineering OnLine(2024)
Abstract
Purpose Convolution operator-based neural networks have shown great success in medical image segmentation over the past decade. The U-shaped network with a codec structure is one of the most widely used models. Transformer, a technology used in natural language processing, can capture long-distance dependencies and has been applied in Vision Transformer to achieve state-of-the-art performance on image classification tasks. Recently, researchers have extended transformer to medical image segmentation tasks, resulting in good models. Methods This review comprises publications selected through a Web of Science search. We focused on papers published since 2018 that applied the transformer architecture to medical image segmentation. We conducted a systematic analysis of these studies and summarized the results. Results To better comprehend the benefits of convolutional neural networks and transformers, the construction of the codec and transformer modules is first explained. Second, the medical image segmentation model based on transformer is summarized. The typically used assessment markers for medical image segmentation tasks are then listed. Finally, a large number of medical segmentation datasets are described. Conclusion Even if there is a pure transformer model without any convolution operator, the sample size of medical picture segmentation still restricts the growth of the transformer, even though it can be relieved by a pretraining model. More often than not, researchers are still designing models using transformer and convolution operators.
MoreTranslated text
Key words
Deep learning,Transformer,Medical image,Segmentation,Codec
AI Read Science
Must-Reading Tree
Example
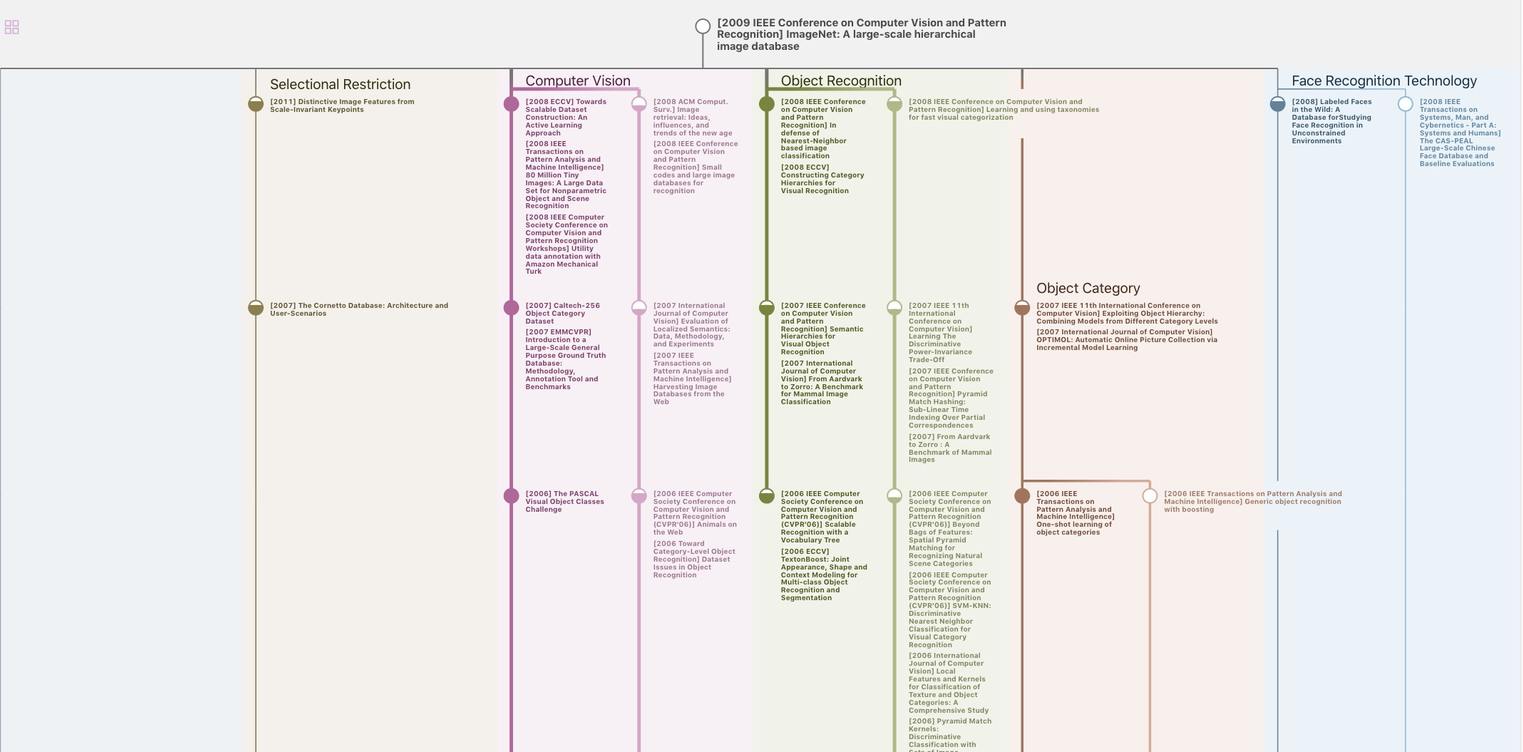
Generate MRT to find the research sequence of this paper
Chat Paper
Summary is being generated by the instructions you defined