Merging Contour-and Region-Aware Branches in the UNet Decoder to Enhance Osseous Segmentation in Shoulder CT Scans.
MetroXRAINE(2023)
摘要
Medical image segmentation plays a crucial role in modern healthcare systems, and the use of artificial intelligence tools, particularly deep convolutional neural networks, has significantly advanced the field. However, achieving accurate voxel resolution segmentation and 3D reconstruction remains challenging due to inherent image noise, complex anatomical structures, and overlapping regions. This study explores the application of a novel Unet variant, CEL-Unet, to boost humerus and scapula segmentation in osteoarthritic shoulder joints. The performance of CEL-Unet is compared to standard Unet models trained alternatively with two different loss functions: Dice Loss and Focal Loss. The results demonstrate high recall and precision rates for the predicted masks, with CEL-Unet statistically outperforming the other models in terms of recall. Additionally, 3D surface evaluation shows low Root Mean Square Errors and Hausdorff distances, with CEL-Unet achieving the lowest mean and maximum distance compared to the other networks. The combination of Personalized Surgery Instrumentation (PSI) and automatic CT segmentation offers improved surgical accuracy and shorter operating times. Although manual refinement is necessary for optimal results, the semi-automatic approach significantly reduces processing time while ensuring accurate segmentation. This collaborative approach enhances the speed and accuracy of the segmentation process, making it valuable in personalized surgery instrumentation, medical research, and clinical practice.
更多查看译文
关键词
Automatic segmentation,Computed Tomography,Convolutional Neural Networks,Personalized Surgery Instrumentation
AI 理解论文
溯源树
样例
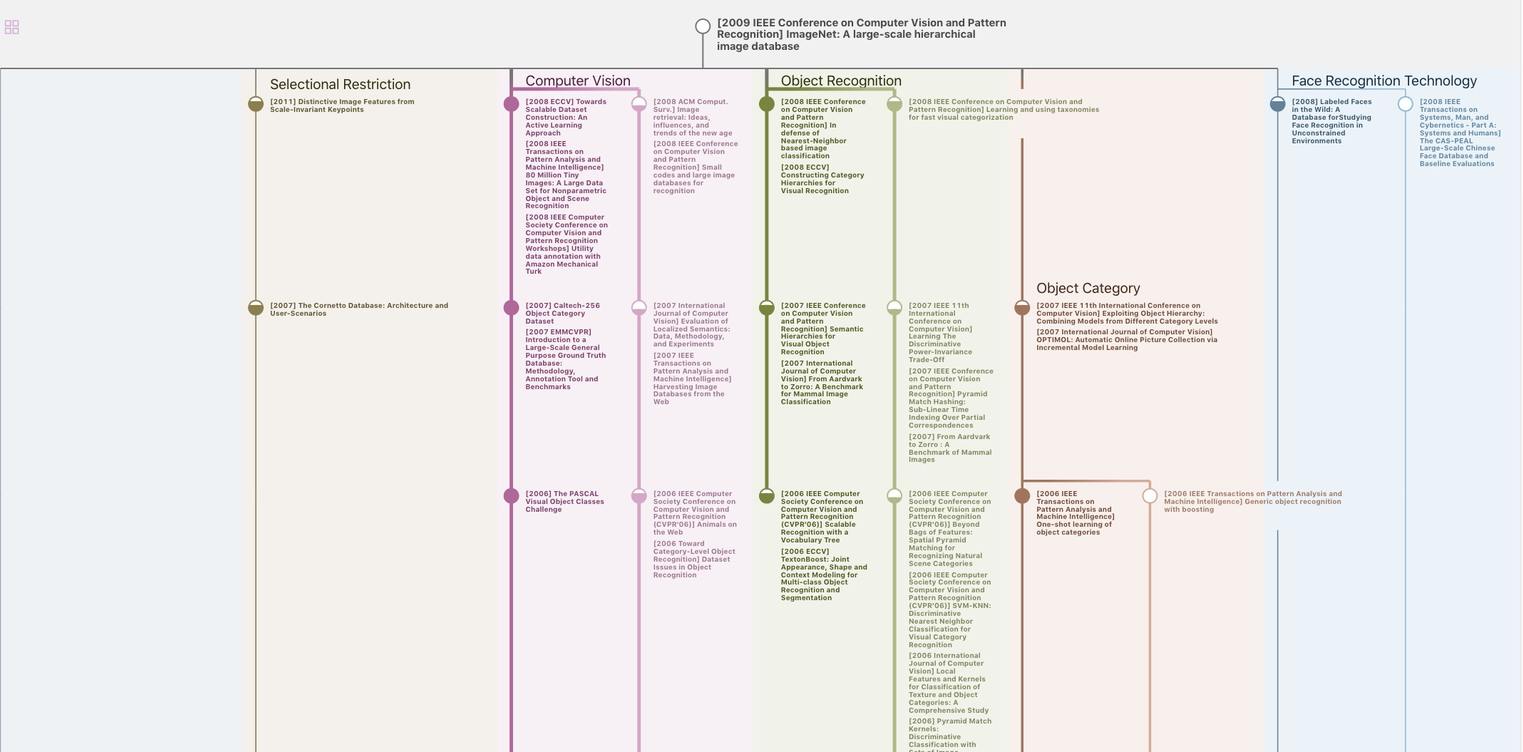
生成溯源树,研究论文发展脉络
Chat Paper
正在生成论文摘要