Heterogeneous transfer learning: recent developments, applications, and challenges
Multimedia Tools and Applications(2024)
摘要
Transfer learning (TL) has emerged as a promising area of research in machine learning (ML) due to its ability to enhance learning efficiency and accuracy by leveraging knowledge from related domains. However, traditional TL is limited in its applicability to real-world scenarios where the assumption of identical feature spaces and distributions between source and target domains is untenable. To address this limitation, Heterogeneous Transfer Learning (HeTL) has emerged as an important research direction that enables knowledge transfer between domains with heterogeneous feature spaces and distributions. Motivated by the growing interest and significance of HeTL, this survey paper comprehensively reviews recent HeTL developments, beginning with mathematical TL definitions and a taxonomy of TL categories. It delves into HeTL, explaining its classification and research status, and highlights symmetric and asymmetric HeTL advancements. Next, we explored the applications of HeTL in various disciplines, such as image and text classification, activity recognition, and cross-project defect prediction, emphasizing HeTL’s advantages over Traditional TL. Furthermore, we also discuss the challenges in HeTL, such as heterogeneity, transferability, negative learning, interpretability, and explainability. Finally, we conclude with a discussion on HeTL directions for future research.
更多查看译文
关键词
Machine learning,Transfer learning,Heterogeneous transfer learning,Feature spaces,Knowledge transfer
AI 理解论文
溯源树
样例
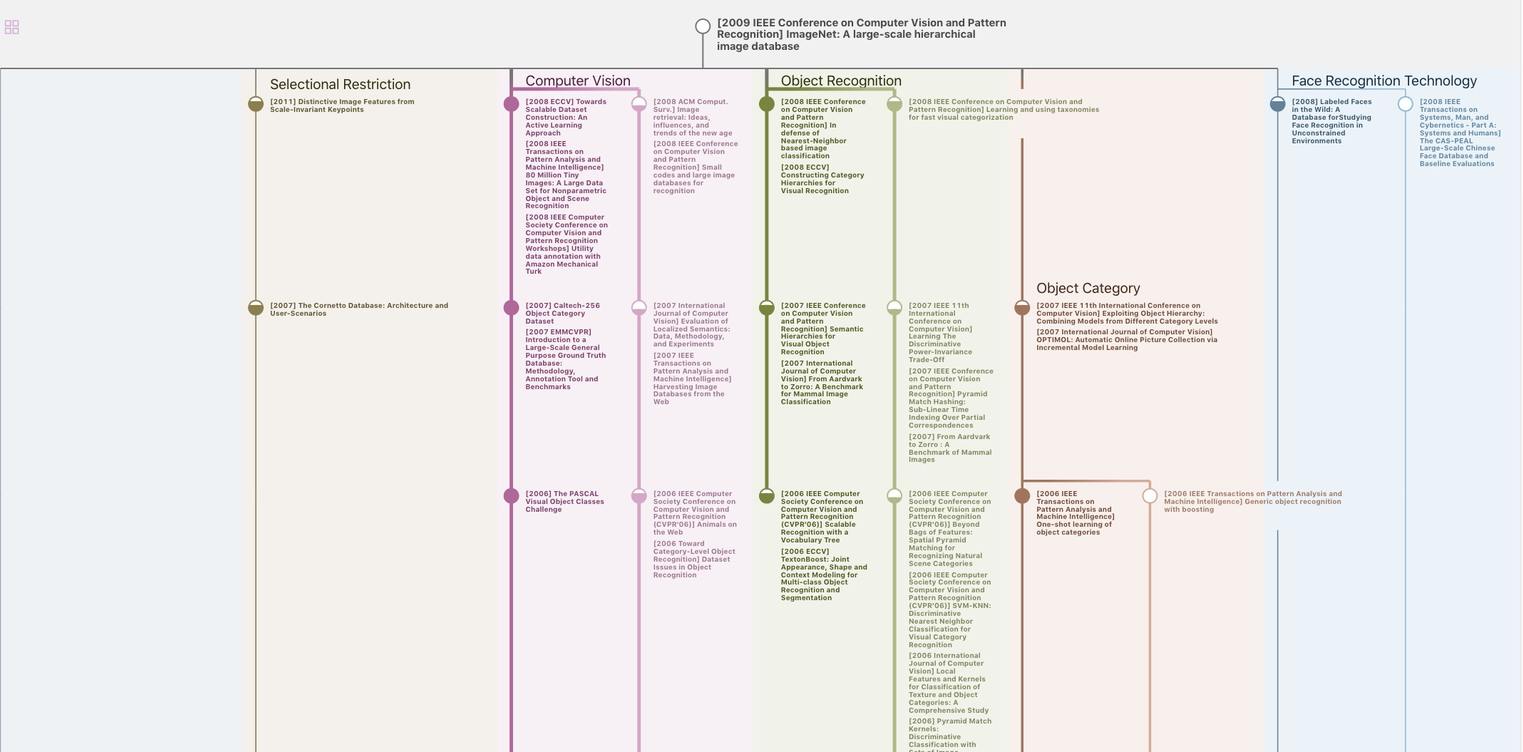
生成溯源树,研究论文发展脉络
Chat Paper
正在生成论文摘要