Electromyography Gestures Sensing with Deeply Quantized Neural Networks.
MetroXRAINE(2023)
摘要
Electromyography measures muscle electrical activity as the response to a nerve's stimulation. Recent developments in deep learning have also been applied to process bio-signals. The development of Deeply Quantized Neural Networks offers the opportunity to design low complex machine learning inferences. They target very low bit-depth representations for weights, biases, and activations. However, they could be highly affected by decreased performances if pushed to one-bit representation. This paper investigated hand gesture classification using electromyography signals. To accomplish this task at the lowest bit depth to save memory and complexity assets, was challenging from the accuracy perspective. One objective was to build a highly accurate model to classify the hand gestures while fulfilling the limited memory and computation latency constraints of a low-cost microcontroller, according to the model complexity. The classification accuracy achieved was 93.94% on the Italian Sign Language dataset and 83.70% on the Gestures Master dataset. The models were characterized on two STM32 microcontrollers in terms of execution time. On STM32H7, the 8-bits model ran around 8.306ms/inference. It was 11 ms faster than a fp32 based convolutional neural network. On STM32G4 instead, the 8-bits model was executed around 40 ms/inference while 102 ms for the fp32 model. Furthermore, these models have been profiled by ISPU.AI, an experimental tool to deploy pre-trained neural networks into super integrated inertial sensor processing unit, requiring less hardware resources than an off-the-shelf microcontroller. On the Italian Sign Language dataset and the Gestures Master dataset the maximum RAM was 29.17 KiB while the FLASH 21.13KiB with a computational complexity of 1,451,413 multiply and accumulates at 10 MHz
更多查看译文
关键词
Hand gesture classification,electromyography,deep quantization,microcontrollers,intelligent signal processing unit
AI 理解论文
溯源树
样例
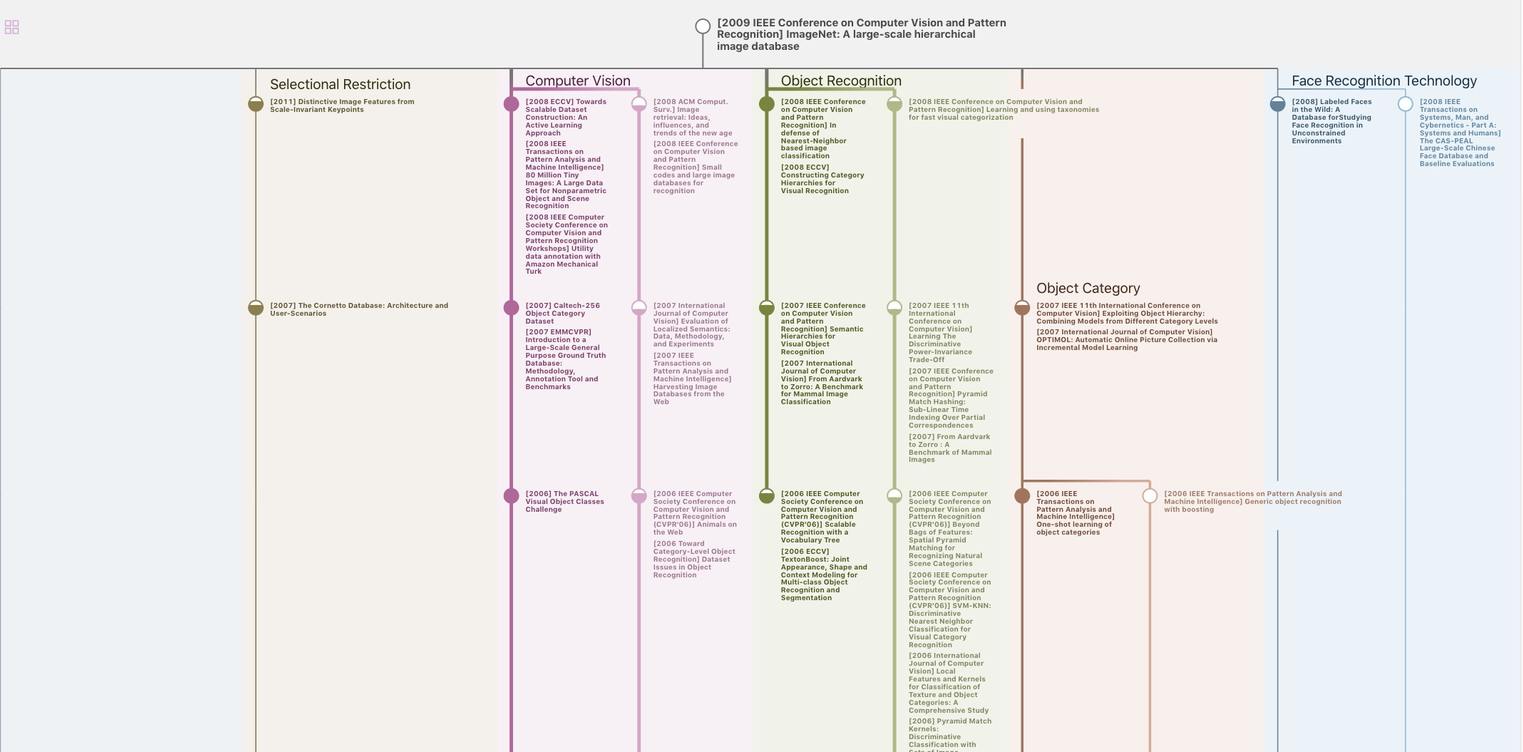
生成溯源树,研究论文发展脉络
Chat Paper
正在生成论文摘要