TinyRCE: Forward Learning Under Tiny Constraints.
MetroXRAINE(2023)
摘要
The challenge posed by on-tiny-devices learning targeting ultra-low power devices has recently attracted several machine learning researchers. A typical on-device model learning session processes real time streams of data produced by heterogeneous sensors. In such a context, this paper proposes TinyRCE, a forward-only learning approach based on a hyperspherical classifier aiming to be deployed on microcontrollers and, potentially, on sensors. The learning process is fed by labeled data streams to be classified by the proposed method. The classical RCE algorithm has been modified by adding a forget mechanism to discard useless neurons from the classifier's hidden layer, since they could become redundant over time. TinyRCE is fed with compact features extracted by a convolutional neural network which could be an extreme learning machine. In such case, the weights of the topology were randomly initialized instead of trained offline with backpropagation. Its weights are stored in a tiny read-only memory of 76.45KiB. The classifier required up to 40.26KiB of RAM to perform a complete on-device learning workload in 0.216s, running on an MCU clocked at 480MHz. TinyRCE has been evaluated with a new interleaved learning and testing protocol to mimic an on-tiny-device forward learning workload. It has been tested with openly available datasets representing human activity monitoring (PAMAP2, SHL) and ball-bearing anomaly detection (CWRU) case studies. Experiments have shown that TinyRCE performed competitively against a supervised convolutional topology followed by a SoftMax classifier trained with backpropagation on all these datasets.
更多查看译文
关键词
On-Tiny-Device Learning,TinyML,Hyper-Spherical Classifier,Extreme Learning Machines,Feature Extraction,Human Activity Recognition
AI 理解论文
溯源树
样例
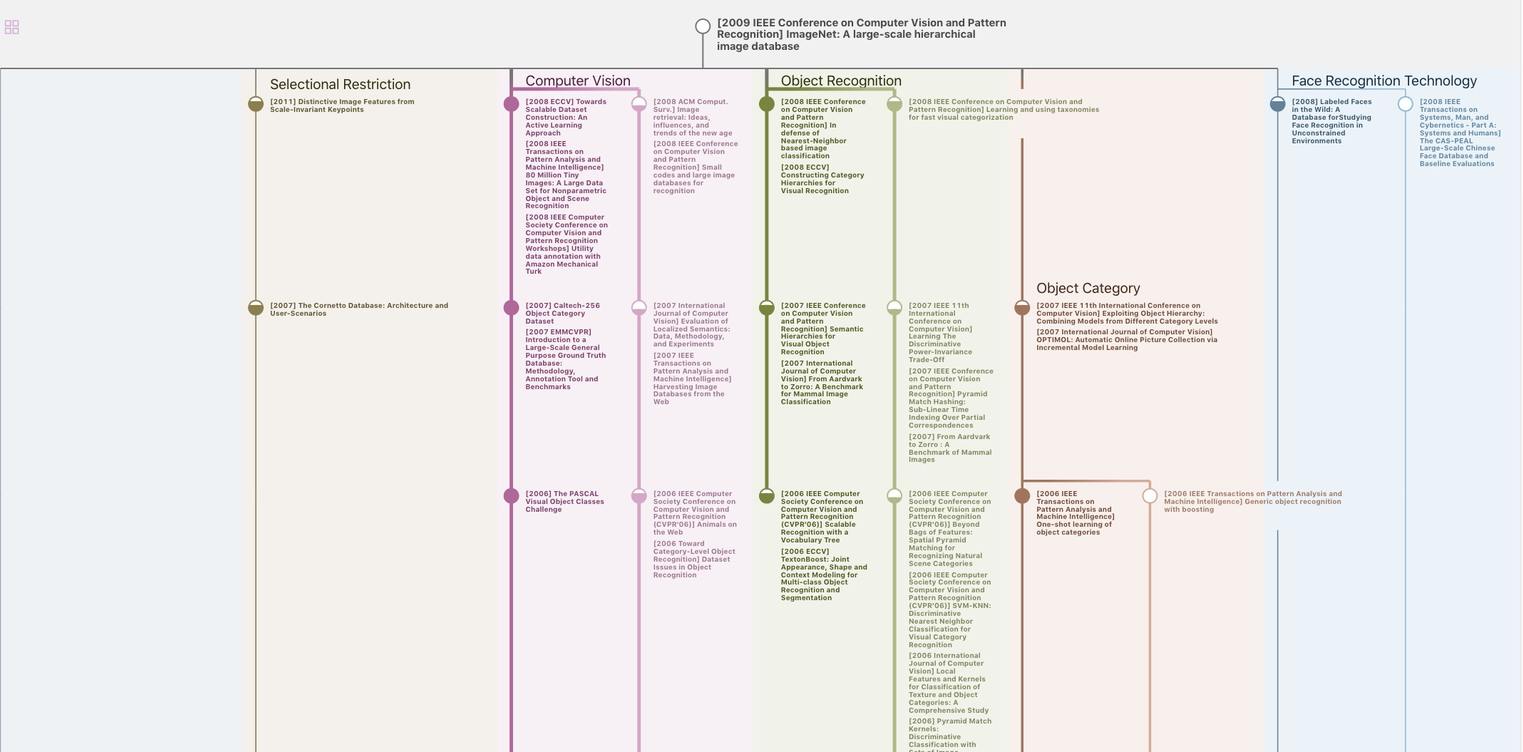
生成溯源树,研究论文发展脉络
Chat Paper
正在生成论文摘要