An Electrical Load Forecasting Method Using An Improved Online Training Strategy
2023 IEEE International Conference on Energy Technologies for Future Grids (ETFG)(2023)
摘要
This work proposes a one-step-ahead electrical load forecasting strategy based on machine learning. The approach relies on a novel data-efficient online training data selection mechanism that uses an improved dynamic time warping method to dynamically subselect the most relevant training data from the entire dataset. It also uses an adaptive prediction result optimization method to further enhance forecasting accuracy. By continuously assessing the accuracy of prior forecasts, the proposed optimization method adjusts the real-time prediction outputs and the prediction framework to best reflect current consumption conditions. The effectiveness and robustness of the proposed strategy are assessed by two real-world datasets: a university campus demand dataset and an open-source dataset provided by Open Power System. Its performance is compared with nine alternative forecasting methods using six different evaluation metrics. Our experimental outputs show that the proposed forecasting approach consistently outperforms the alternative methods, yielding the most accurate forecasts across all metrics while using the smallest training data size. The proposed forecasting strategy, therefore, shows great potential to enhance the capabilities of ML-based prediction methods in smart grids.
更多查看译文
关键词
Machine learning,electrical load forecasting,online training,data efficiency,smart grid
AI 理解论文
溯源树
样例
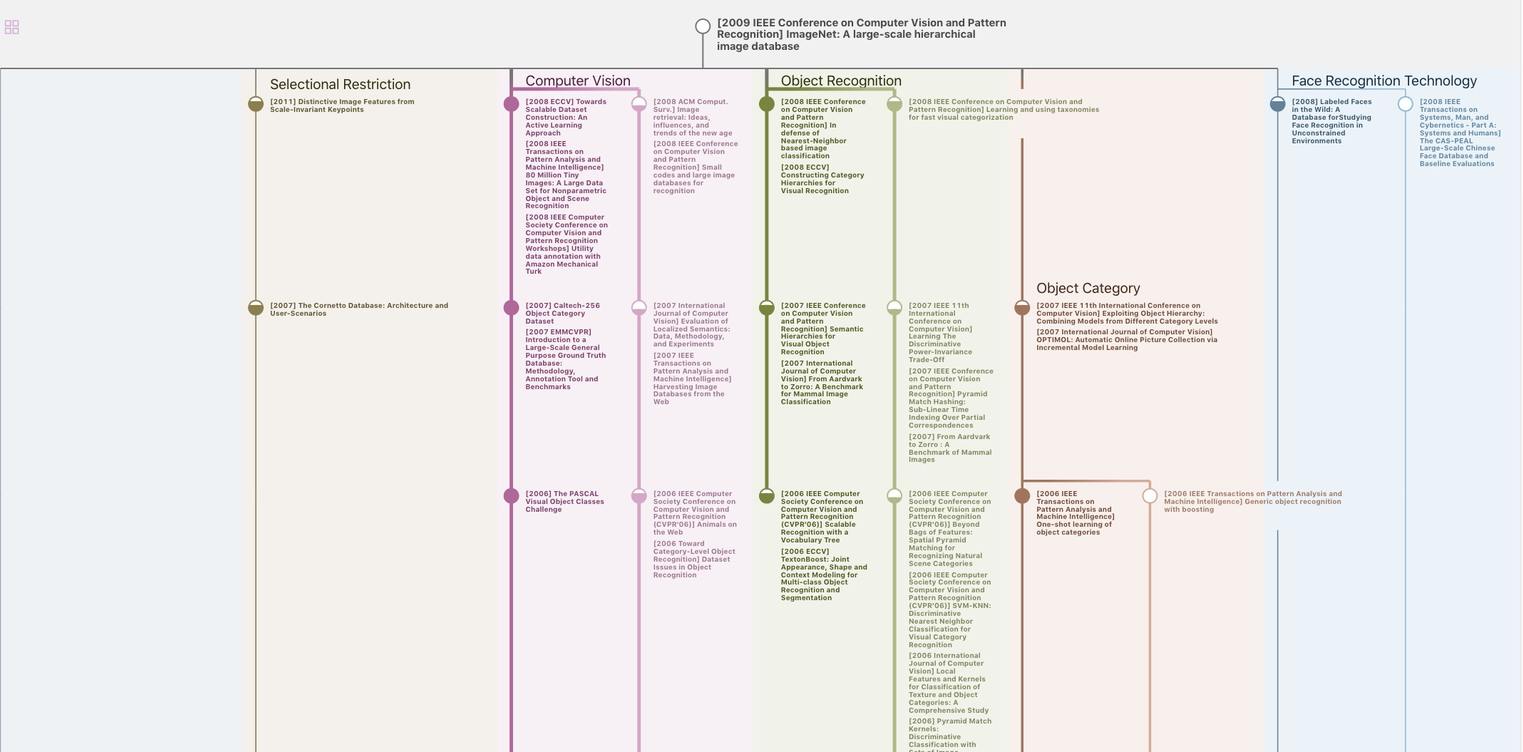
生成溯源树,研究论文发展脉络
Chat Paper
正在生成论文摘要