Robust Online Estimation of Power Flow Sensitivity Factors against Renewable Energy Stochasticity: A Sparse Representation-based Approach
2023 IEEE International Conference on Energy Technologies for Future Grids (ETFG)(2023)
摘要
Online sparse estimation of power flow sensitivity factors like injection shift factors (ISFs) plays a vital role in power system monitoring and secure operation. However, the integration of renewable energy sources makes it challenging to achieve good performance with existing sparse representation (SR) methods. It is because the pre-requisite numerical regularity conditions are guaranteed due to renewable energy stochasticity, degrading the estimation consistency. This paper proposes an Online Adaptive Lasso (Online AdaLasso) method to address this issue. It allows for the robust sparse online estimation against renewable energy stochasticity. The estimation is formulated as a sparse regression problem by using synchrophasor measurements. We leverage the AdaLasso SR method to promote the sparsity of ISF estimates and develop a new LARS-AdaLasso algorithm for online estimation. Results on the IEEE 118-bus system with wind generations show that the regularity conditions are violated with high probability due to wind power stochasticity, leading to biased estimates by existing methods. By contrast, the proposed method achieves good estimation consistency and robustness against renewable energy stochasticity. It produces unbiased estimates of the dominant ISFs and has high computational efficiency for online applications.
更多查看译文
关键词
Power system monitoring,renewable energy integration,robust estimation,sensitivity analysis,situational awareness,sparse representation
AI 理解论文
溯源树
样例
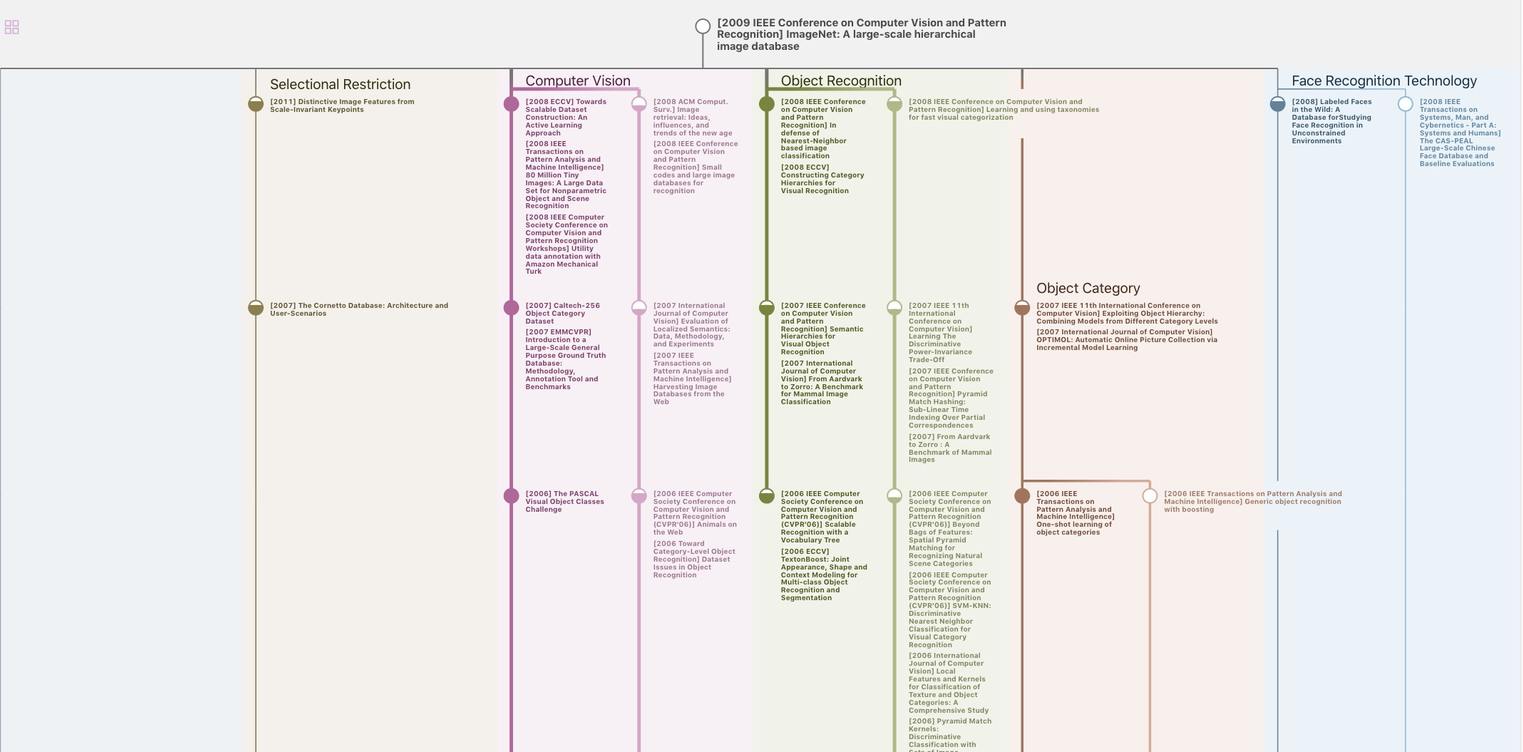
生成溯源树,研究论文发展脉络
Chat Paper
正在生成论文摘要