TopEC: Improved classification of enzyme function by a localized 3D protein descriptor and 3D Graph Neural Networks
biorxiv(2024)
摘要
Accurately annotating molecular function to enzymes remains challenging. Computational methods can aid in this and allow for high-throughput annotation. Tools available for inferring enzyme function from general sequence, fold, or evolutionary information are generally successful. However, they can lead to misclassification if for certain sequences a deviation in local structural features influences the function. Here, we present TopEC, a 3D graph neural network based on a localized 3D descriptor to learn chemical reactions of enzymes from (predicted) enzyme structures and predict Enzyme Commission (EC) classes. Using the message passing frameworks from SchNet and DimeNet++, we include distance and angle information to improve the predictive performance compared to regular 2D graph neural networks. We obtained significantly improved EC classification prediction (F-score: 0.72) without fold bias at residue and atomic resolutions and trained networks that can classify both experimental and computationally generated enzyme structures for a vast functional space (> 800 ECs). Our model is robust to uncertainties in binding site locations and similar functions in distinct binding sites. By investigating the importance of each graph node to the predictive performance, we see that TopEC networks learn from an interplay between biochemical features and local shape-dependent features.
### Competing Interest Statement
The authors have declared no competing interest.
更多查看译文
AI 理解论文
溯源树
样例
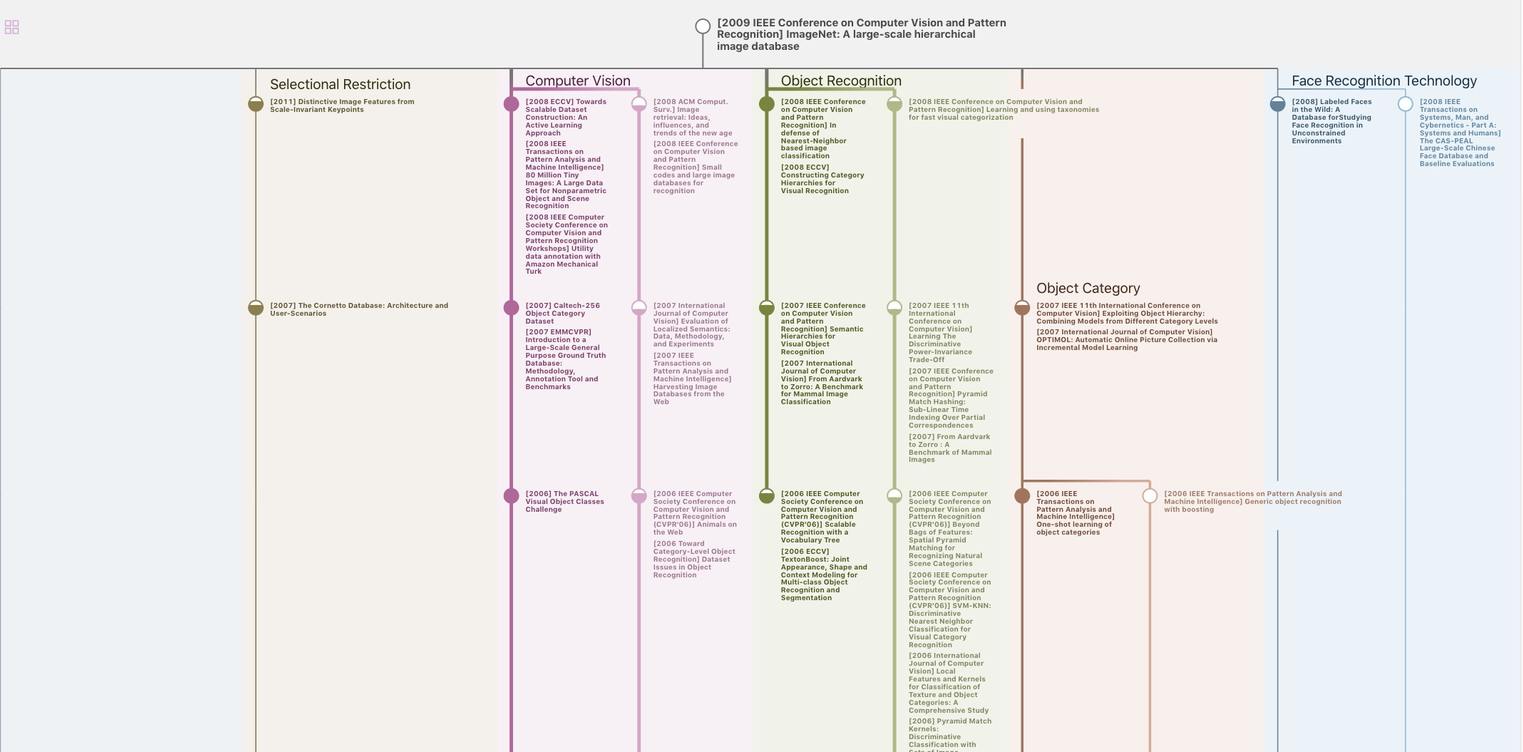
生成溯源树,研究论文发展脉络
Chat Paper
正在生成论文摘要