Counter-Intuitive Effects of Q-Learning Exploration in a Congestion Dilemma
IEEE ACCESS(2024)
摘要
Exploration is an integral part of learning dynamics which allows algorithms to search a space of solutions. When many algorithms simultaneously explore, this can lead to counter-intuitive effects. This paper contributes an analysis of the influence that exploration has on a multi-agent system of Q-learners in a famous congestion dilemma, the Braess paradox. I find ranges of the exploration rate for which epsilon-greedy Q-learners show chaotic and oscillatory dynamics which do not converge, and yield better than Nash equilibrium results. I decouple the dynamics endogenous to Q-learning from the exogenous exploration rate epsilon, and find that Q-learners implicitly coordinate with low exploration rates epsilon is an element of (0, 0.1), but are disrupted in their coordination for larger exploration rates epsilon > 0.1. The best implicit coordination leads to a 20% reduction in average travel times which approaches the social optimum. I discuss how our results may inform multi-agent algorithm design, fit within a cognitive science perspective of cognitive noise during learning, and provide a mechanistic hypothesis for the lack of empirical evidence of the Braess Paradox in traffic systems.
更多查看译文
关键词
Braess paradox,chaos,congestion games,learning dynamics,reinforcement learning,Q-learning
AI 理解论文
溯源树
样例
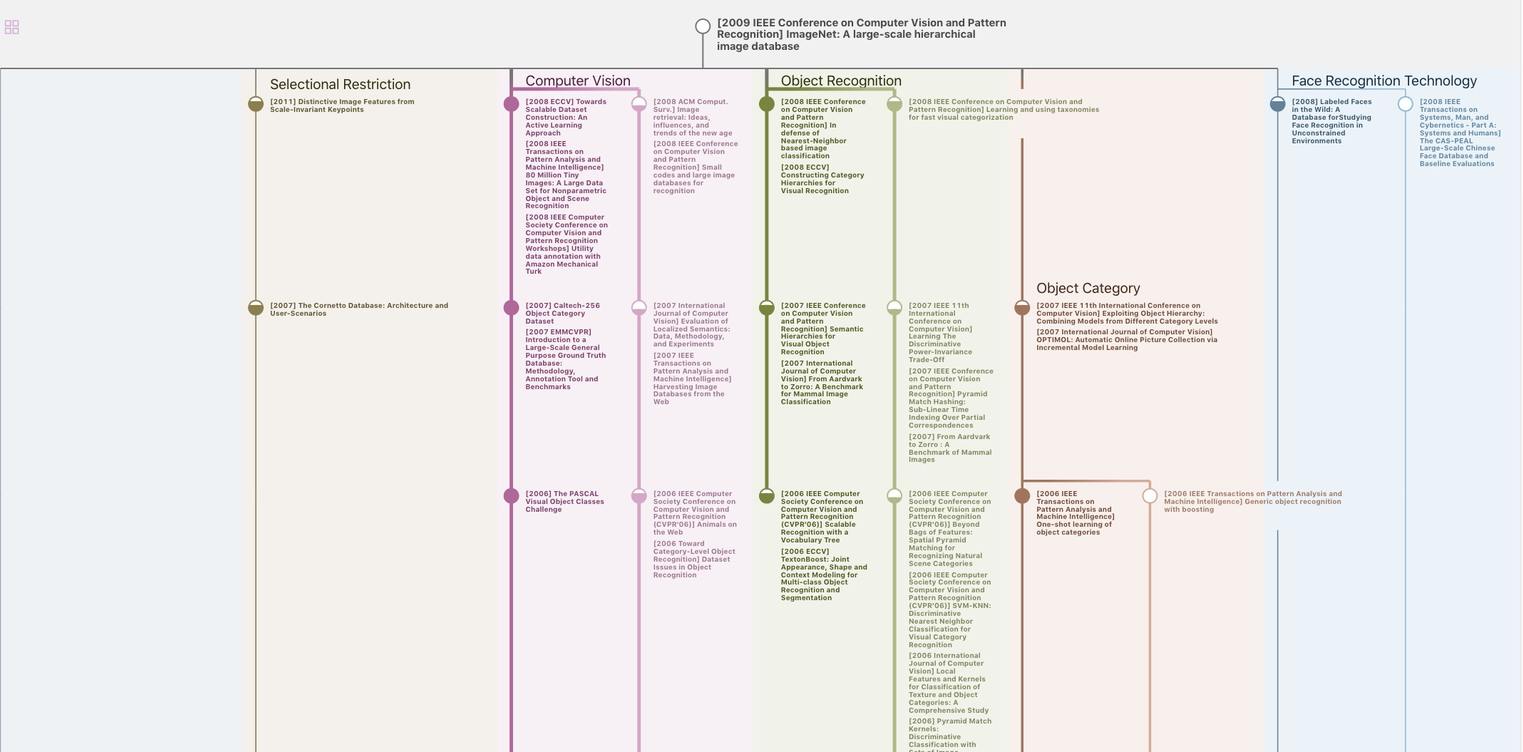
生成溯源树,研究论文发展脉络
Chat Paper
正在生成论文摘要