Reinforcement Learning-based Energy Management System for Microgrids with High Renewable Energy Penetration
2023 IEEE International Conference on Energy Technologies for Future Grids (ETFG)(2023)
摘要
This paper presents a microgrid energy management system (M-EMS) integrating artificial intelligence (AI) and the Internet of Things (IoT), designed for high renewable energy penetration microgrids. The system comprises three control stages. First, the day-ahead planning considers the solar power generation forecasts, load consumption forecasts, and electric vehicle (EV) charging demand. A linear programming optimization algorithm generates optimal scheduling and power transaction recommendations. Second, the daily economic dispatch optimization occurs in five-minute time windows, including energy storage system (ESS) and EV charger scheduling, and outputs power adjustment strategies for solar smart inverters. Lastly, the real-time control utilizes an AI-based reinforcement learning (RL) model within the five-minute time window at one-minute intervals, swiftly adapting microgrid ESS and EV charging power to tackle uncertainties in renewable energy generation and load consumption. The proposed M-EMS has been tested in a commercial building microgrid. With the proposed system, power utilization efficiency improves by 4%, power savings increase by 121%, power losses reduce by 61%, power transaction aggregation surges by 135%, and peak demand reduction reaches 17%. This approach aims to address uncertainties in renewable energy generation and load electricity consumption, enhancing the overall efficiency and reliability of microgrids.
更多查看译文
关键词
artificial intelligence,reinforcement learning,internet of things,energy management system,optimal scheduling
AI 理解论文
溯源树
样例
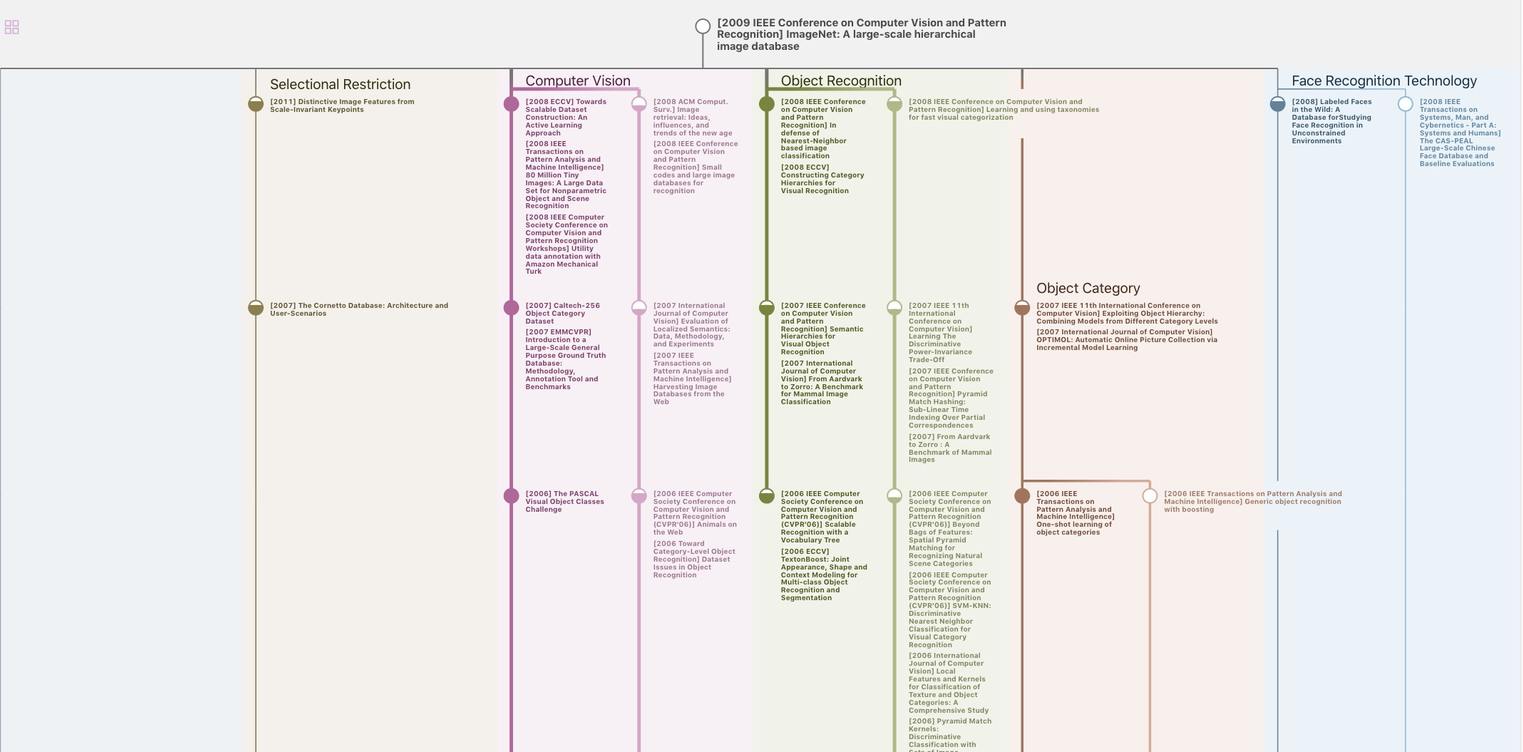
生成溯源树,研究论文发展脉络
Chat Paper
正在生成论文摘要