Multi-Turbine Wind Power Forecasting with Simplified Firework Algorithm Optimized GCN-LSTM
2023 IEEE International Conference on Energy Technologies for Future Grids (ETFG)(2023)
摘要
In this article, we present a model to enhance the wind power forecasting by incorporating both temporal and spatial capabilities. We employ the long short-term memory (LSTM) algorithm to forecast wind power based on time-series SCADA data from wind turbines. However, the LSTM algorithm introduces numerous parameters required to find the optimal value. To address this, we employ the bare bones fireworks algorithm (BBFWA) to optimize the hyperparameter tuning which offers good global search ability and is easy to implement. Furthermore, recognizing the importance of capturing the spatial correlation among turbines within a wind farm, the graph convolutional networks (GCN) is applied to extract spatial features that would affect performance from multiple turbines. The proposed GCN-BBFWA-LSTM model aims at increasing the accuracy of the prediction with simplified hyperparameter tuning and facilitating an investigation into the spatial correlation between turbines in the wind farm.
更多查看译文
关键词
BBFWA,LSTM,Wind Power,GCN-BBFWA-LSTM
AI 理解论文
溯源树
样例
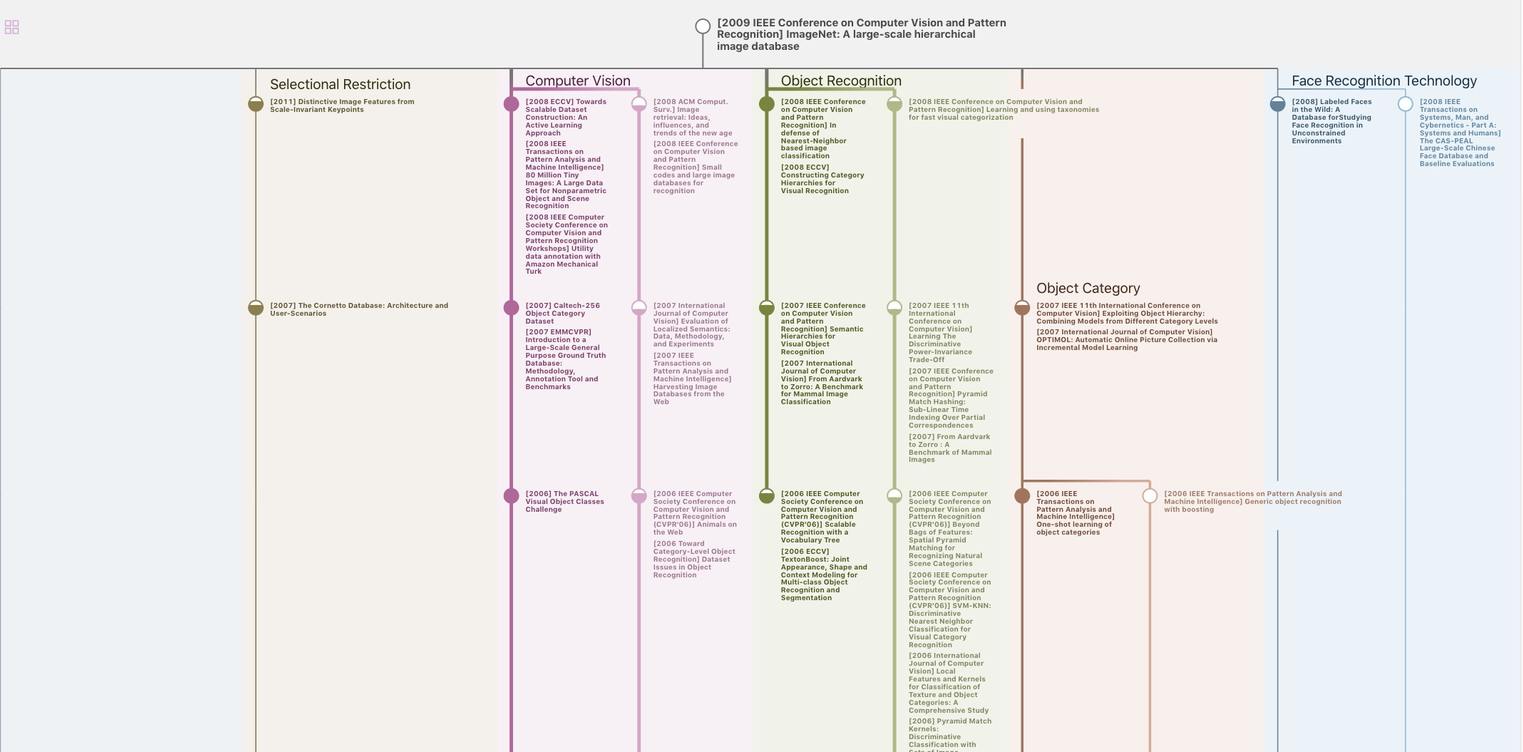
生成溯源树,研究论文发展脉络
Chat Paper
正在生成论文摘要